Deep Learning Based Superposition Coded Modulation for Hierarchical Semantic Communications over Broadcast Channels
CoRR(2024)
摘要
We consider multi-user semantic communications over broadcast channels. While
most existing works consider that each receiver requires either the same or
independent semantic information, this paper explores the scenario where the
semantic information desired by different receivers is different but
correlated. In particular, we investigate semantic communications over Gaussian
broadcast channels where the transmitter has a common observable source but the
receivers wish to recover hierarchical semantic information in adaptation to
their channel conditions. Inspired by the capacity achieving property of
superposition codes, we propose a deep learning based superposition coded
modulation (DeepSCM) scheme. Specifically, the hierarchical semantic
information is first extracted and encoded into basic and enhanced feature
vectors. A linear minimum mean square error (LMMSE) decorrelator is then
developed to obtain a refinement from the enhanced features that is
uncorrelated with the basic features. Finally, the basic features and their
refinement are superposed for broadcasting after probabilistic modulation.
Experiments are conducted for two-receiver image semantic broadcasting with
coarse and fine classification as hierarchical semantic tasks. DeepSCM
outperforms the benchmarking coded-modulation scheme without a superposition
structure, especially with large channel disparity and high order modulation.
It also approaches the performance upperbound as if there were only one
receiver.
更多查看译文
AI 理解论文
溯源树
样例
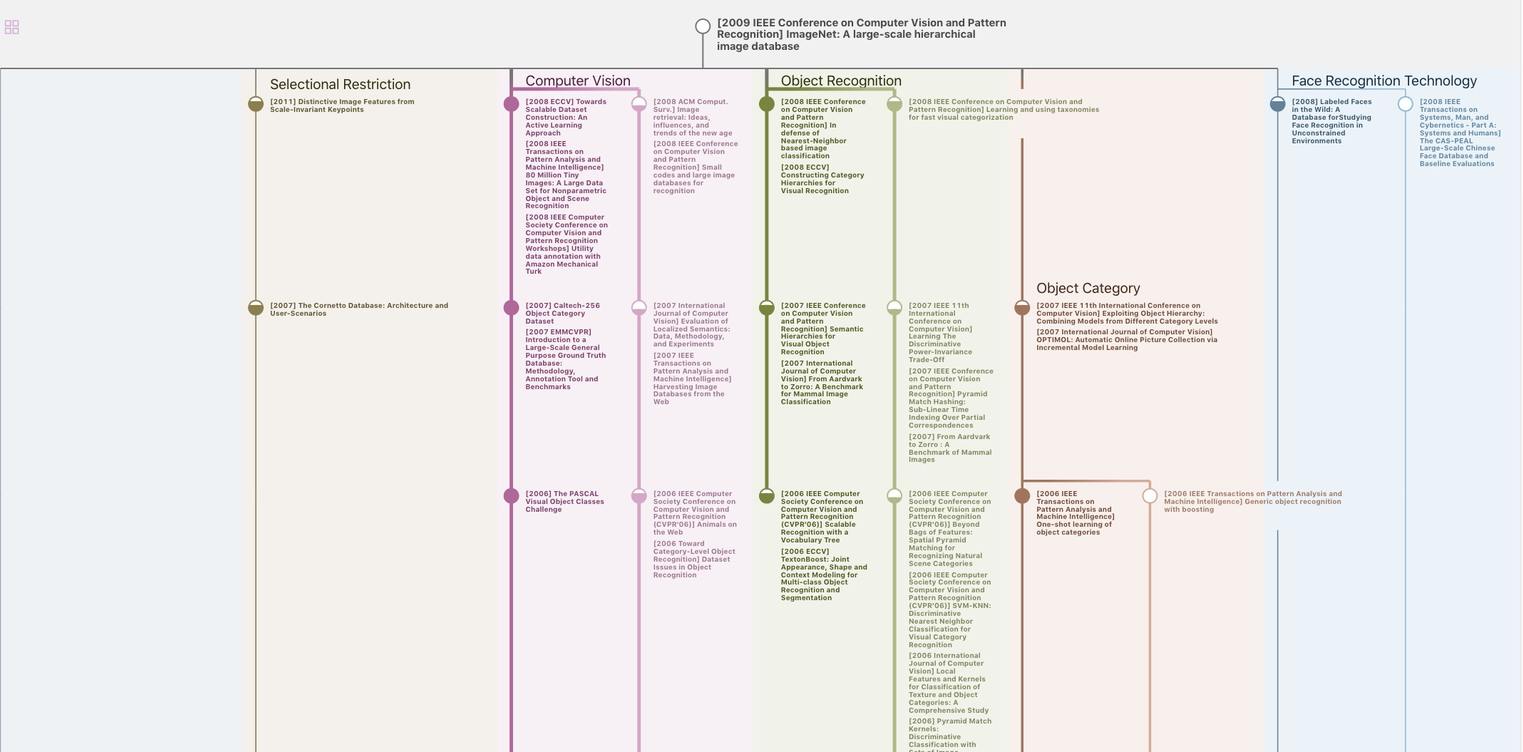
生成溯源树,研究论文发展脉络
Chat Paper
正在生成论文摘要