Solving imbalanced learning with outlier detection and features reduction
Machine Learning(2023)
摘要
A critical problem for several real world applications is class imbalance. Indeed, in contexts like fraud detection or medical diagnostics, standard machine learning models fail because they are designed to handle balanced class distributions. Existing solutions typically increase the rare class instances by generating synthetic records to achieve a balanced class distribution. However, these procedures generate not plausible data and tend to create unnecessary noise. We propose a change of perspective where instead of relying on resampling techniques, we depend on unsupervised features engineering approaches to represent records with a combination of features that will help the classifier capturing the differences among classes, even in presence of imbalanced data. Thus, we combine a large array of outlier detection, features projection, and features selection approaches to augment the expressiveness of the dataset population. We show the effectiveness of our proposal in a deep and wide set of benchmarking experiments as well as in real case studies.
更多查看译文
关键词
Imbalanced data learning,Outlier detection,Features reduction,Features selection,Classification framework
AI 理解论文
溯源树
样例
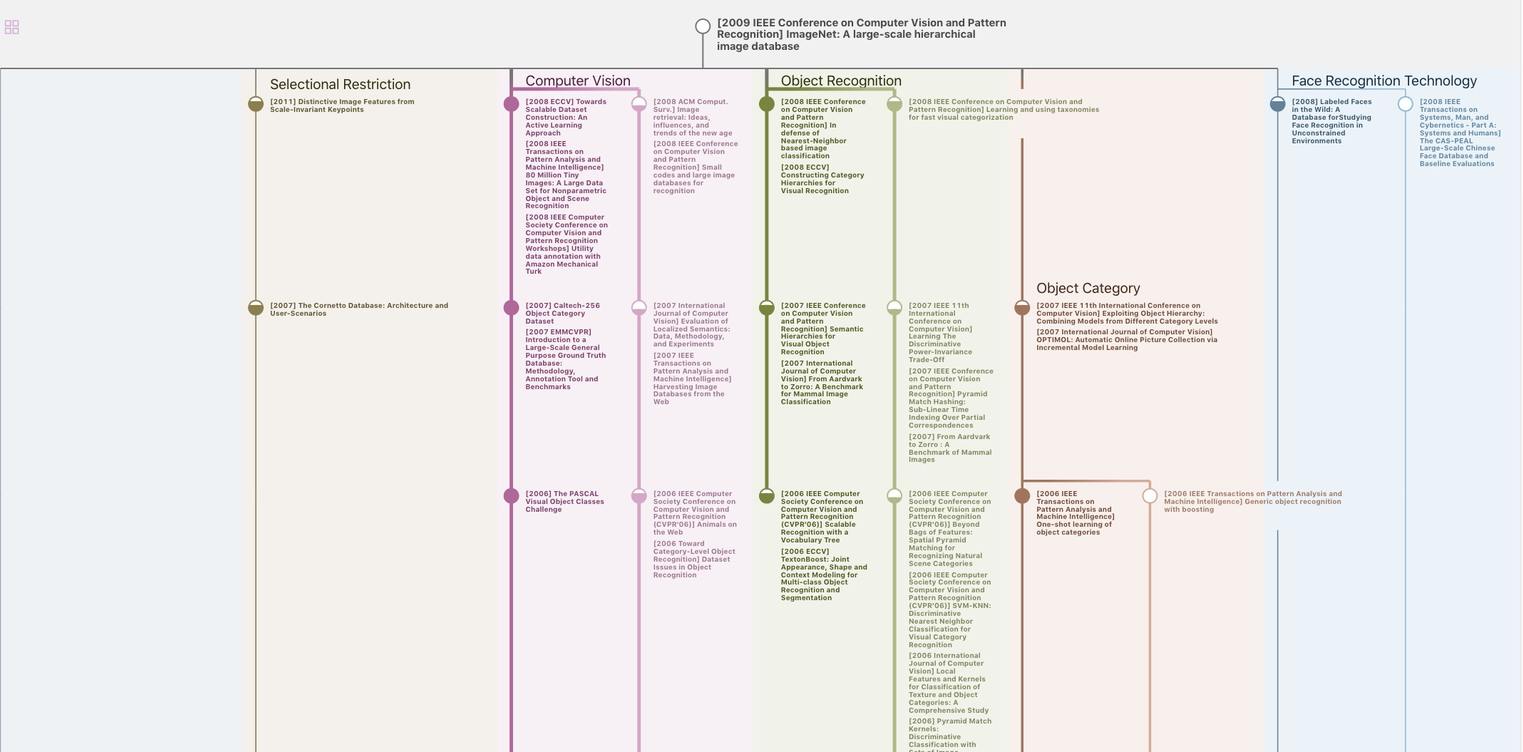
生成溯源树,研究论文发展脉络
Chat Paper
正在生成论文摘要