A New Dataflow Implementation to Improve Energy Efficiency of Monolithic 3D Systolic Arrays
CoRR(2024)
摘要
Systolic arrays are popular for executing deep neural networks (DNNs) at the
edge. Low latency and energy efficiency are key requirements in edge devices
such as drones and autonomous vehicles. Monolithic 3D (MONO3D) is an emerging
3D integration technique that offers ultra-high bandwidth among processing and
memory elements with a negligible area overhead. Such high bandwidth can help
meet the ever-growing latency and energy efficiency demands for DNNs. This
paper presents a novel implementation for weight stationary (WS) dataflow in
MONO3D systolic arrays, called WS-MONO3D. WS-MONO3D utilizes multiple resistive
RAM layers and SRAM with high-density vertical interconnects to multicast
inputs and perform high-bandwidth weight pre-loading while maintaining the same
order of multiply-and-accumulate operations as in native WS dataflow.
Consequently, WS-MONO3D eliminates input and weight forwarding cycles and,
thus, provides up to 40
native WS implementation in 2D at iso-configuration. WS-MONO3D also provides
10X improvement in inference per second per watt per footprint due to multiple
vertical tiers. Finally, we also show that temperature impacts the energy
efficiency benefits in WS-MONO3D.
更多查看译文
AI 理解论文
溯源树
样例
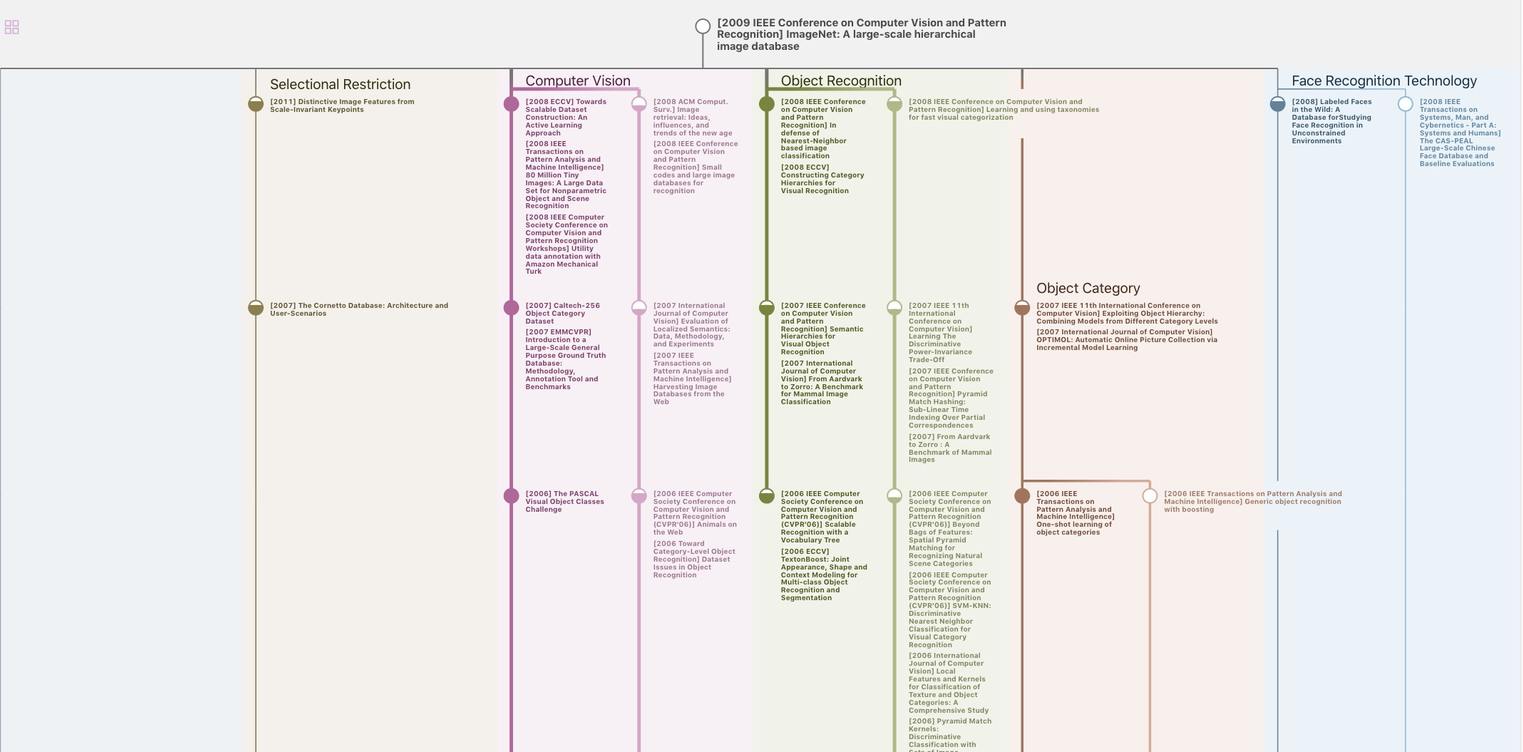
生成溯源树,研究论文发展脉络
Chat Paper
正在生成论文摘要