Exploring The Predictive Capabilities of AlphaFold Using Adversarial Protein Sequences
IEEE Transactions on Artificial Intelligence(2024)
摘要
Protein folding neural networks (PFNNs) such as AlphaFold predict remarkably accurate structures of proteins compared to other approaches. However, the robustness of such networks has heretofore not been fully explored. This is particularly relevant given the broad social implications of such technologies and the fact that biologically small perturbations to non-critical residues of a protein sequence do not typically lead to drastic changes in the protein structure. Our study demonstrates that, similar to adversarial methods in machine learning, small changes to protein sequences can result in significant differences in the predicted protein structures using AlphaFold as determined by large distance measures. Despite this, our findings using multiple protein sequences suggest that AlphaFold is able to accurately predict the domain structure and folding regions of a protein. To gauge structural differences, we employ two alignment-based measures (root-mean-square deviation (RMSD) and the Global Distance Test (GDT) similarity), and one alignment-free measure, which is an effective Graph-based Structure Representation (GraSR) method. We prove that the problem of minimally perturbing protein sequences is NP-complete. Based on the well-established BLOSUM62 sequence alignment scoring matrix, we generate adversarial sequences. In our experimental evaluation, we consider 111 proteins (including 29 COVID-19 sequences) in the Universal Protein resource (UniProt), a central resource for protein data. Our findings suggest that, despite the high RMSD values returned by AlphaFold, it is capable of handling the BLOSUM adversarial sequences considered in our analysis, as evidenced by the preservation of the folded regions and the GraSR results.
更多查看译文
关键词
Protein Folding Neural Networks,AlphaFold,BLOSSUM62 Distance,Adversarial Protein Sequences,Neural Networks Robustness
AI 理解论文
溯源树
样例
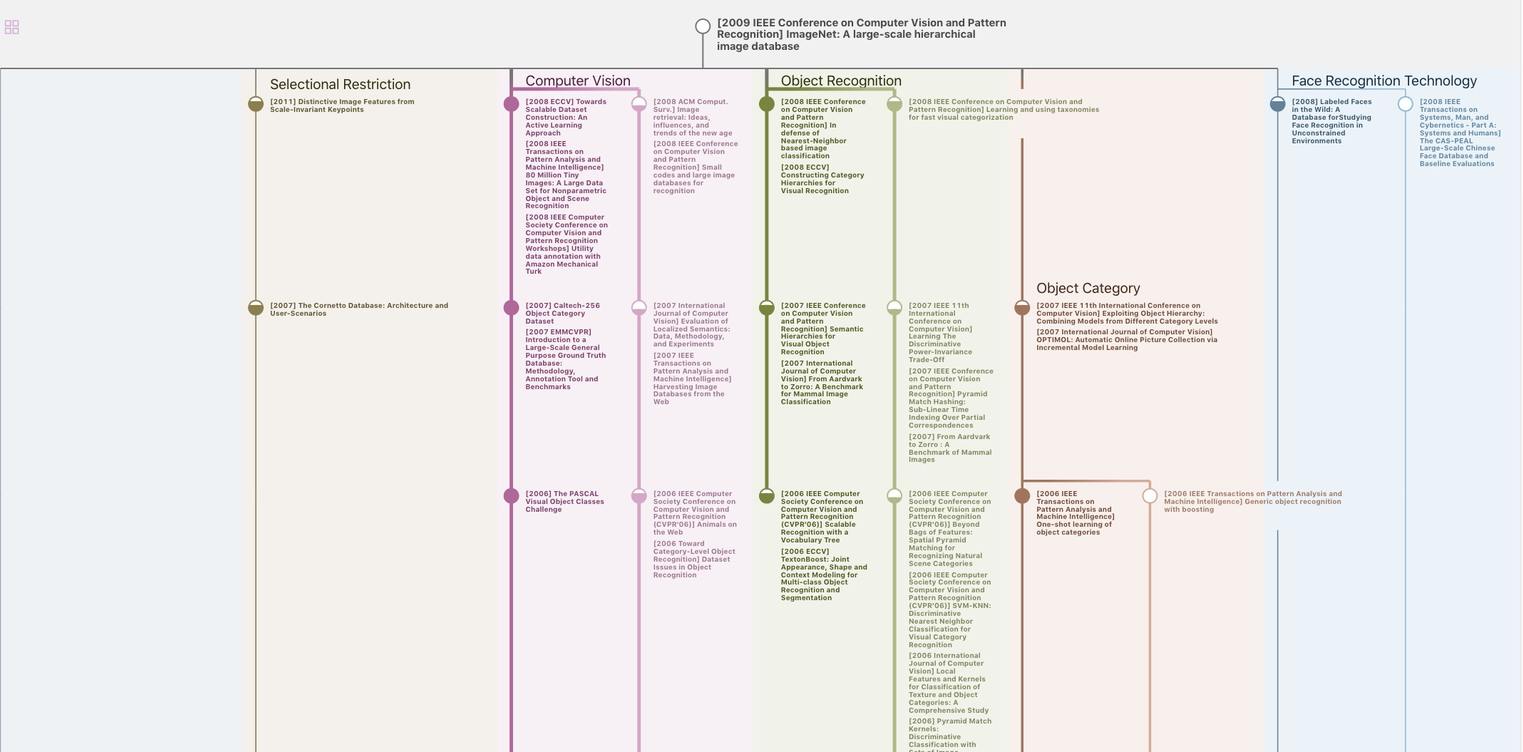
生成溯源树,研究论文发展脉络
Chat Paper
正在生成论文摘要