Societal Versus Encoded Stereotypes in Text Encoders
2023 IEEE 35TH INTERNATIONAL CONFERENCE ON TOOLS WITH ARTIFICIAL INTELLIGENCE, ICTAI(2023)
摘要
Text encoders have recently been convicted of encoding unjustified social stereotypes, which lead models to make biased and prejudiced predictions when trained on downstream tasks such as sentiment analysis or question answering. The presence of bias in NLP models is dangerous since it promotes the divide between different social groups. Thus, attempts at mitigating biases from NLP models constitute an active line of research. However, these methods assume that models replicate exactly the same stereotypes ingrained in society's impression, leading to potential inaccuracies in the normative framing of bias. In this work we confirm that text encoders are indeed biased. Nonetheless, we show that encoded biases are slightly different from survey-based biases proper to human prejudice. We ground our findings on the Stereotype Content Model, an acclaimed framework to interpret stereotypes, prejudice and inter-group relations in social psychology.
更多查看译文
关键词
Language models,Social bias,Debiasing,Stereotype Content Model
AI 理解论文
溯源树
样例
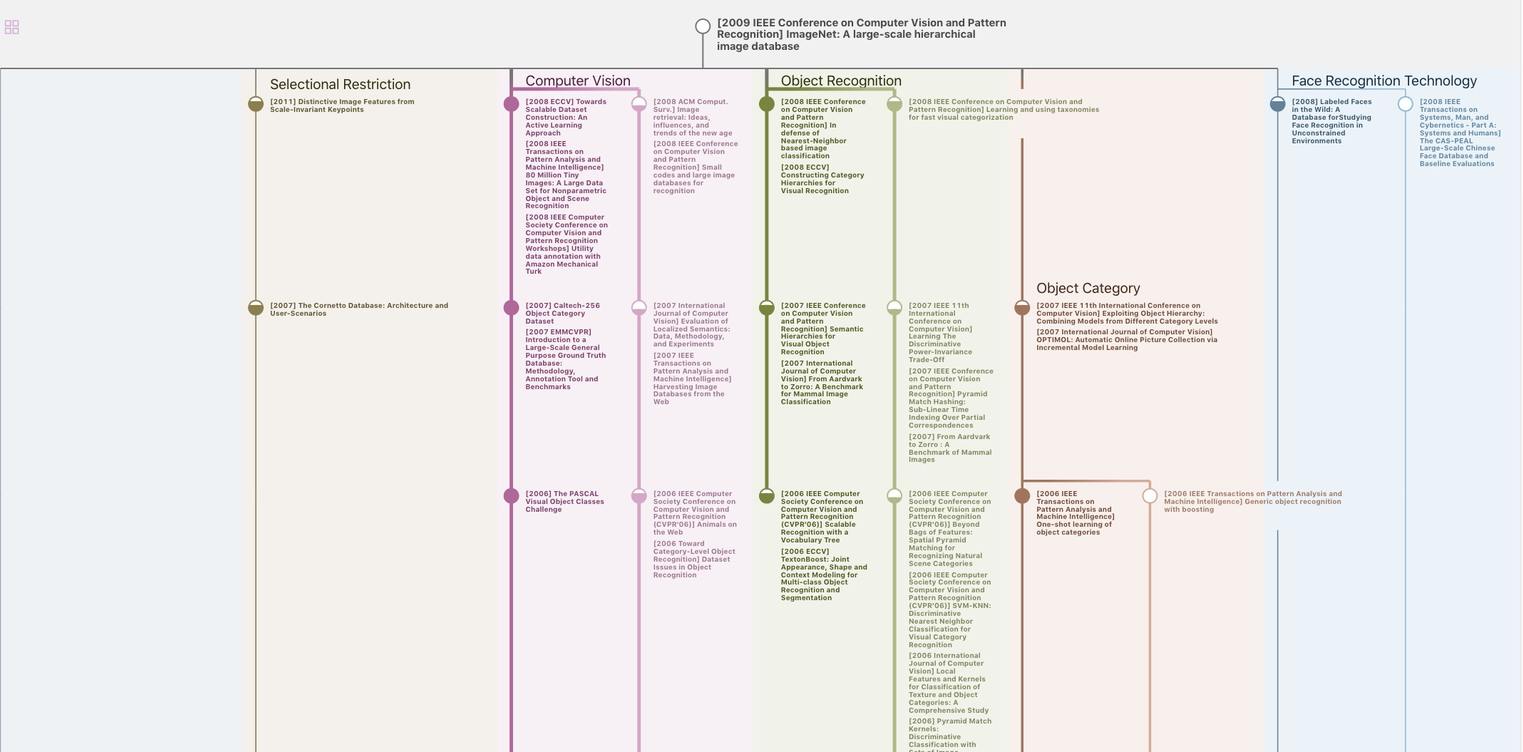
生成溯源树,研究论文发展脉络
Chat Paper
正在生成论文摘要