PaperMage: A Unified Toolkit for Processing, Representing, and Manipulating Visually-Rich Scientific Documents.
EMNLP 2023(2023)
摘要
Despite growing interest in applying natural language processing (NLP) and computer vision (CV) models to the scholarly domain, scientific documents remain challenging to work with. They’re often in difficult-to-use PDF formats, and the ecosystem of models to process them is fragmented and incomplete. We introduce PaperMage, an open-source Python toolkit for analyzing and processing visually-rich, structured scientific documents. PaperMage offers clean and intuitive abstractions for seamlessly representing and manipulating both textual and visual document elements. PaperMage achieves this by integrating disparate state-of-the-art NLP and CV models into a unified framework, and provides turn-key recipes for common scientific document processing use-cases. PaperMage has powered multiple research prototypes of AI applications over scientific documents, along with Semantic Scholar’s large-scale production system for processing millions of PDFs. GitHub: https://github.com/allenai/papermage
更多查看译文
AI 理解论文
溯源树
样例
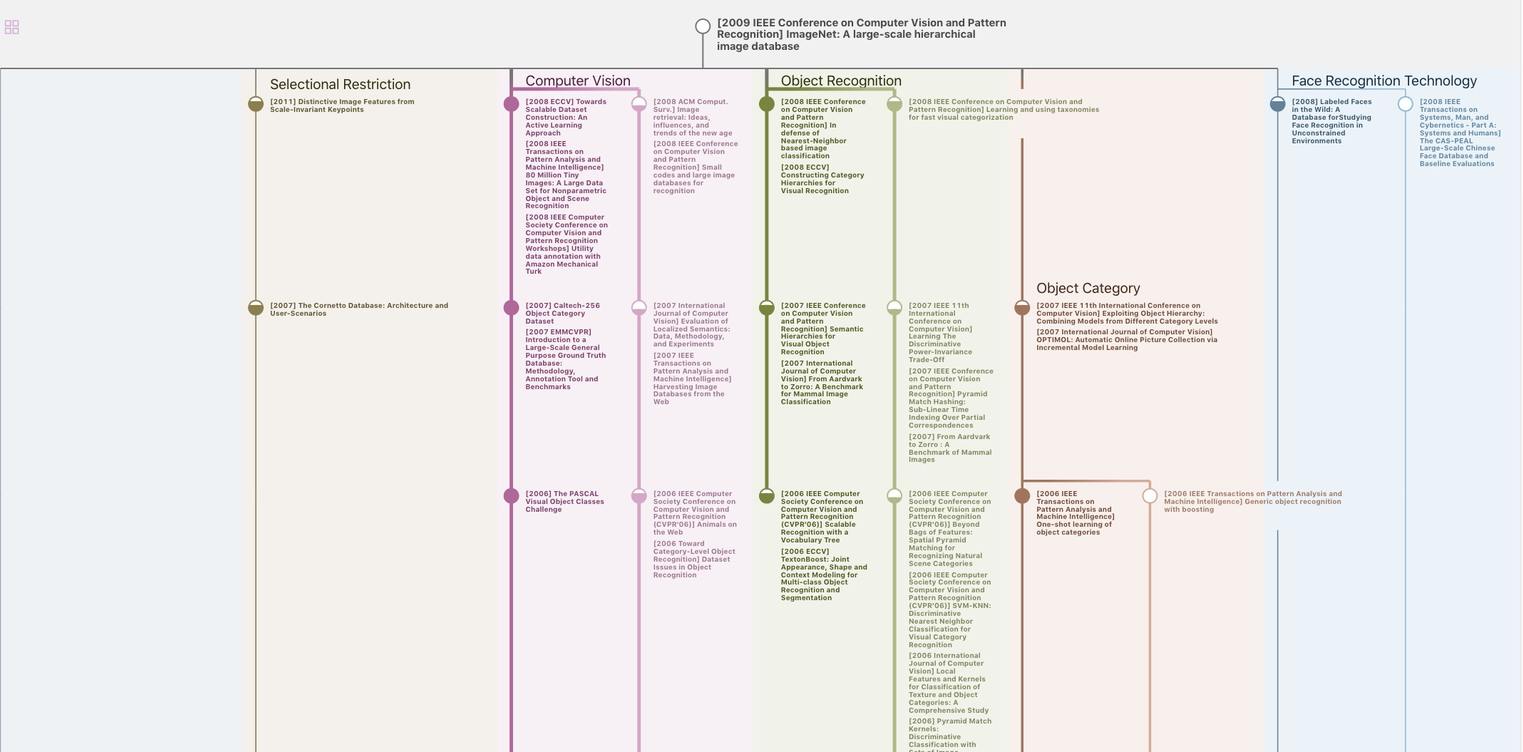
生成溯源树,研究论文发展脉络
Chat Paper
正在生成论文摘要