Class correlation correction for unbiased scene graph generation
PATTERN RECOGNITION(2024)
摘要
The long-tail distribution in the scene graph generation (SGG) task has spurred immense interest in unbiased SGG. However, current state-of-the-art debiasing techniques extract statistics from the independent category, while ignoring the correlation between categories. To address this issue, we propose a simple but effective method, class correlation correction, to aggregate dependency knowledge among various classes. Specifically, given biased predictions, two kinds of debiasing transformations are developed employing the class correlation aware label to recover the unbiased estimates. We also propose to retrain SGG models with the biasing transformations adapted to the biased data distribution. The proposed debiasing method is evaluated using several biased datasets that are constructed from CIFAR-10 and Fashion-MNIST, as well as the commonly used SGG dataset and Caltech 101 dataset. Multiple evaluation metrics are used to assess the debiasing performance, and the results of extensive experiments show the effectiveness of the proposed method. The Pytorch (R) implementations can be downloaded from an open-source GitHub project https://github.com/Dlut-lab-zmn/class-correlation-correction.
更多查看译文
关键词
Unbiased scene graph generation,Class correlation correction,Debiasing transformation,Biasing transformation
AI 理解论文
溯源树
样例
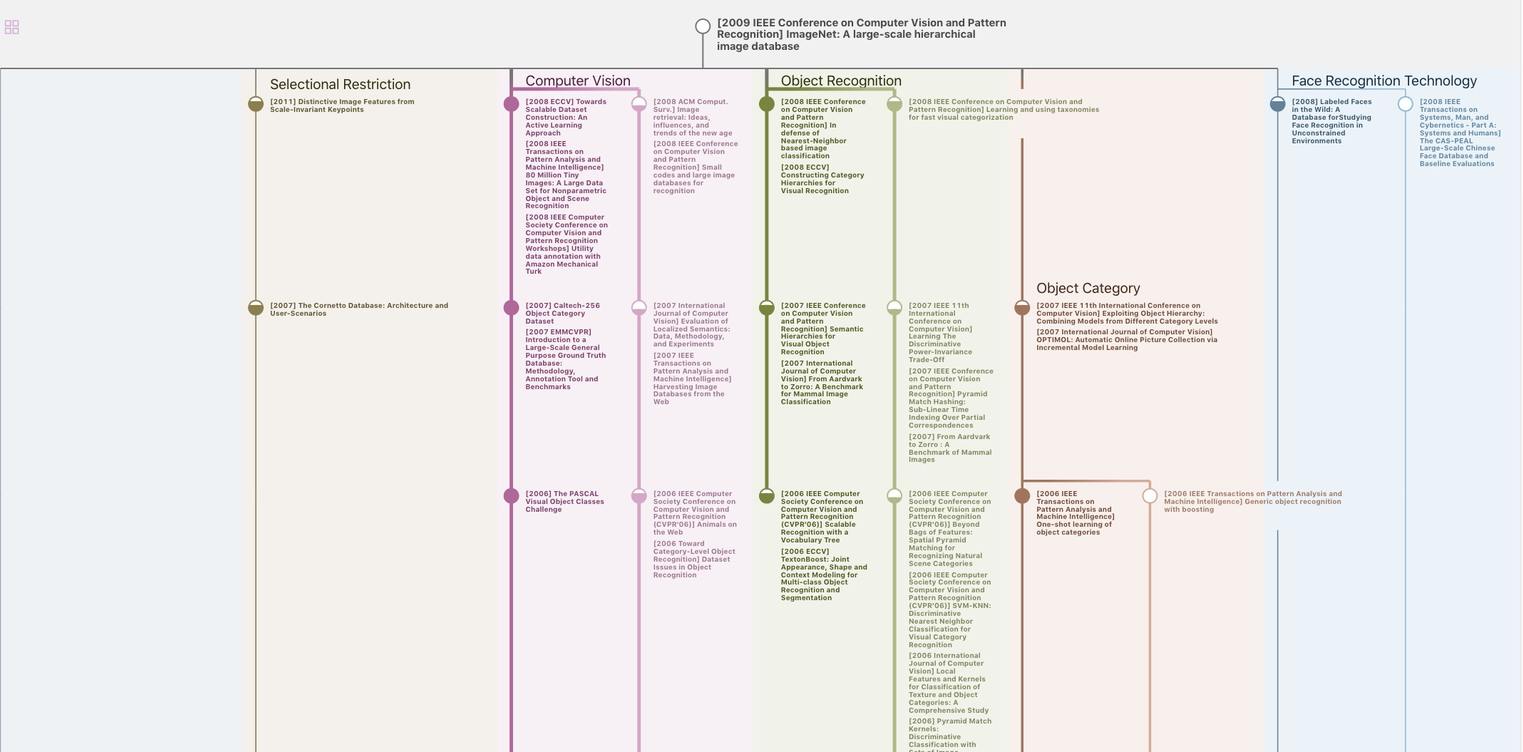
生成溯源树,研究论文发展脉络
Chat Paper
正在生成论文摘要