WeightRelay: Efficient Heterogeneous Federated Learning on Time Series
ADVANCES IN ARTIFICIAL INTELLIGENCE, AI 2023, PT I(2024)
摘要
Federated learning for heterogeneous devices aims to obtain models of various structural configurations in order to fit multiple devices according to their hardware configurations and external environments. Existing solutions train those heterogeneous models simultaneously, which requires extra cost (e.g. computation, communication, or data) to transfer knowledge between models. In this paper, we proposed a method, namely, weight relay (WeightRelay), that could get heterogeneous models without any extra training cost. Specifically, we find that, compared with the classic random weight initialization, initializing the weight of a large neural network with the weight of a well-trained small network could reduce the training epoch and still maintain a similar performance. Therefore, we could order models from the smallest and train them one by one. Each model (except the first one) can be initialized with the prior model's trained weight for training cost reduction. In the experiment, we evaluate the weight relay on 128-time series datasets from multiple domains, and the result confirms the effectiveness of WeightRelay. More theoretical analysis and code can be found in (https://github.com/Wensi-Tang/DPSN/blob/master/AJCAI23_wensi_fedTSC.pdf).
更多查看译文
关键词
Time series classification,Federated learning,Heterogeneous model
AI 理解论文
溯源树
样例
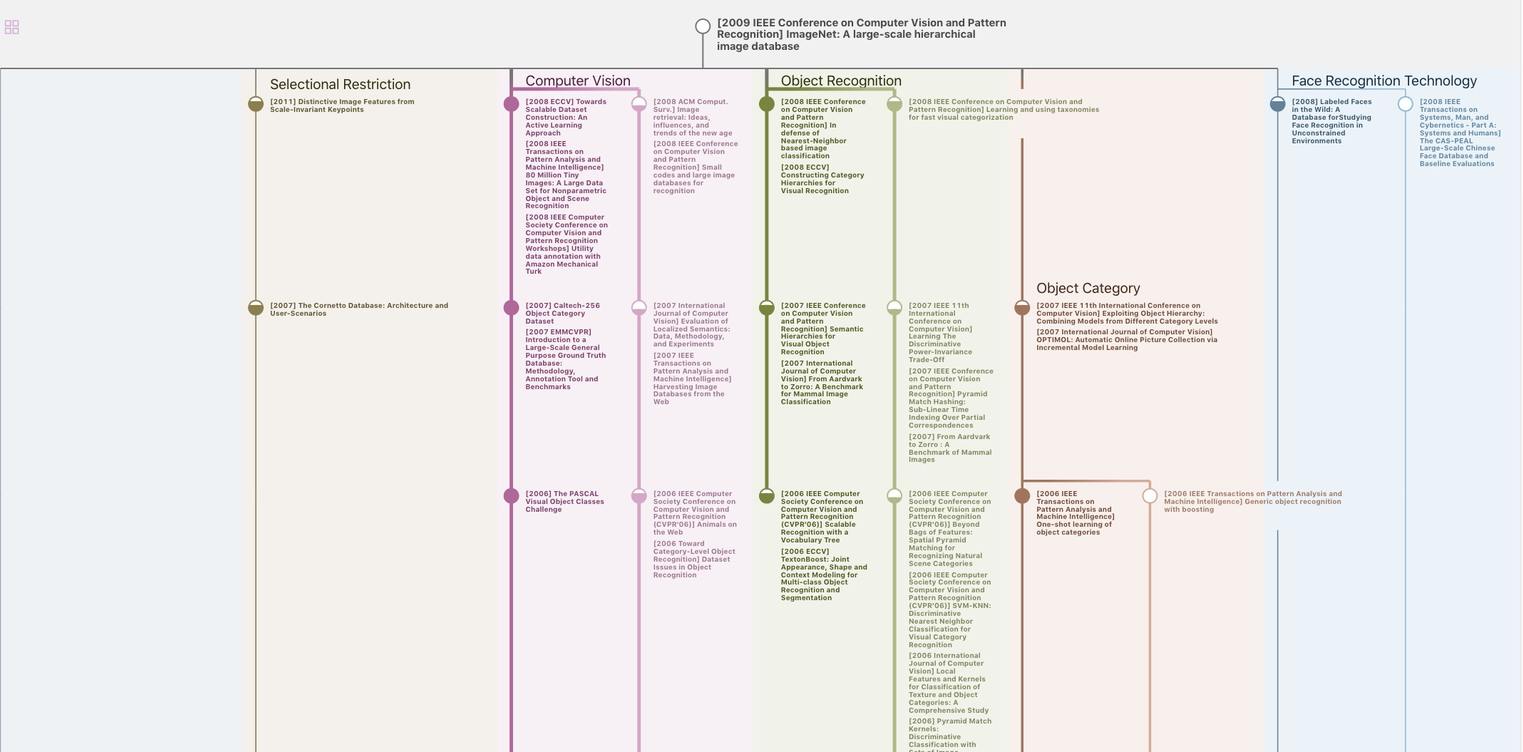
生成溯源树,研究论文发展脉络
Chat Paper
正在生成论文摘要