A Contrastive-Enhanced Ensemble Framework for Efficient Multi-Agent Reinforcement Learning
EXPERT SYSTEMS WITH APPLICATIONS(2024)
摘要
Multi-agent reinforcement learning is promising for real-world applications as it encourages agents to perceive and interact with their surrounding environment autonomously. However, sample efficiency is still a concern that prevents the application of multi-agent reinforcement learning in practice. A well-performing agent typically needs an abundance of interaction data for training, while obtaining numerous interaction data in a 'trial-and-error' manner is usually overhead-expensive or even infeasible for real-world tasks. In this paper, we propose a data-efficient framework, Contrastive-Enhanced Ensemble framework for Multi-Agent Reinforcement Learning (C2E-MARL), with the aim of training better-performing agents in the multi-agent system with fewer interaction data. Specifically, the proposed framework deploys an ensemble of centralized critic networks for action value estimation, i.e., it combines the outputs of multiple critic networks to estimate the action value. It makes full use of data from various perspectives to reduce the estimation error, which is helpful for efficient policy updating. Moreover, contrastive learning, a prevailing self-supervised technology, is employed to enhance the learning efficiency of submodels in C2E-MARL by augmenting the interaction data. Extensive experimental results compared with the state-of-the-art methods on three multi-agent benchmark scenarios demonstrate the superiority of C2E-MARL in terms of efficiency and performance.
更多查看译文
关键词
Multi-agent reinforcement learning,Sample efficiency,Ensemble learning,Contrastive learning,Multi-agent system
AI 理解论文
溯源树
样例
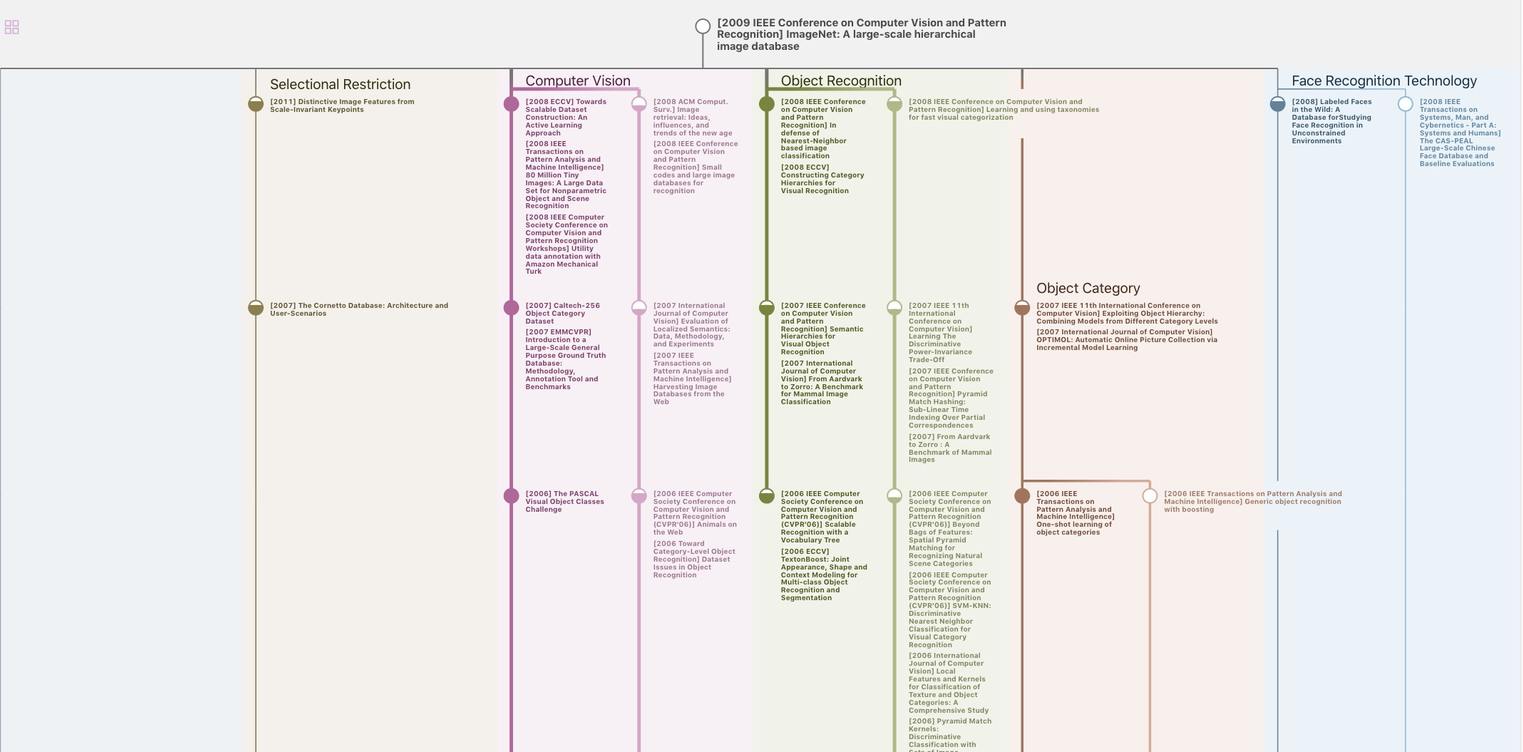
生成溯源树,研究论文发展脉络
Chat Paper
正在生成论文摘要