Image Restoration Through Generalized Ornstein-Uhlenbeck Bridge
CoRR(2023)
摘要
Diffusion models possess powerful generative capabilities enabling the
mapping of noise to data using reverse stochastic differential equations.
However, in image restoration tasks, the focus is on the mapping relationship
from low-quality images to high-quality images. To address this, we introduced
the Generalized Ornstein-Uhlenbeck Bridge (GOUB) model. By leveraging the
natural mean-reverting property of the generalized OU process and further
adjusting the variance of its steady-state distribution through the Doob's
h-transform, we achieve diffusion mappings from point to point with minimal
cost. This allows for end-to-end training, enabling the recovery of
high-quality images from low-quality ones. Additionally, we uncovered the
mathematical essence of some bridge models, all of which are special cases of
the GOUB and empirically demonstrated the optimality of our proposed models.
Furthermore, benefiting from our distinctive parameterization mechanism, we
proposed the Mean-ODE model that is better at capturing pixel-level information
and structural perceptions. Experimental results show that both models achieved
state-of-the-art results in various tasks, including inpainting, deraining, and
super-resolution. Code is available at https://github.com/Hammour-steak/GOUB.
更多查看译文
AI 理解论文
溯源树
样例
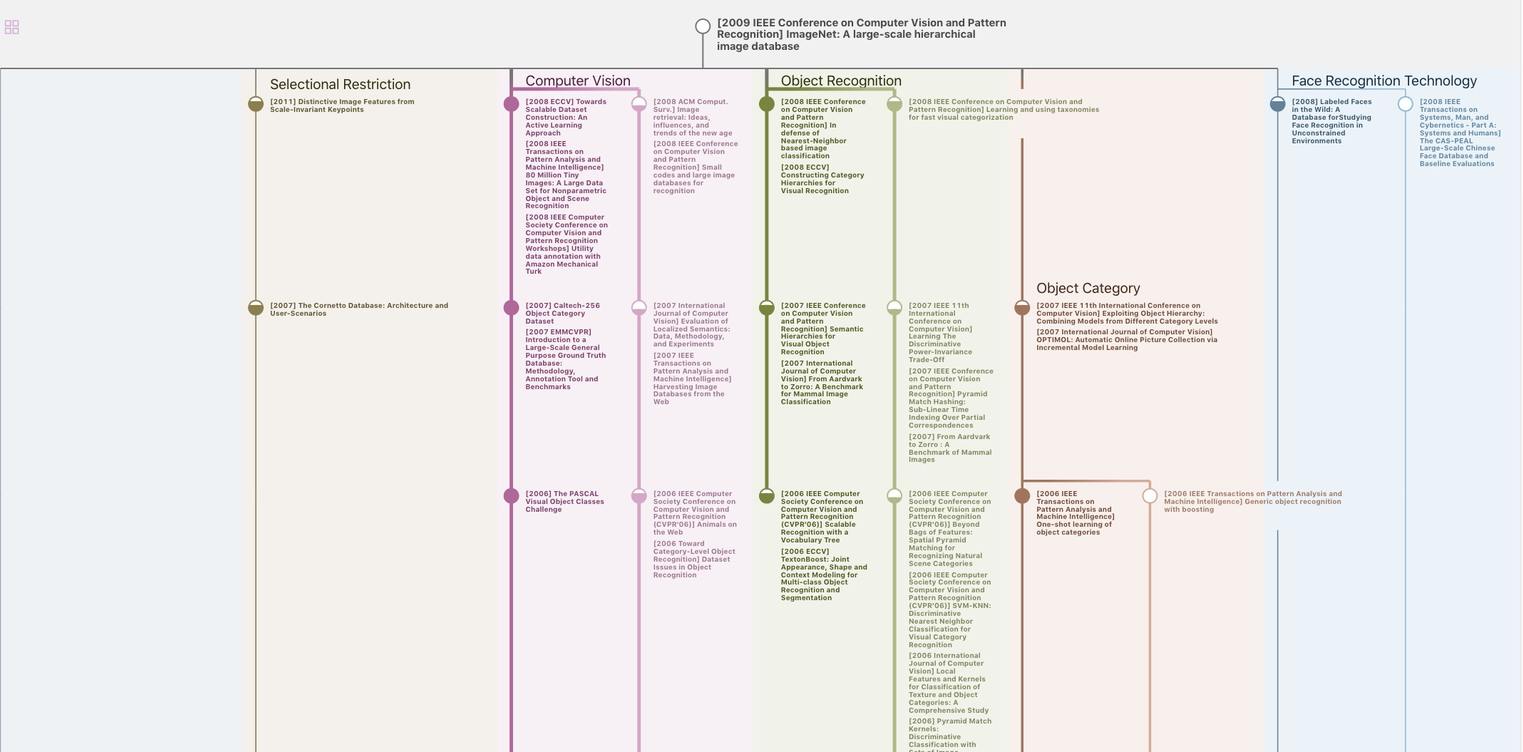
生成溯源树,研究论文发展脉络
Chat Paper
正在生成论文摘要