Deep-learning-augmented microscopy for super-resolution imaging of nanoparticles
OPTICS EXPRESS(2024)
摘要
Conventional optical microscopes generally provide blurry and indistinguishable images for subwavelength nanostructures. However, a wealth of intensity and phase information is hidden in the corresponding diffraction -limited optical patterns and can be used for the recognition of structural features, such as size, shape, and spatial arrangement. Here, we apply a deep -learning framework to improve the spatial resolution of optical imaging for metal nanostructures with regular shapes yet varied arrangement. A convolutional neural network (CNN) is constructed and pre -trained by the optical images of randomly distributed gold nanoparticles as input and the corresponding scanning -electron microscopy images as ground truth. The CNN is then learned to recover reversely the non -diffracted super -resolution images of both regularly arranged nanoparticle dimers and randomly clustered nanoparticle multimers from their blurry optical images. The profiles and orientations of these structures can also be reconstructed accurately. Moreover, the same network is extended to deblur the optical images of randomly cross -linked silver nanowires. Most sections of these intricate nanowire nets are recovered well with a slight discrepancy near their intersections. This deep -learning augmented framework opens new opportunities for computational super -resolution optical microscopy with many potential applications in the fields of bioimaging and nanoscale fabrication and characterization. It could also be applied to significantly enhance the resolving capability of low -magnification scanning -electron microscopy. (c) 2023 Optica Publishing Group under the terms of the Optica Open Access Publishing Agreement
更多查看译文
AI 理解论文
溯源树
样例
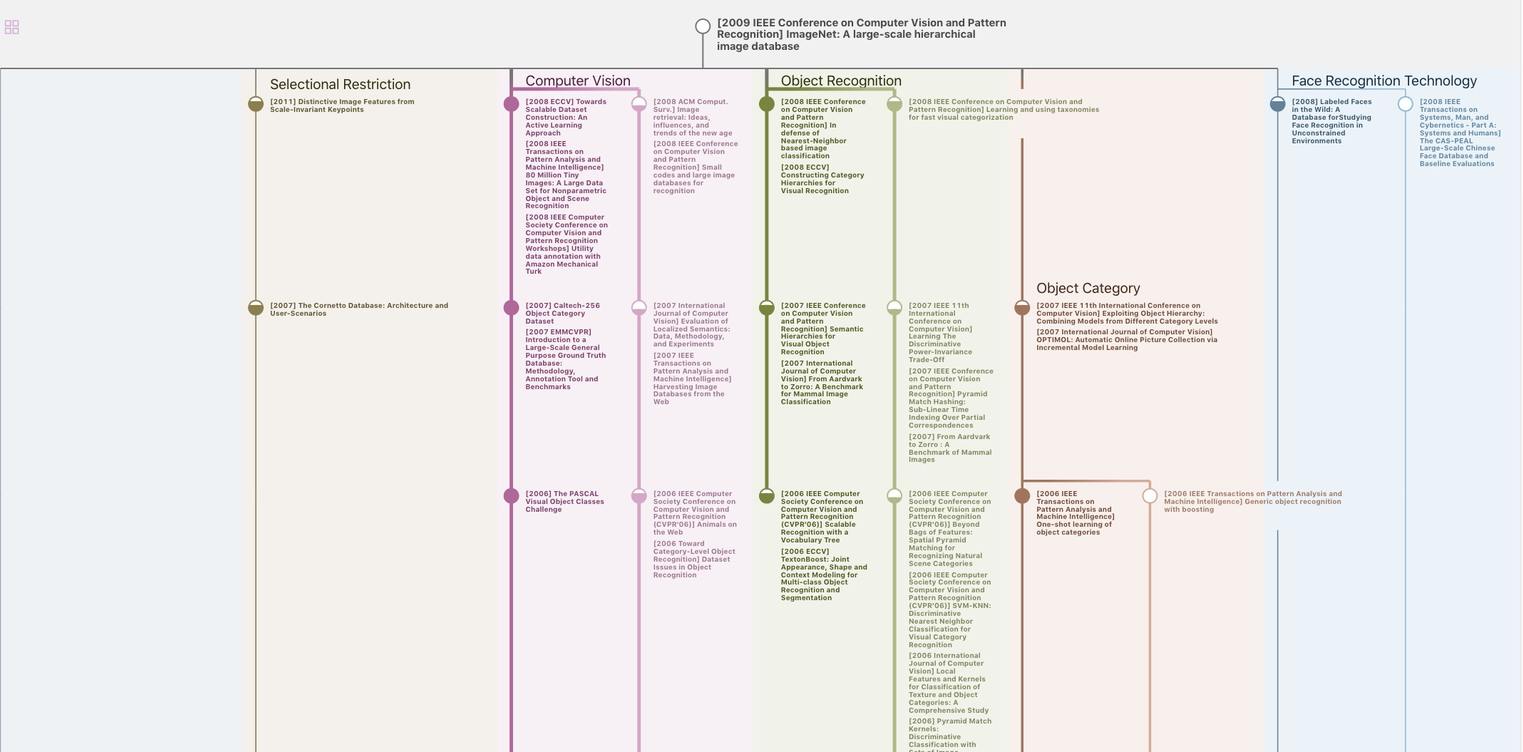
生成溯源树,研究论文发展脉络
Chat Paper
正在生成论文摘要