Retrospective: A Scalable Processing-in-Memory Accelerator for Parallel Graph Processing
arXiv (Cornell University)(2023)
摘要
Our ISCA 2015 paper provides a new programmable processing-in-memory (PIM) architecture and system design that can accelerate key data-intensive applications, with a focus on graph processing workloads. Our major idea was to completely rethink the system, including the programming model, data partitioning mechanisms, system support, instruction set architecture, along with near-memory execution units and their communication architecture, such that an important workload can be accelerated at a maximum level using a distributed system of well-connected near-memory accelerators. We built our accelerator system, Tesseract, using 3D-stacked memories with logic layers, where each logic layer contains general-purpose processing cores and cores communicate with each other using a message-passing programming model. Cores could be specialized for graph processing (or any other application to be accelerated). To our knowledge, our paper was the first to completely design a near-memory accelerator system from scratch such that it is both generally programmable and specifically customizable to accelerate important applications, with a case study on major graph processing workloads. Ensuing work in academia and industry showed that similar approaches to system design can greatly benefit both graph processing workloads and other applications, such as machine learning, for which ideas from Tesseract seem to have been influential. This short retrospective provides a brief analysis of our ISCA 2015 paper and its impact. We briefly describe the major ideas and contributions of the work, discuss later works that built on it or were influenced by it, and make some educated guesses on what the future may bring on PIM and accelerator systems.
更多查看译文
关键词
graph,parallel
AI 理解论文
溯源树
样例
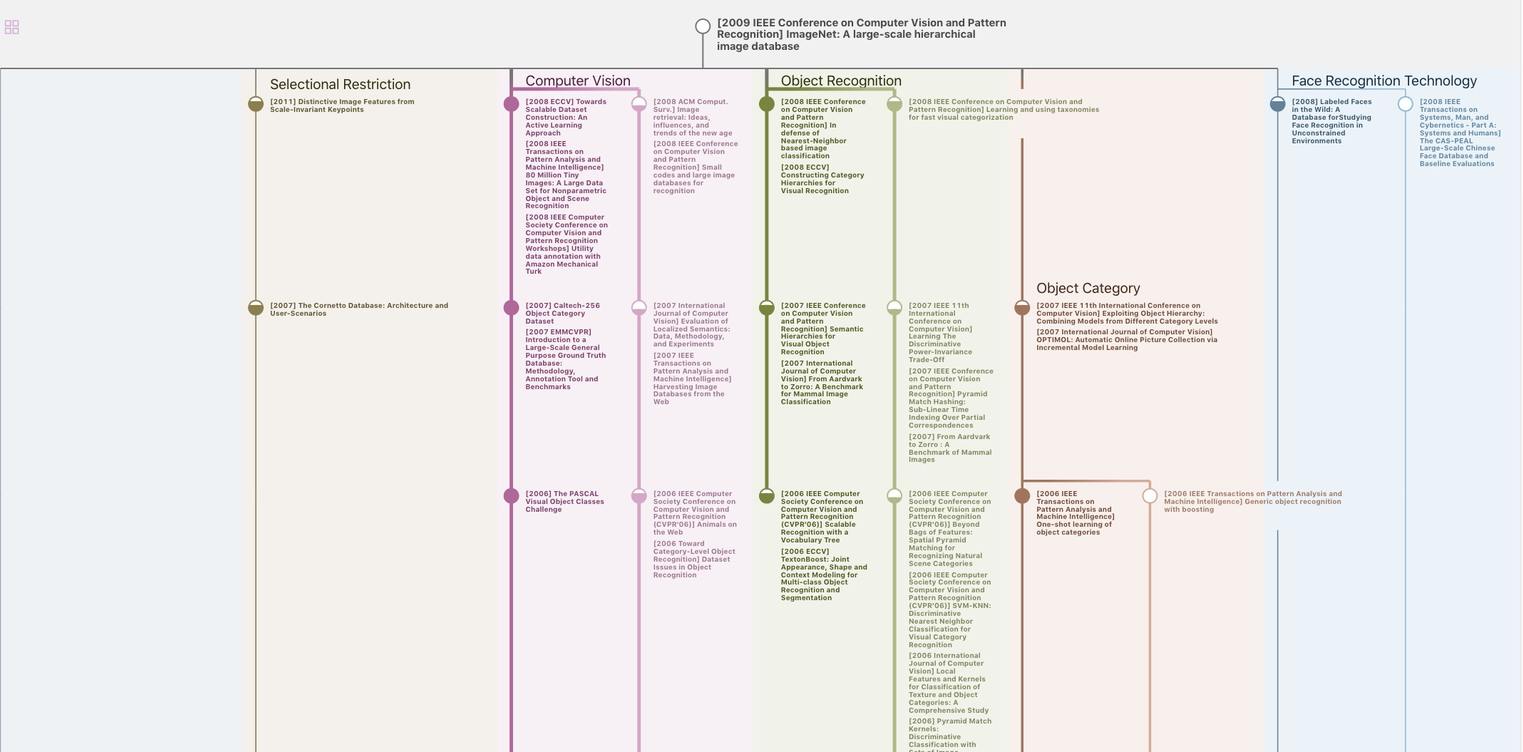
生成溯源树,研究论文发展脉络
Chat Paper
正在生成论文摘要