Triple-Refined Hybrid-Field Beam Training for mmWave Extremely Large-Scale MIMO
IEEE Transactions on Wireless Communications(2024)
摘要
This paper investigates beam training for extremely large-scale
multiple-input multiple-output systems. By considering both the near field and
far field, a triple-refined hybrid-field beam training scheme is proposed,
where high-accuracy estimates of channel parameters are obtained through three
steps of progressive beam refinement. First, the hybrid-field beam gain
(HFBG)-based first refinement method is developed. Based on the analysis of the
HFBG, the first-refinement codebook is designed and the beam training is
performed accordingly to narrow down the potential region of the channel path.
Then, the maximum likelihood (ML)-based and principle of stationary phase
(PSP)-based second refinement methods are developed. By exploiting the
measurements of the beam training, the ML is used to estimate the channel
parameters. To avoid the high computational complexity of ML, closed-form
estimates of the channel parameters are derived according to the PSP. Moreover,
the Gaussian approximation (GA)-based third refinement method is developed. The
hybrid-field neighboring search is first performed to identify the potential
region of the main lobe of the channel steering vector. Afterwards, by applying
the GA, a least-squares estimator is developed to obtain the high-accuracy
channel parameter estimation. Simulation results verify the effectiveness of
the proposed scheme.
更多查看译文
关键词
Beam training,extremely large-scale multiple-input multiple-output (XL-MIMO),Gaussian approximation,near field,principle of stationary phase
AI 理解论文
溯源树
样例
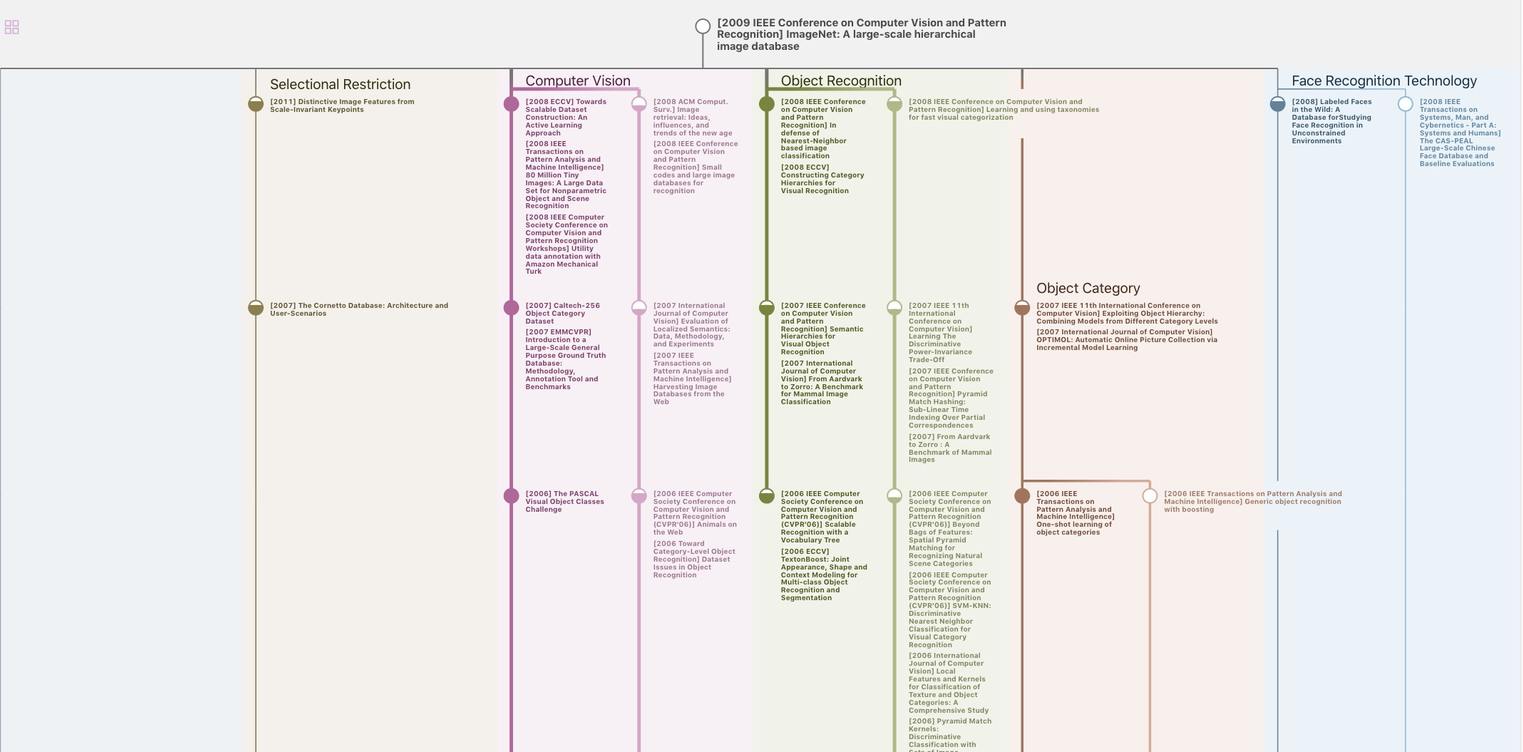
生成溯源树,研究论文发展脉络
Chat Paper
正在生成论文摘要