The Role of Deep Learning in Advancing Proactive Cybersecurity Measures for Smart Grid Networks: A Survey
IEEE Internet of Things Journal(2024)
摘要
As smart grids (SG) increasingly rely on advanced technologies like sensors
and communication systems for efficient energy generation, distribution, and
consumption, they become enticing targets for sophisticated cyberattacks. These
evolving threats demand robust security measures to maintain the stability and
resilience of modern energy systems. While extensive research has been
conducted, a comprehensive exploration of proactive cyber defense strategies
utilizing Deep Learning (DL) in SG remains scarce in the literature. This
survey bridges this gap, studying the latest DL techniques for proactive cyber
defense. The survey begins with an overview of related works and our distinct
contributions, followed by an examination of SG infrastructure. Next, we
classify various cyber defense techniques into reactive and proactive
categories. A significant focus is placed on DL-enabled proactive defenses,
where we provide a comprehensive taxonomy of DL approaches, highlighting their
roles and relevance in the proactive security of SG. Subsequently, we analyze
the most significant DL-based methods currently in use. Further, we explore
Moving Target Defense, a proactive defense strategy, and its interactions with
DL methodologies. We then provide an overview of benchmark datasets used in
this domain to substantiate the discourse. This is followed by a critical
discussion on their practical implications and broader impact on cybersecurity
in Smart Grids. The survey finally lists the challenges associated with
deploying DL-based security systems within SG, followed by an outlook on future
developments in this key field.
更多查看译文
关键词
Smart Grid,Early detection,Proactive Security,Deep Learning,Moving Target Defense
AI 理解论文
溯源树
样例
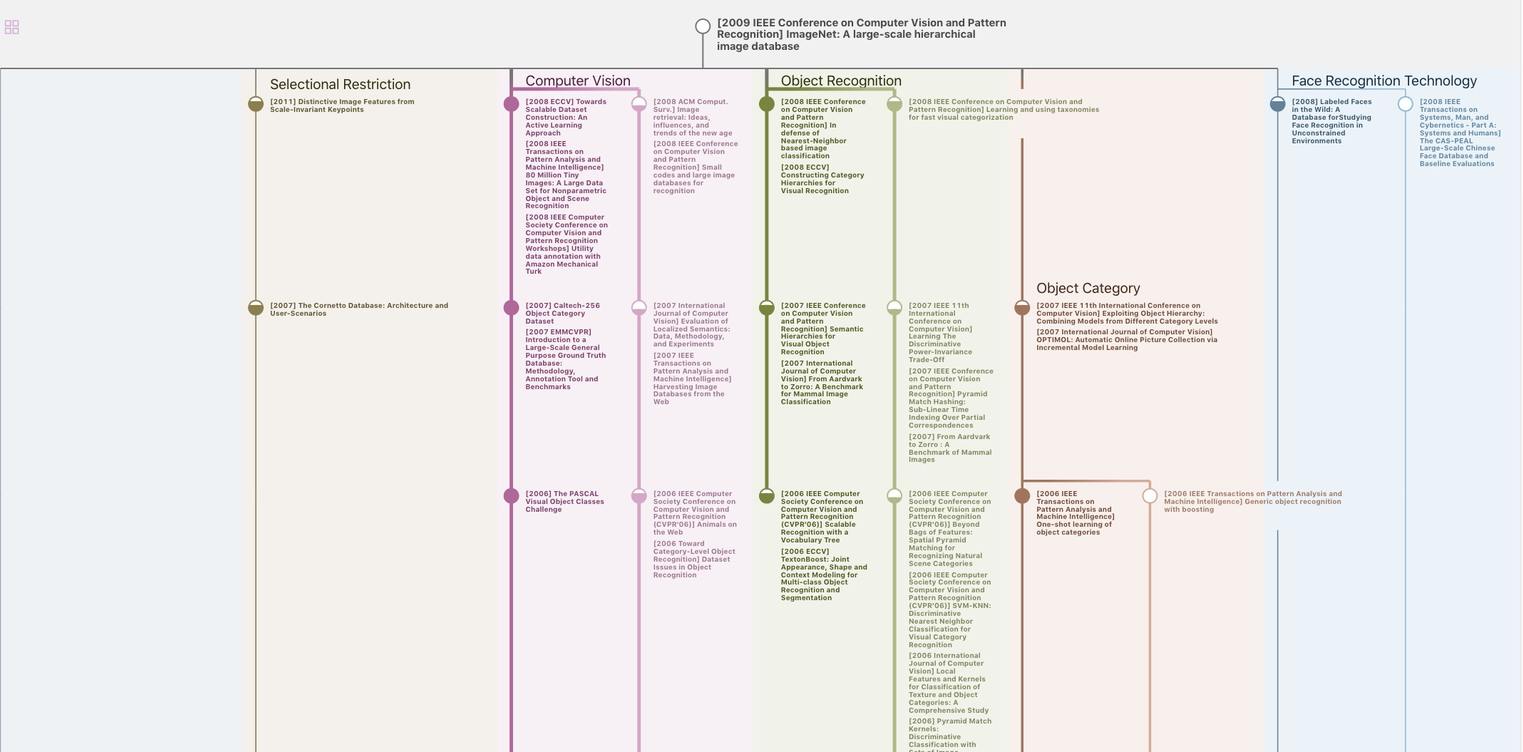
生成溯源树,研究论文发展脉络
Chat Paper
正在生成论文摘要