Deep Heterogeneous Contrastive Hyper-Graph Learning for In-the-Wild Context-Aware Human Activity Recognition
PROCEEDINGS OF THE ACM ON INTERACTIVE MOBILE WEARABLE AND UBIQUITOUS TECHNOLOGIES-IMWUT(2023)
摘要
Human Activity Recognition (HAR) is a challenging, multi-label classification problem as activities may co-occur and sensor signals corresponding to the same activity may vary in different contexts (e.g., different device placements). This paper proposes a Deep Heterogeneous Contrastive Hyper-Graph Learning (DHC-HGL) framework that captures heterogenous Context-Aware HAR (CA-HAR) hypergraph properties in a message-passing and neighborhood-aggregation fashion. Prior work only explored homogeneous or shallow-node-heterogeneous graphs. DHC-HGL handles heterogeneous CA-HAR data by innovatively 1) Constructing three different types of sub-hypergraphs that are each passed through different custom HyperGraph Convolution (HGC) layers designed to handle edge-heterogeneity and 2) Adopting a contrastive loss function to ensure node-heterogeneity. In rigorous evaluation on two CA-HAR datasets, DHC-HGL significantly outperformed state-ofthe-art baselines by 5.8% to 16.7% on Matthews Correlation Coefficient (MCC) and 3.0% to 8.4% on Macro F1 scores. UMAP visualizations of learned CA-HAR node embeddings are also presented to enhance model explainability. Our code is publicly available1 to encourage further research.
更多查看译文
关键词
human activity recognition,heterogeneous graph,hypergraph,graph neural networks
AI 理解论文
溯源树
样例
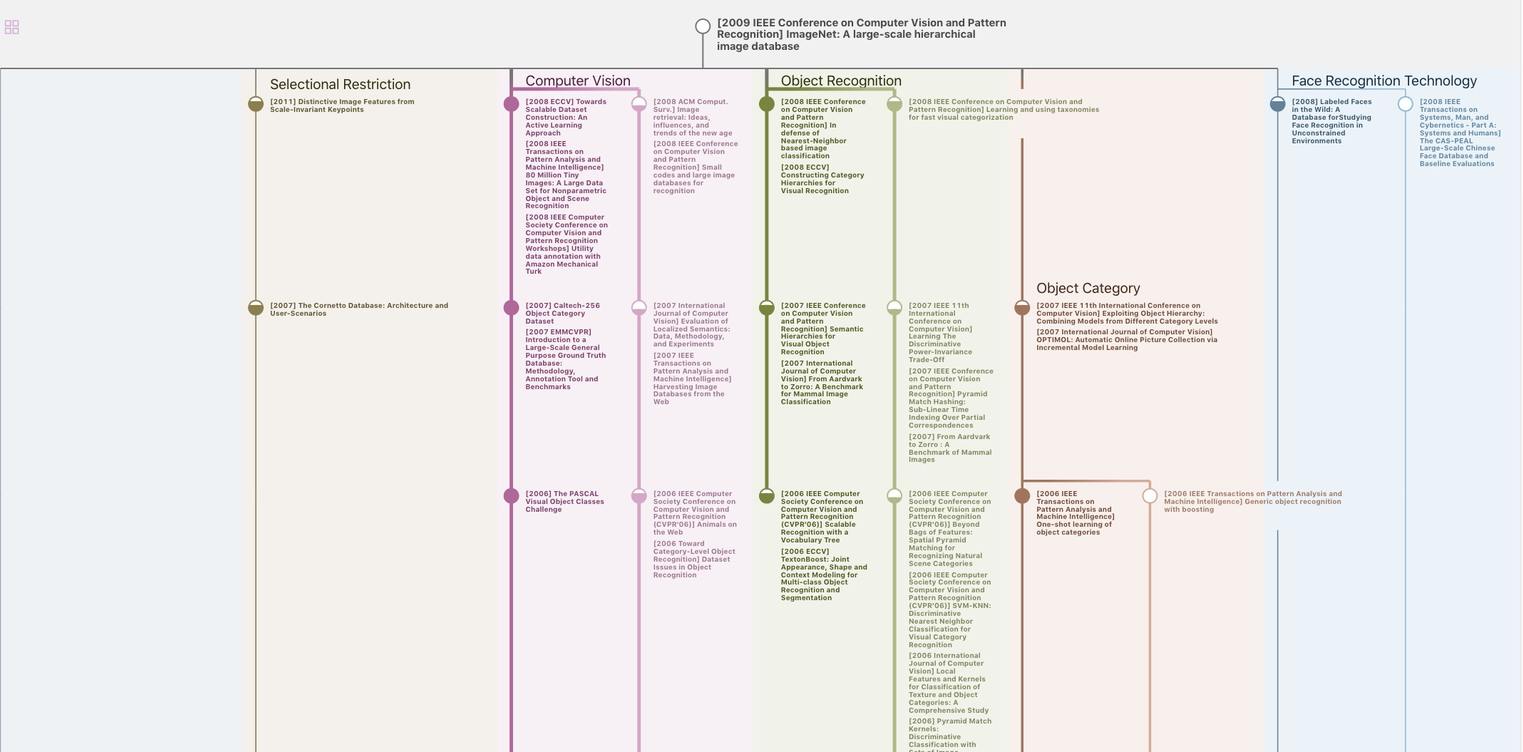
生成溯源树,研究论文发展脉络
Chat Paper
正在生成论文摘要