Improving Domain Transfer of Robot Dynamics Models with Geometric System Identification and Learned Friction Compensation
2023 IEEE-RAS 22ND INTERNATIONAL CONFERENCE ON HUMANOID ROBOTS, HUMANOIDS(2023)
摘要
Dynamic simulation is an important part of the design pipeline for robot controllers, but there is often a significant performance gap between the simulation domain and the real world. This sim-to-real gap makes transferring controllers developed in one simulation environment to other simulations or to real hardware systems difficult and time-consuming. Here, we introduce an approach to reduce this gap for the MIT Humanoid by using physically-feasible system identification methods to match dynamics models across domains, combined with neural networks to model any residual dynamics, such as friction. Using data from our real hardware system as the ground truth, we develop models for transfer from two separate simulation environments to hardware, as well as transfer between the two simulations. Finally, we show experimental results using our fitted dynamic models and characterize our domain transfer success.
更多查看译文
AI 理解论文
溯源树
样例
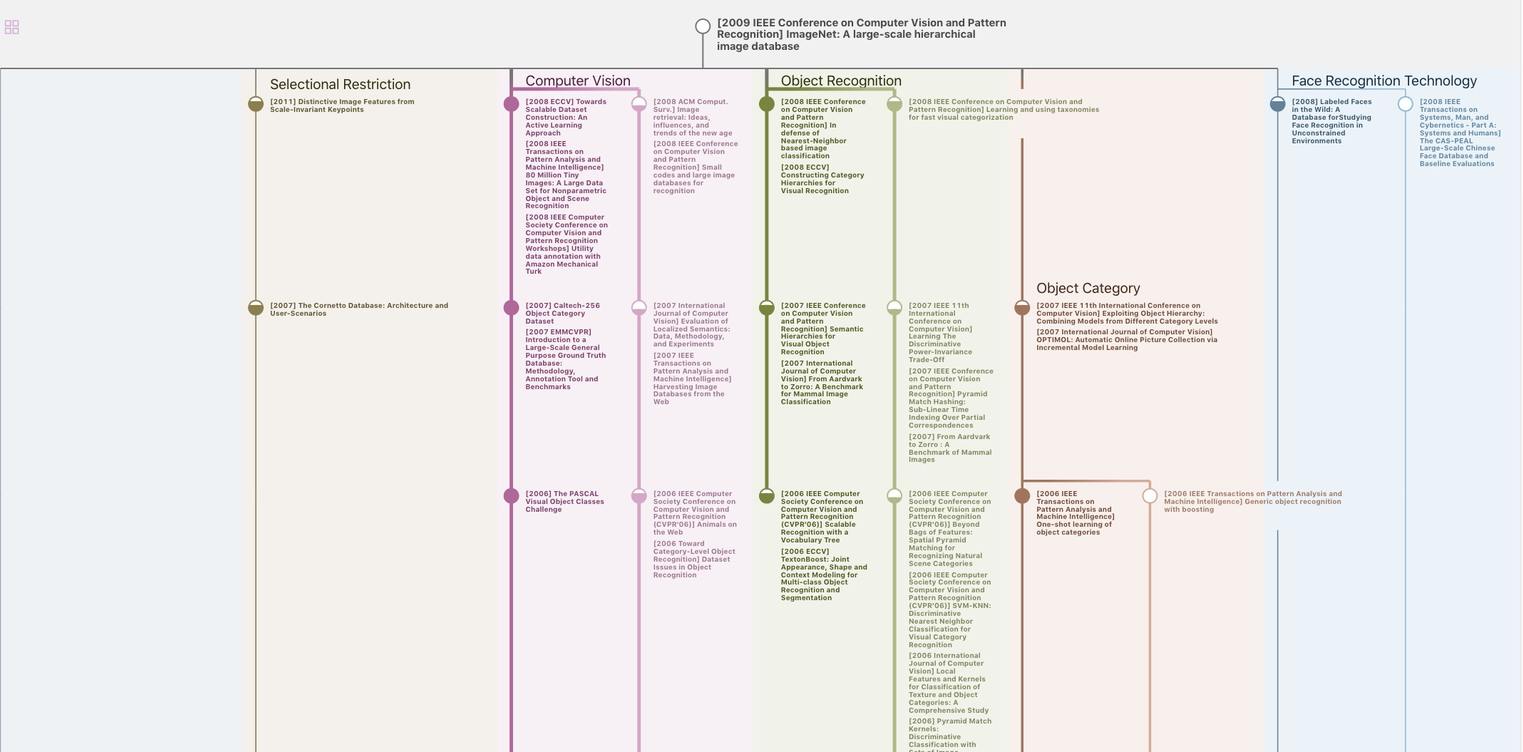
生成溯源树,研究论文发展脉络
Chat Paper
正在生成论文摘要