Unlocking Efficiency: Understanding End-to-End Performance in Distributed Analytics Pipelines
MILCOM 2023 - 2023 IEEE MILITARY COMMUNICATIONS CONFERENCE(2023)
摘要
Internet of Things (IoT) devices have proliferated across a wide range of smart environments that generate vast amounts of data, necessitating the emergence of distributed edge-cloud infrastructures. However, these IoT-edge-cloud infrastructures encounter several challenges in providing efficient and effective services to users, such as real-time service delivery, robustness and resilience, and efficient deployment. Moreover, recent advancements in machine learning, particularly within the realm of deep learning, have ushered in a new era for IoT applications. These applications increasingly lean on data-intensive models to perform a multitude of functions, including classification, detection, data analytics, and decision-making. A key aspect is that many of these tasks exhibit sensitivity to latency and hinge on the deployment of models at the network's edge and on how to efficiently handle data-intensive workloads, especially when network conditions are constrained. In response to these challenges, we present an analytical discussion that delves into the intricacies of distributed IoT pipelines and workloads deployed across both edge and cloud computing environments.
更多查看译文
关键词
Edge computing,ML inference,IoT,Analytical Pipelines
AI 理解论文
溯源树
样例
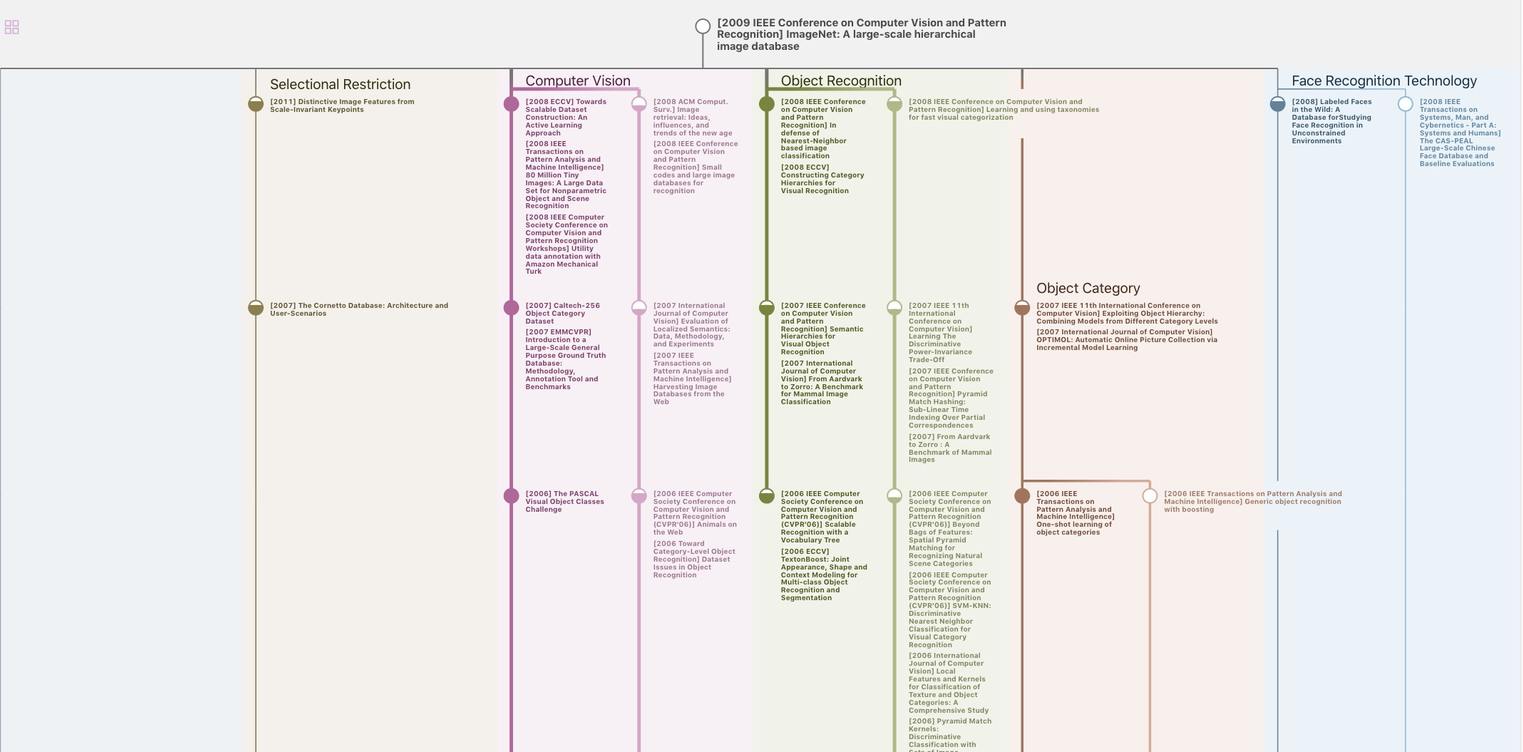
生成溯源树,研究论文发展脉络
Chat Paper
正在生成论文摘要