Segmentary group-sparsity self-representation learning and spectral clustering via double L21 norm
KNOWLEDGE-BASED SYSTEMS(2024)
摘要
With the rapid expansion of data dimensions, subspace representation learning, a method for mapping high -dimensional data samples to their corresponding underlying low -dimensional subspaces, has become an essential process for high -dimensional data clustering. Although the existing methods have achieved reliable data representation learning and precise clustering, few of them realized that the corrupted data points in the dataset will influence the linear representation of the others. When there are multiple heavily corrupted data in a dataset, the matrix of the self -representation coefficient would be influenced by these data. Therefore, this paper proposes the segmentary group -sparsity self -representation learning (SGSSL) and segmentary groupsparsity -based spectral clustering (SGSSC) models to eliminate their influence on representation learning and clustering results. We proposed that imposing varying degrees of row sparsity and column sparsity constraints on the representation coefficient matrix can prevent corrupted data from contaminating other data during the self -representation process, thus obtaining better spectral clustering results. Extensive experiments on several real datasets demonstrate that our proposed method can perform better than several related methods in recent years.
更多查看译文
关键词
Subspace learning,Self-representation learning,Spectral clustering,Group-sparsity,Symmetrical sparsity constraint
AI 理解论文
溯源树
样例
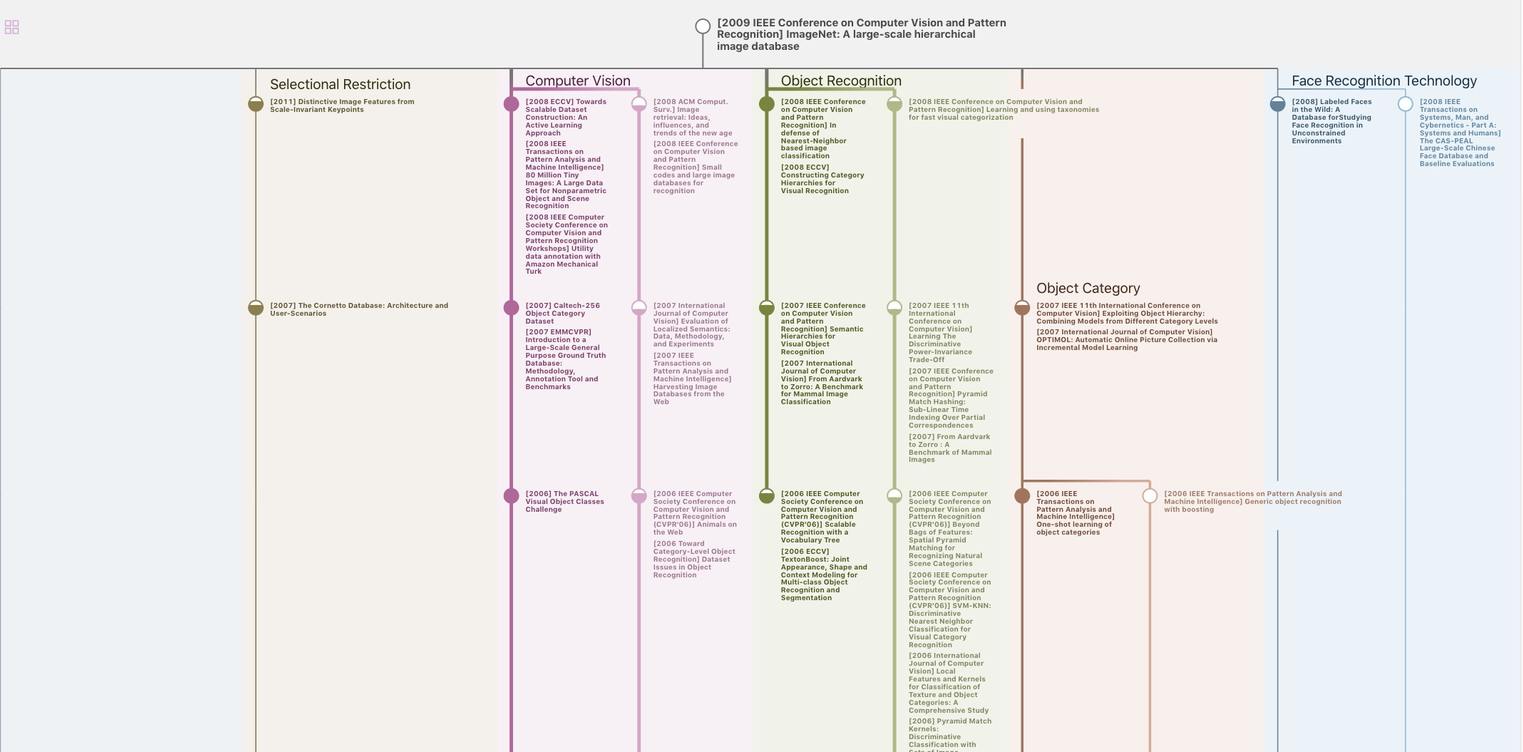
生成溯源树,研究论文发展脉络
Chat Paper
正在生成论文摘要