Driver Digital Twin for Online Recognition of Distracted Driving Behaviors.
IEEE Trans. Intell. Veh.(2024)
摘要
Deep learning has been widely utilized in intelligent vehicle systems, particularly in the field of driver distraction detection. However, existing methods in this application tend to focus solely on appearance or cognitive state as indicators of distraction, while neglecting the significance of temporal modeling in accurately identifying driver actions. This oversight can result in limitations such as difficulty in comprehending context, incapability to recognize gradual changes, and failure to capture complex behaviors. To address these limitations, this paper introduces a new framework based on the concept of Driver Digital Twin (DDT). The DDT framework serves as a digital replica of the driver, capturing their naturalistic driving data and behavioral models. It consists of a transformer-based driver action recognition module and a novel temporal localization module to detect distracted behaviors. Additionally, we propose a pseudo-labeled multi-task learning algorithm that includes driver emotion recognition as supplementary information for recognizing distractions. We have validated the effectiveness of our approach using three publicly available driver distraction detection benchmarks: SFDDD, AUCDD, and SynDD2. The results demonstrate that our framework achieves state-of-theart performance in both driver action recognition and temporal localization tasks. It outperforms the leading methods by 6.5 and 0.9 percentage points on SFDDD and AUCDD, respectively. Furthermore, it ranks in the top 5% on the SynDD2 leaderboard
更多查看译文
关键词
Digital twin,driver behavior modeling,automated vehicle,driver distraction detection,temporal action localization
AI 理解论文
溯源树
样例
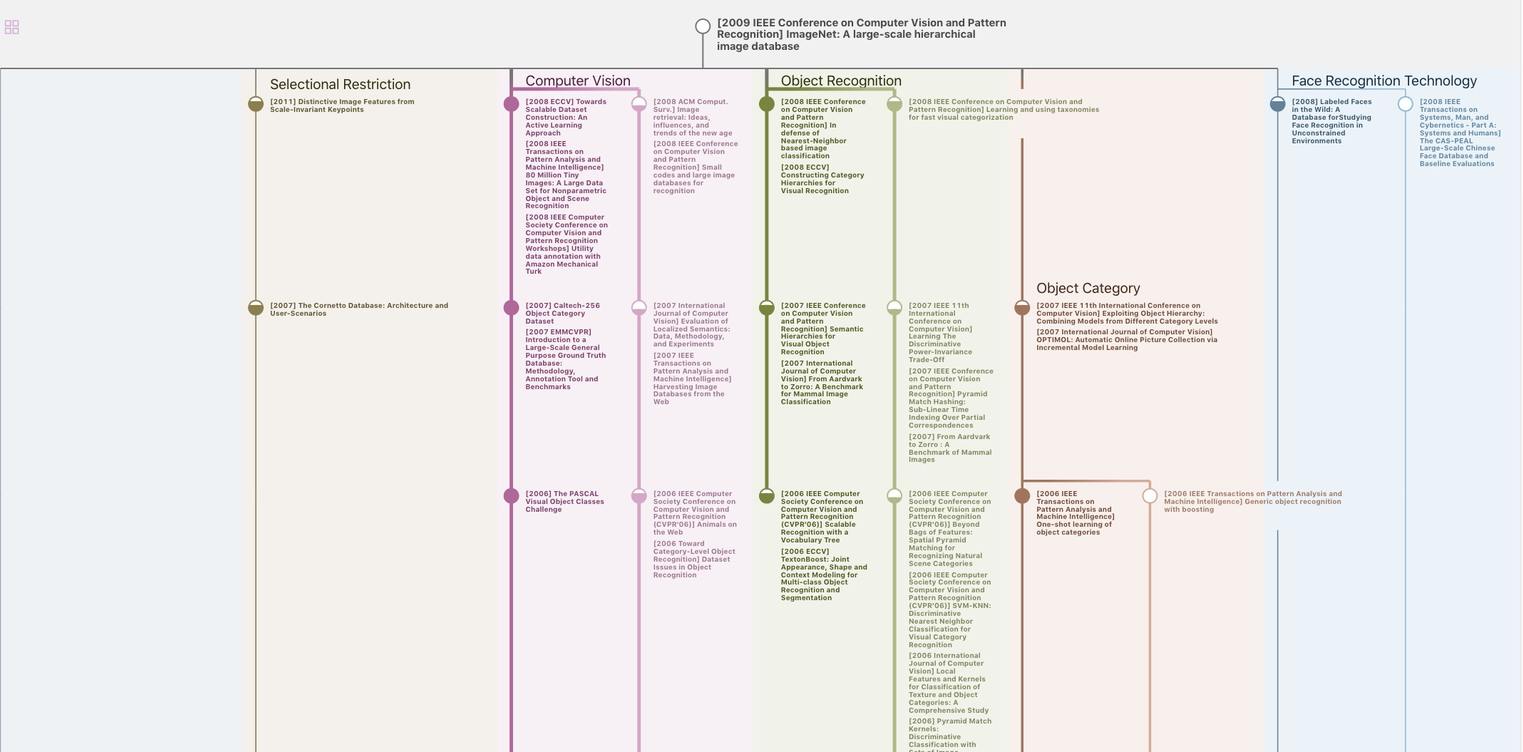
生成溯源树,研究论文发展脉络
Chat Paper
正在生成论文摘要