Universal Cross-Lingual Data Generation for Low Resource ASR
IEEE-ACM TRANSACTIONS ON AUDIO SPEECH AND LANGUAGE PROCESSING(2024)
摘要
Significant advances in end-to-end (E2E) automatic speech recognition (ASR) have primarily been concentrated on languages rich in annotated data. Nevertheless, a large proportion of languages worldwide, which are typically low-resource, continue to pose significant challenges. To address this issue, this study presents a novel speech synthesis framework based on data splicing that leverages self-supervised learning (SSL) units from Hidden Unit BERT (HuBERT) as universal phonetic units. In our framework, the SSL phonetic units serve as crucial bridges between speech and text across different languages. By leveraging these units, we successfully splice speech fragments from high-resource languages into synthesized speech that maintains acoustic coherence with text from low-resource languages. To further enhance the practicality of the framework, we introduce a sampling strategy based on confidence scores assigned to the speech segments used in data splicing. The application of this confidence sampling strategy in data splicing significantly accelerates ASR model convergence and enhances overall ASR performance. Experimental results on the CommonVoice dataset show 25-35% relative improvement for four Indo-European languages and about 20% for Turkish using a 4-gram language model for rescoring, under a 10-hour low-resource setup. Furthermore, we showcase the scalability of our framework by incorporating a larger unsupervised speech corpus for generating speech fragments in data splicing, resulting in an additional 10% relative improvement.
更多查看译文
关键词
Splicing,Data models,Phonetics,Training,Speech synthesis,Dictionaries,Data mining,Low-resource speech recognition,text-to-seech,data splicing,self-supervised learning
AI 理解论文
溯源树
样例
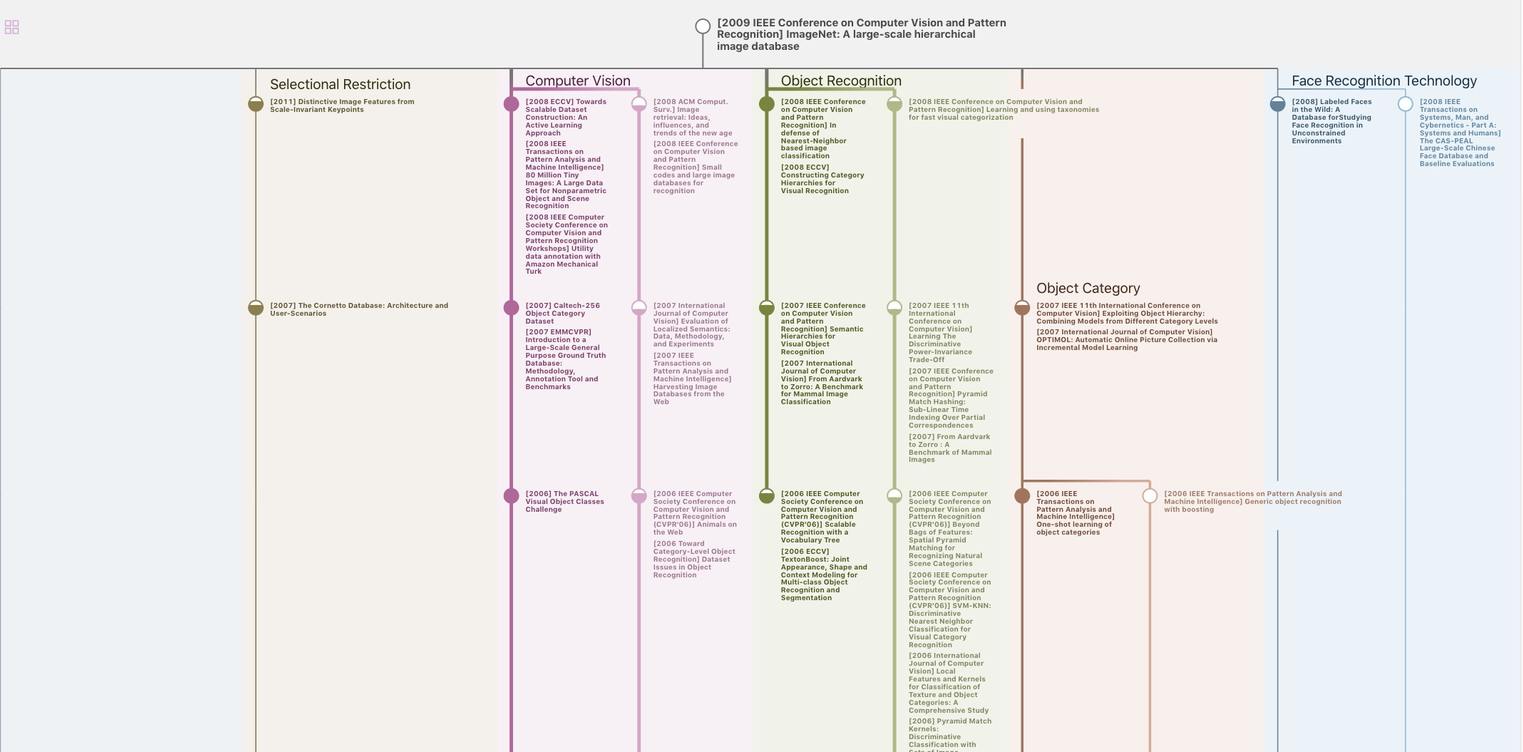
生成溯源树,研究论文发展脉络
Chat Paper
正在生成论文摘要