Two-stage sample robust optimization
arXiv (Cornell University)(2019)
摘要
We investigate a simple approximation scheme, based on overlapping linear decision rules, for solving data-driven two-stage distributionally robust optimization problems with the type-$\infty$ Wasserstein ambiguity set. Our main result establishes that this approximation scheme is asymptotically optimal for two-stage stochastic linear optimization problems; that is, under mild assumptions, the optimal cost and optimal first-stage decisions obtained by approximating the robust optimization problem converge to those of the underlying stochastic problem as the number of data points grows to infinity. These guarantees notably apply to two-stage stochastic problems that do not have relatively complete recourse, which arise frequently in applications. In this context, we show through numerical experiments that the approximation scheme is practically tractable and produces decisions which significantly outperform those obtained from state-of-the-art data-driven alternatives.
更多查看译文
关键词
optimization,sample,two-stage
AI 理解论文
溯源树
样例
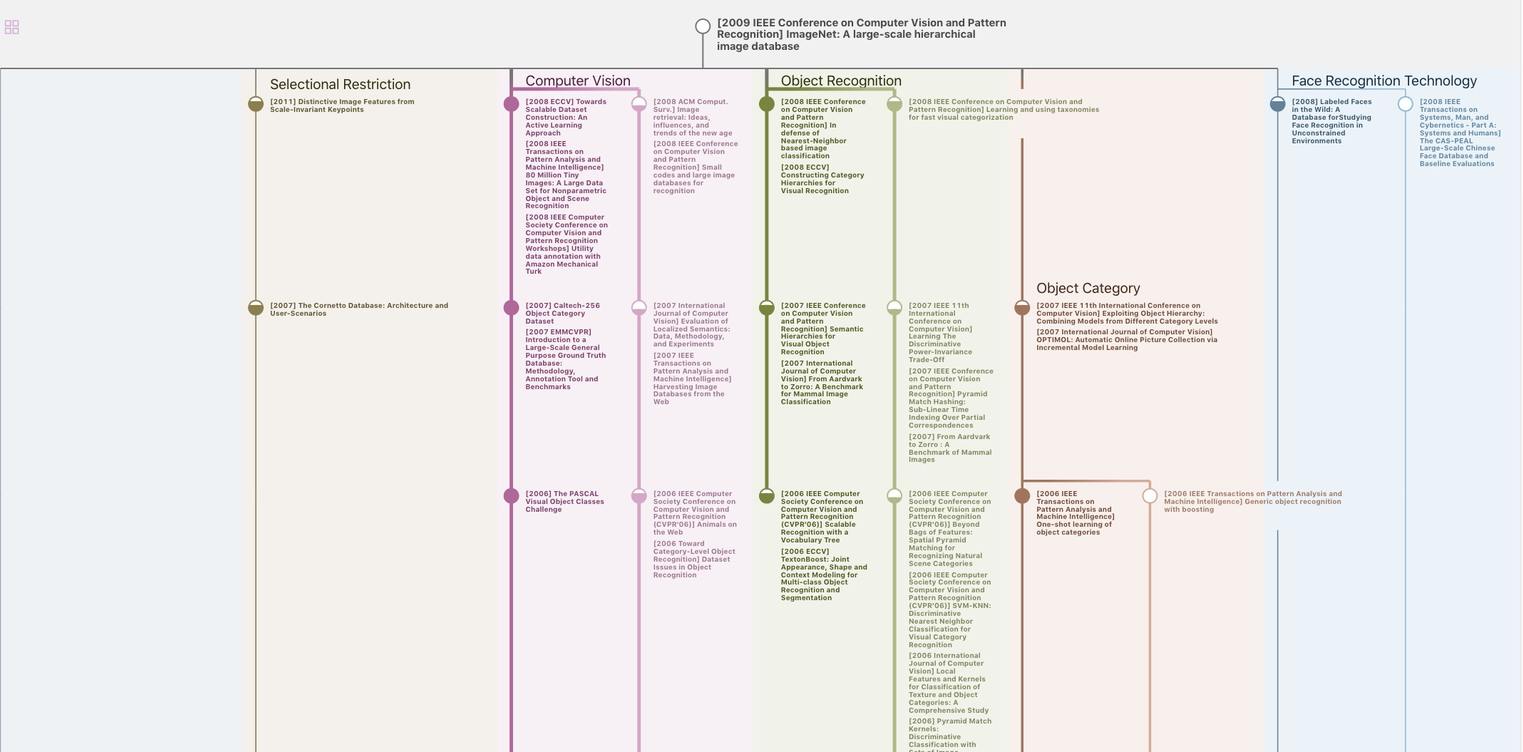
生成溯源树,研究论文发展脉络
Chat Paper
正在生成论文摘要