Enhancing Communication Efficiency and Training Time Uniformity in Federated Learning through Multi-Branch Networks and the Oort Algorithm
ALGORITHMS(2024)
摘要
In this study, we present a federated learning approach that combines a multi-branch network and the Oort client selection algorithm to improve the performance of federated learning systems. This method successfully addresses the significant issue of non-iid data, a challenge not adequately tackled by the commonly used MFedAvg method. Additionally, one of the key innovations of this research is the introduction of uniformity, a metric that quantifies the disparity in training time amongst participants in a federated learning setup. This novel concept not only aids in identifying stragglers but also provides valuable insights into assessing the fairness and efficiency of the system. The experimental results underscore the merits of the integrated multi-branch network with the Oort client selection algorithm and highlight the crucial role of uniformity in designing and evaluating federated learning systems.
更多查看译文
关键词
federated learning,uniformity,communication efficiency,client selection,multi-branch network
AI 理解论文
溯源树
样例
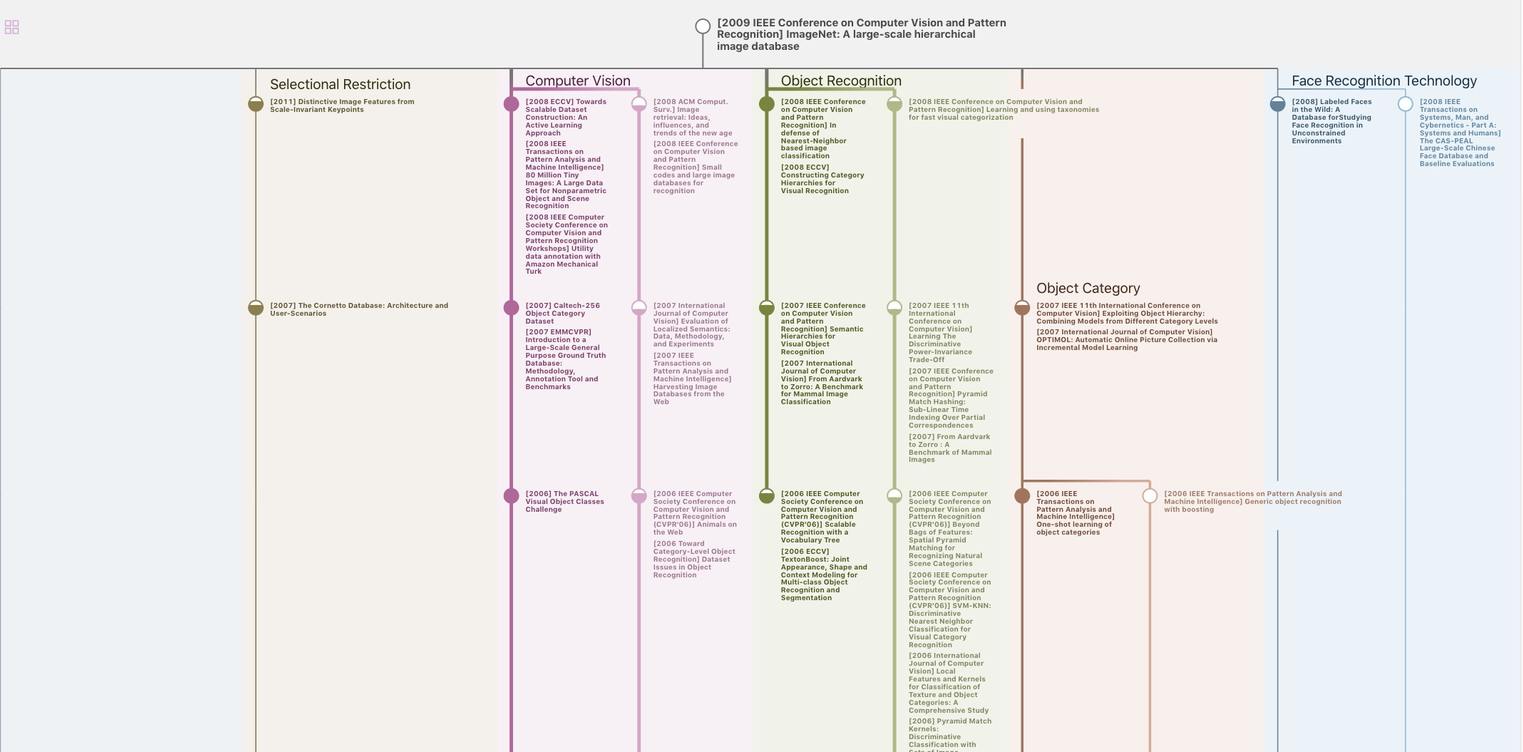
生成溯源树,研究论文发展脉络
Chat Paper
正在生成论文摘要