Combining general and personal models for epilepsy detection with hyperdimensional computing
ARTIFICIAL INTELLIGENCE IN MEDICINE(2024)
摘要
Epilepsy is a highly prevalent chronic neurological disorder with great negative impact on patients' daily lives. Despite this there is still no adequate technological support to enable epilepsy detection and continuous outpatient monitoring in everyday life. Hyperdimensional (HD) computing is a promising method for epilepsy detection via wearable devices, characterized by a simpler learning process and lower memory requirements compared to other methods. In this work, we demonstrate additional avenues in which HD computing and the manner in which its models are built and stored can be used to better understand, compare and create more advanced machine learning models for epilepsy detection. These possibilities are not feasible with other state-of-the-art models, such as random forests or neural networks. We compare inter-subject model similarity of different classes (seizure and non-seizure), study the process of creating general models from personal ones, and finally posit a method of combining personal and general models to create hybrid models. This results in an improved epilepsy detection performance. We also tested knowledge transfer between models trained on two different datasets. The attained insights are highly interesting not only from an engineering perspective, to create better models for wearables, but also from a neurological perspective, to better understand individual epilepsy patterns.
更多查看译文
关键词
Hyperdimensional computing,Epilepsy,Personal models,General models,Hybrid models,Seizure detection
AI 理解论文
溯源树
样例
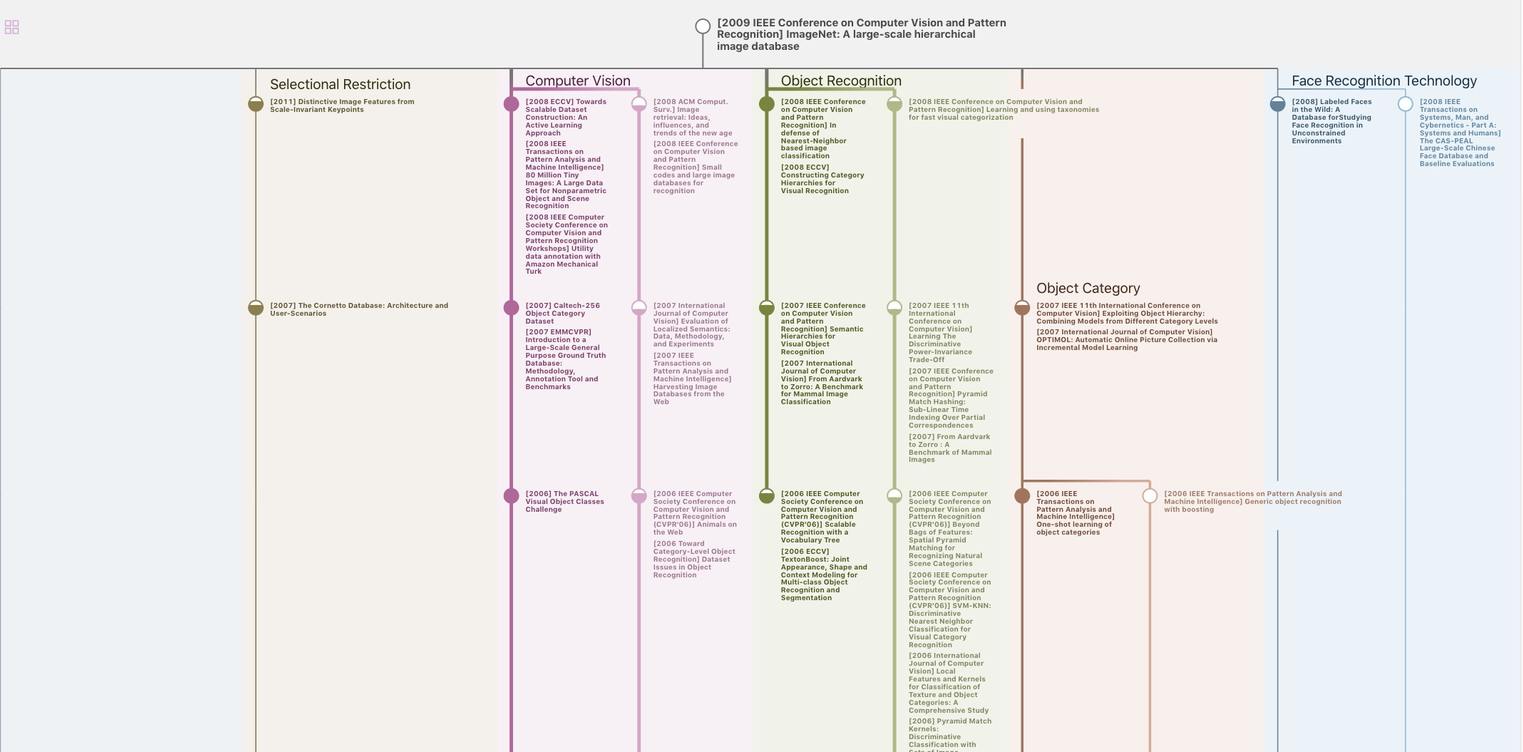
生成溯源树,研究论文发展脉络
Chat Paper
正在生成论文摘要