Cross-Modal Attentive Recalibration and Dynamic Fusion for Multispectral Pedestrian Detection
PATTERN RECOGNITION AND COMPUTER VISION, PRCV 2023, PT I(2024)
摘要
Multispectral pedestrian detection can provide accurate and reliable results from color-thermal modalities and has drawn much attention. However, how to effectively capture and leverage complementary information from multiple modalities for superior performance is still a core issue. This paper presents a Cross-Modal Attentive Recalibration and Dynamic Fusion Network (CMRF-Net) to adaptively recalibrate and dynamically fuse multi-modal features from multiple perspectives. CMRF-Net consists of a Cross-modal Attentive Feature Recalibration (CAFR) module and a Multi-Modal Dynamic Feature Fusion (MDFF) module in each feature extraction stage. The CAFR module recalibrates features by fully leveraging local and global complementary information in spatial- and channel-wise dimensions, leading to better cross-modal feature alignment and extraction. The MDFF module adopts dynamically learned convolutions to further exploit complementary information in kernel space, enabling more efficient multi-modal feature aggregation. Extensive experiments are conducted on three multispectral datasets to show the effectiveness and generalization of the proposed method and the state-of-the-art detection performance. Specifically, CMRF-Net can achieve 2.3% mAP gains over the baseline on FLIR dataset.
更多查看译文
关键词
Multispectral pedestrian detection,Cross-modal attentive feature recalibration,Multi-modal dynamic feature fusion
AI 理解论文
溯源树
样例
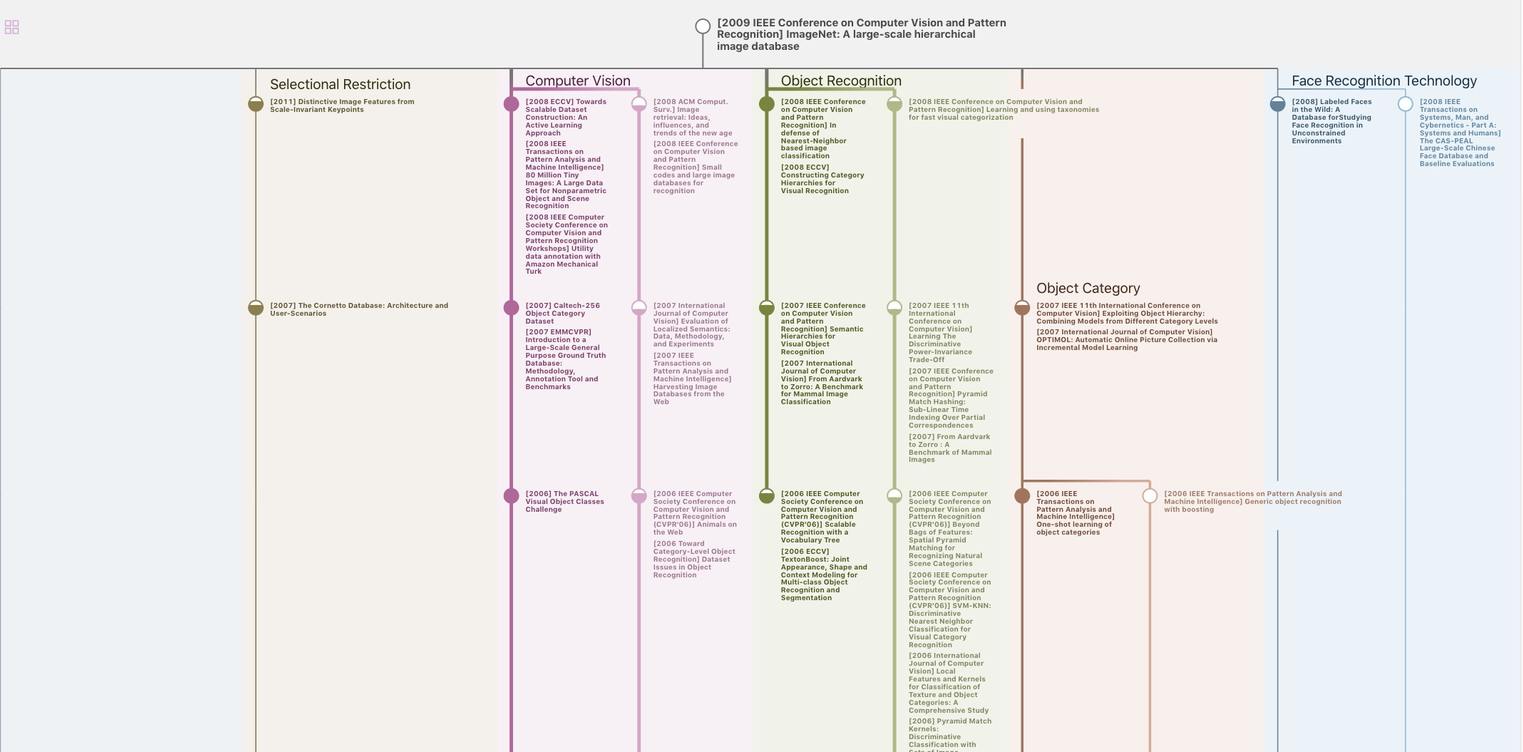
生成溯源树,研究论文发展脉络
Chat Paper
正在生成论文摘要