Approaching the Information-Theoretic Limit of Privacy Disclosure With Utility Guarantees.
IEEE Trans. Inf. Forensics Secur.(2024)
摘要
The possibility for public attributes to disclose private information has caused widespread concern. Traditional privacy-preserving approaches have two limitations: (1) Approaches based on data anonymization or distortion often lead to poor utility-privacy trade-offs, and (2) approaches based on data encryption face heavy computational costs. These problems have prompted calls for an effective privacy-preserving framework that provides adequate privacy guarantees while maintaining good data utility. Inspired by denoising autoencoders, in this paper, we regard the information about privacy attributes contained in the public attributes as a kind of noise and design an ex ante privacy-preserving model called the Mutual Information Autoencoder (MIAE), which reconstructs the loss function of the original autoencoder by combining reconstruction errors and mutual information, and we introduce a trade-off coefficient to achieve utility-privacy trade-offs. To elucidate the superiority of the proposed model, we consider utility-privacy trade-offs with the expected distortion function as a metric of data utility and the joint mutual information as a metric of privacy disclosure, and then, we construct a convex optimization problem with multiple constraints based on rate-distortion theory. From an information theory perspective, we provide a lower bound for privacy disclosure with utility guarantees. Elaborate experiments over a real-world dataset reveal that as the level of expected distortion increases, the achievable bound obtained by MIAE exhibits a trend similar to that of the information-theoretic bound. When the expected distortion surpasses 2.2, the achievable bound obtained by MIAE also converges to 0, and the maximum gap between the achievable bound obtained by MIAE and the information-theoretic bound is no more than 1.4. Compared to existing models, MIAE can provide a tighter achievable bound and achieve good utility-privacy trade-offs.
更多查看译文
关键词
Privacy inference,data distortion,privacy preservation,rate-distortion theory,utility-privacy trade-offs
AI 理解论文
溯源树
样例
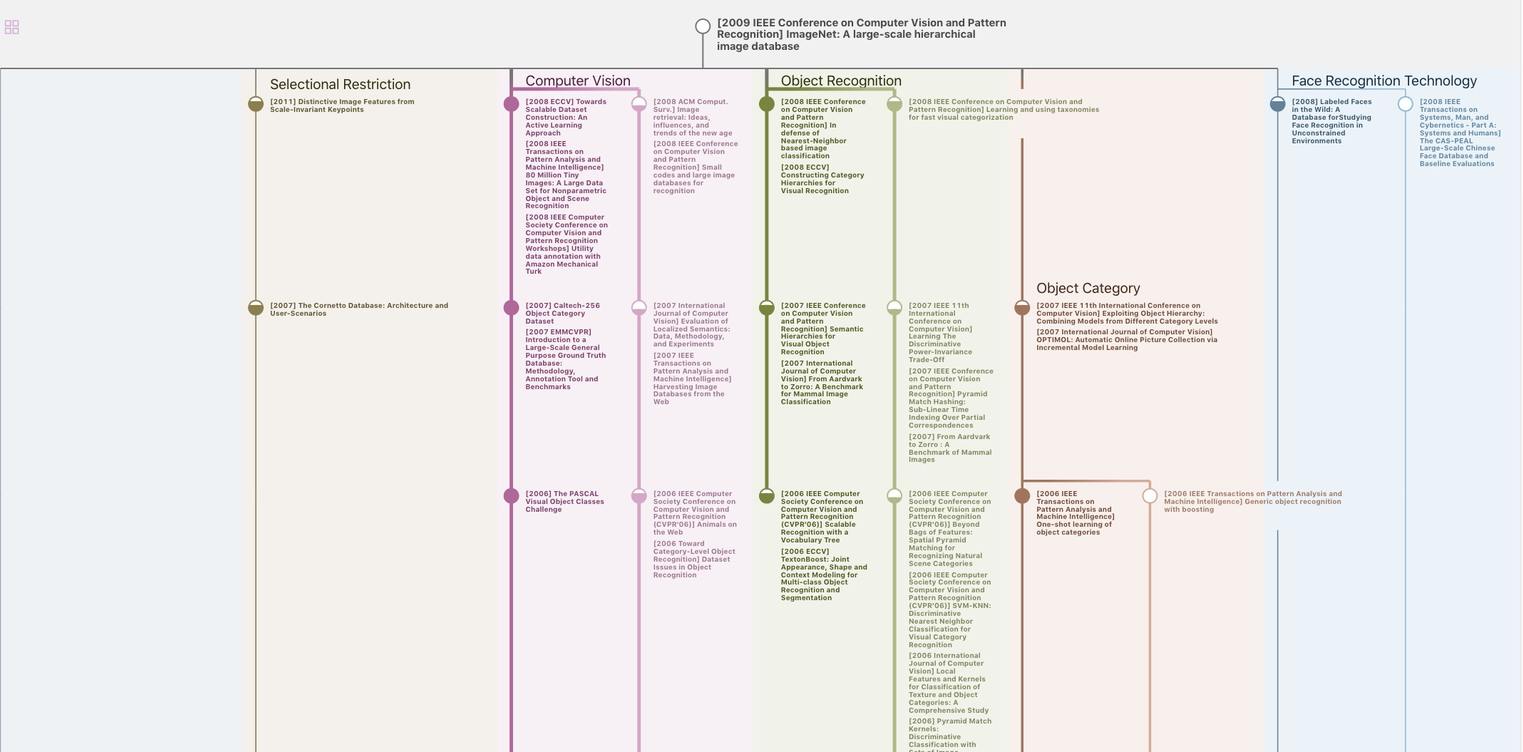
生成溯源树,研究论文发展脉络
Chat Paper
正在生成论文摘要