Detection of hypoplastic left heart syndrome anatomy from cardiovascular magnetic resonance images using machine learning
Magnetic Resonance Materials in Physics, Biology and Medicine(2024)
摘要
Objective The prospect of being able to gain relevant information from cardiovascular magnetic resonance (CMR) image analysis automatically opens up new potential to assist the evaluating physician. For machine-learning-based classification of complex congenital heart disease, only few studies have used CMR. Materials and methods This study presents a tailor-made neural network architecture for detection of 7 distinctive anatomic landmarks in CMR images of patients with hypoplastic left heart syndrome (HLHS) in Fontan circulation or healthy controls and demonstrates the potential of the spatial arrangement of the landmarks to identify HLHS. The method was applied to the axial SSFP CMR scans of 46 patients with HLHS and 33 healthy controls. Results The displacement between predicted and annotated landmark had a standard deviation of 8–17 mm and was larger than the interobserver variability by a factor of 1.1–2.0. A high overall classification accuracy of 98.7% was achieved. Discussion Decoupling the identification of clinically meaningful anatomic landmarks from the actual classification improved transparency of classification results. Information from such automated analysis could be used to quickly jump to anatomic positions and guide the physician more efficiently through the analysis depending on the detected condition, which may ultimately improve work flow and save analysis time.
更多查看译文
关键词
Congenital heart disease,Convolutional neural network,Classification,Anatomy
AI 理解论文
溯源树
样例
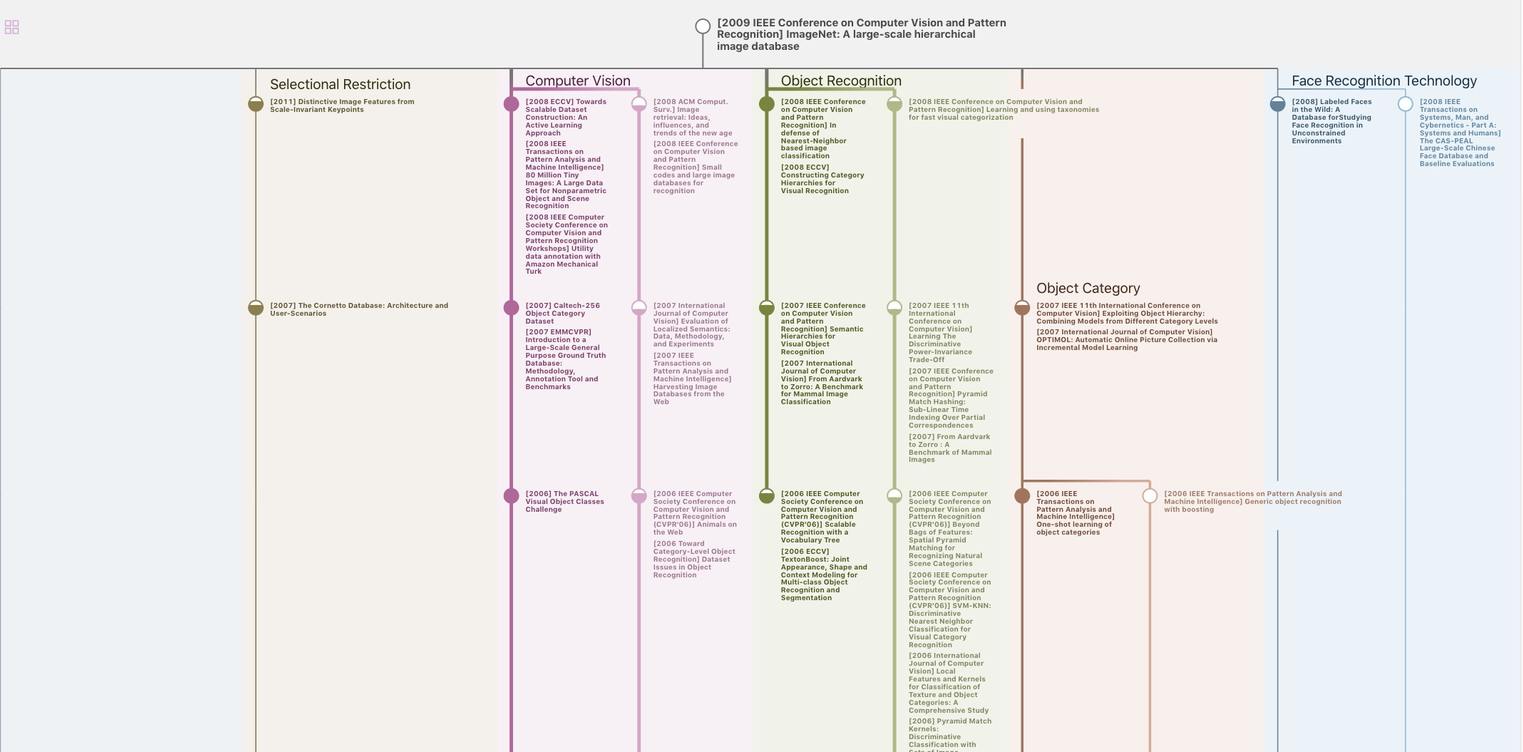
生成溯源树,研究论文发展脉络
Chat Paper
正在生成论文摘要