Efficiency and Security Trade-offs of Secure Multi-Party Computation for Machine Learning
International Conference on Knowledge-Based Intelligent Information & Engineering Systems(2023)
摘要
Machine learning methods require massive data collection to produce accurate predictions, raising privacy concerns. Secure Multi-Party Computation (SMPC) is one of the possible techniques to preserve data privacy during the computation process. Despite recent advances, the efficiency and scalability of SMPC in combination with machine learning remains an uncertain area. Moreover, various implementations of protocols exist that were optimized for the particular use cases, making the decision towards a suitable solution more complicated. The question of which protocol should be used under what circumstances naturally arises. In this case, when is defined as a security requirement and participant number. This work focuses on exploring the feasibility of using SMPC and machine learning in different settings. The work presents a comparison of selected multi-party computation protocols with different numbers of participants, malicious, and semihonest security models. Each relevant group of settings was constructed for the given input parameters, and the same inference scenario is performed within those settings. The inference scenario involves using a model trained on private statistical data inside a simulated multi-party computation session with a given number of participants and a security model. The results revealed a noticeable increase in computation time when moving from two to three participants, and the addition of a fourth participant led to a less substantial increase in computation time. Furthermore, as the number of participants continued to increase, the increase pattern became less aggressive. The study also noted that transitioning from a semi-honest to a malicious setting resulted in a significant increase in computation time. The results achieved help to outline the trade-offs between the protocol, the security model, and the number of participants, and help to choose the most efficient approach for future work.
更多查看译文
关键词
privacy-preserving,distributed secure computation,secure multi-party computation,machine learning,regression
AI 理解论文
溯源树
样例
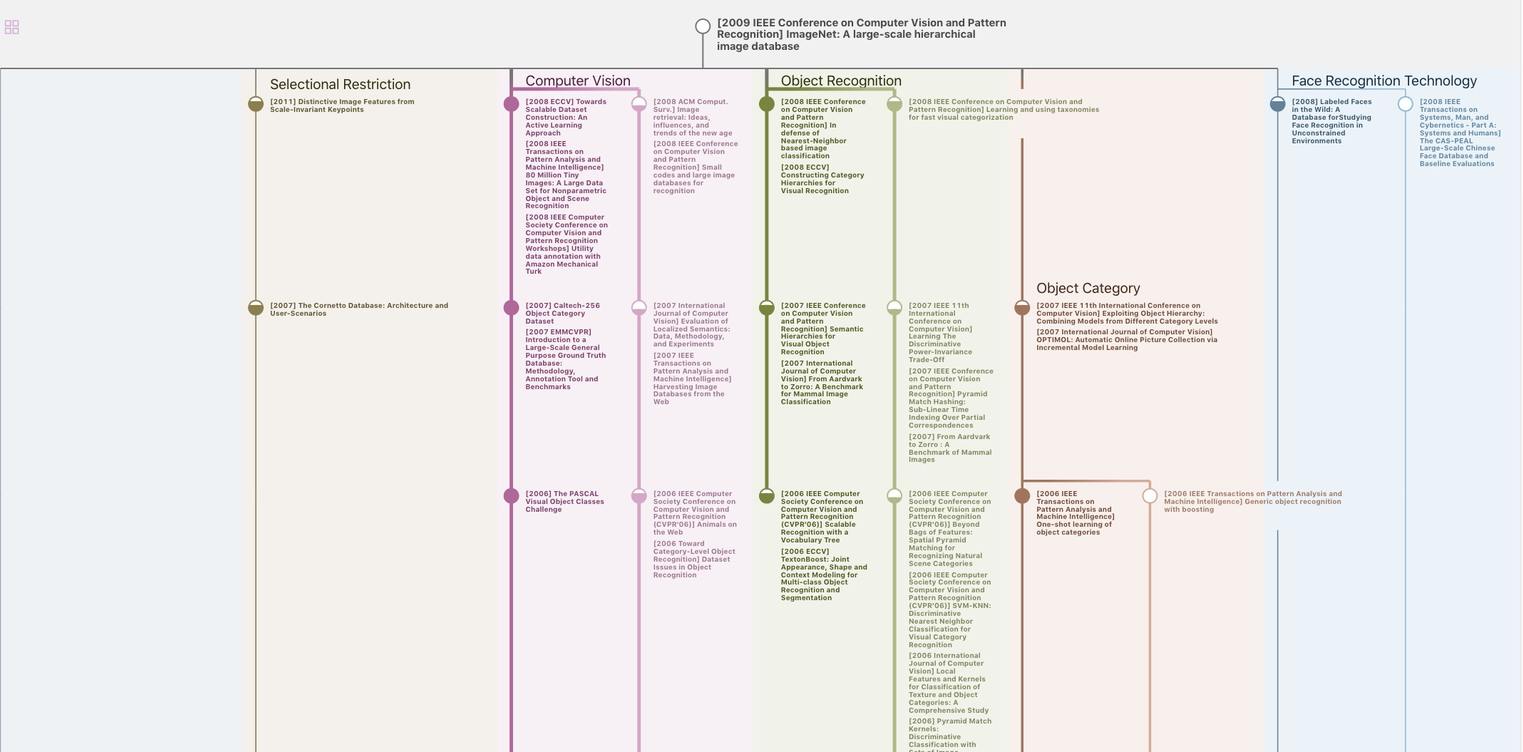
生成溯源树,研究论文发展脉络
Chat Paper
正在生成论文摘要