On the hardness of learning under symmetries
CoRR(2024)
摘要
We study the problem of learning equivariant neural networks via gradient
descent. The incorporation of known symmetries ("equivariance") into neural
nets has empirically improved the performance of learning pipelines, in domains
ranging from biology to computer vision. However, a rich yet separate line of
learning theoretic research has demonstrated that actually learning shallow,
fully-connected (i.e. non-symmetric) networks has exponential complexity in the
correlational statistical query (CSQ) model, a framework encompassing gradient
descent. In this work, we ask: are known problem symmetries sufficient to
alleviate the fundamental hardness of learning neural nets with gradient
descent? We answer this question in the negative. In particular, we give lower
bounds for shallow graph neural networks, convolutional networks, invariant
polynomials, and frame-averaged networks for permutation subgroups, which all
scale either superpolynomially or exponentially in the relevant input
dimension. Therefore, in spite of the significant inductive bias imparted via
symmetry, actually learning the complete classes of functions represented by
equivariant neural networks via gradient descent remains hard.
更多查看译文
关键词
Equivariance,statistical query,lower bound,computational hardness,invariance,symmetry,neural networks
AI 理解论文
溯源树
样例
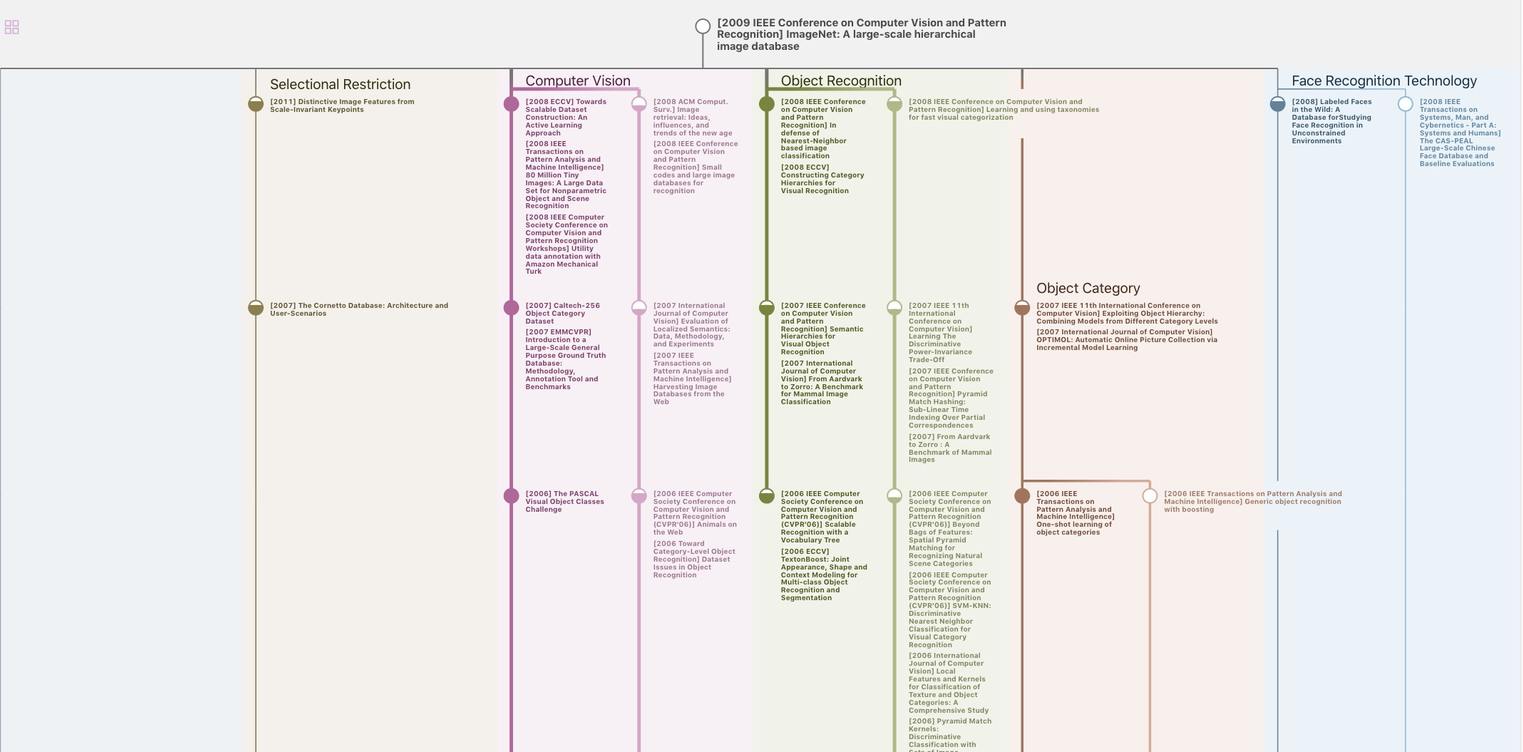
生成溯源树,研究论文发展脉络
Chat Paper
正在生成论文摘要