\MetaEmotionNet: Spatial-Spectral-Temporal-Based Attention 3-D Dense Network With Meta-Learning for EEG Emotion Recognition
IEEE Transactions on Instrumentation and Measurement(2024)
摘要
Emotion recognition has become an important area in affective computing. Emotion recognition based on multichannel electroencephalogram (EEG) signals has gradually become popular in recent years. However, on one hand, how to make full use of different EEG features and the discriminative local patterns among the features for various emotions is challenging. Existing methods ignore the complementarity among the spatial-spectral-temporal features and discriminative local patterns in all features, which limits the classification performance. On the other hand, when dealing with cross-subject emotion recognition, existing transfer learning (TL) methods need a lot of training data. At the same time, it is extremely expensive and time-consuming to collect the labeled EEG data, which is not conducive to the wide application of emotion recognition models for new subjects. To solve the above challenges, we propose a novel spatial-spectral-temporal-based attention 3-D dense network (SST-Net) with meta-learning, named MetaEmotionNet, for emotion recognition. Specifically, MetaEmotionNet integrates the spatial-spectral-temporal features simultaneously in a unified network framework through two-stream fusion. At the same time, the 3-D attention mechanism can adaptively explore discriminative local patterns. In addition, a meta-learning algorithm is applied to reduce dependence on training data. Experiments demonstrate that the MetaEmotionNet is superior to the baseline models on two benchmark datasets.
更多查看译文
关键词
Affective computing,attention mechanism,electroencephalogram (EEG),emotion recognition,meta-learning
AI 理解论文
溯源树
样例
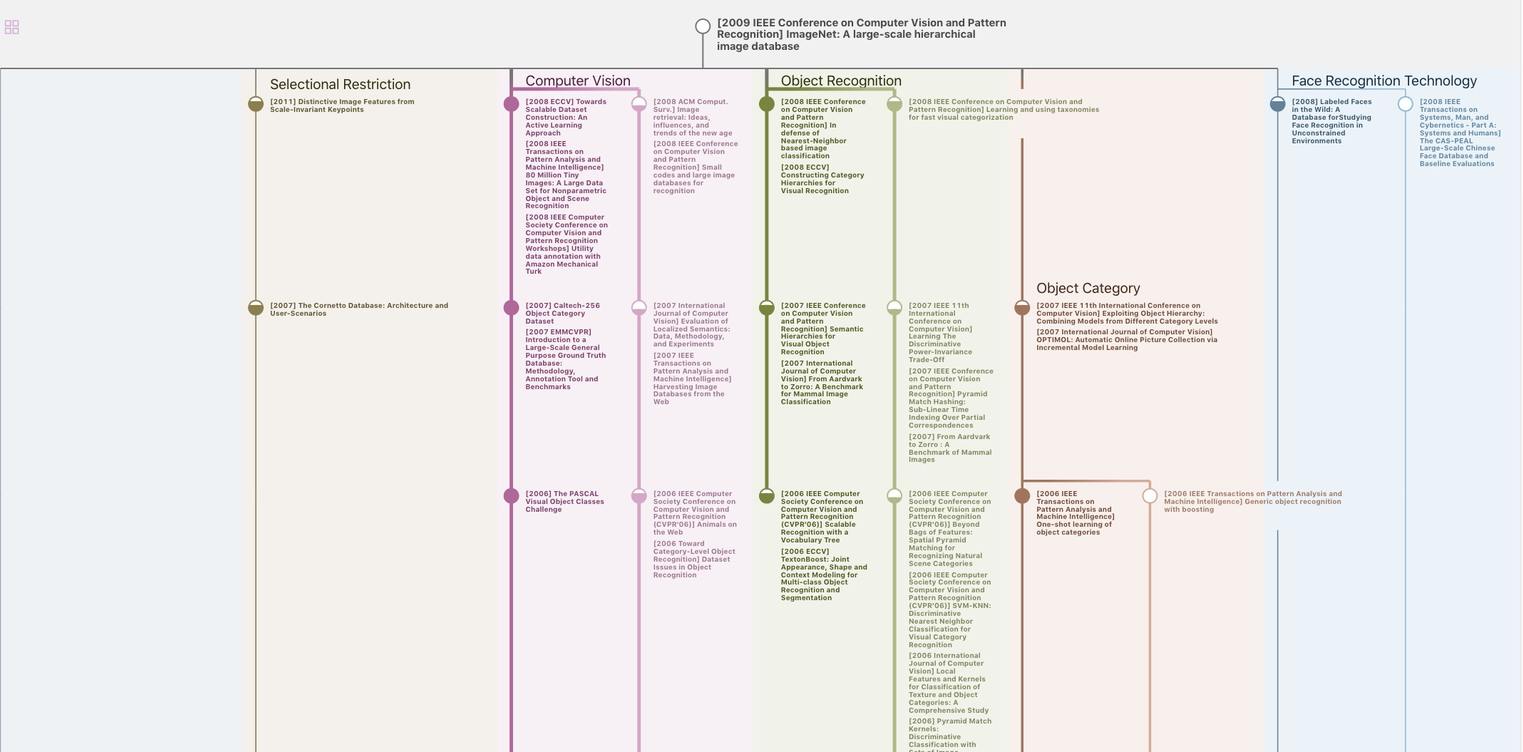
生成溯源树,研究论文发展脉络
Chat Paper
正在生成论文摘要