Xtune: An XAI-Based Hyperparameter Tuning Method for Time-series Forecasting Using Deep Learning
Research Square (Research Square)(2023)
摘要
Abstract Given a time-series dataset and deep learning based time-series forecasting method, how can we determine the optimal hyperparameter value efficiently? How can we determine this value without running the method with a large number of candidate values? In this study, we present \textit{Xtune}, an efficient and novel hyperparameter tuning method, utilizing explainable AI, for a time-series forecasting using deep learning. Our proposed method has the following properties: (a) It is effective: it determines the optimal hyperparameter value of deep learning based time-series forecasting methods. (b) It is efficient: it does not need to run the method on various hyperparameter values. (c) It is applicable: it can be used to tune the hyperprameter tuning for the anomaly detection method utilizing deep learning-based time-series forecasting. Extensive experiments on real datasets and time-series forecasting methods demonstrate that \textit{Xtune} does indeed determine the optimal hyperparameter value efficiently, and consistently outperforms the existing methods in terms of execution speed.
更多查看译文
关键词
deep learning,xai-based,time-series
AI 理解论文
溯源树
样例
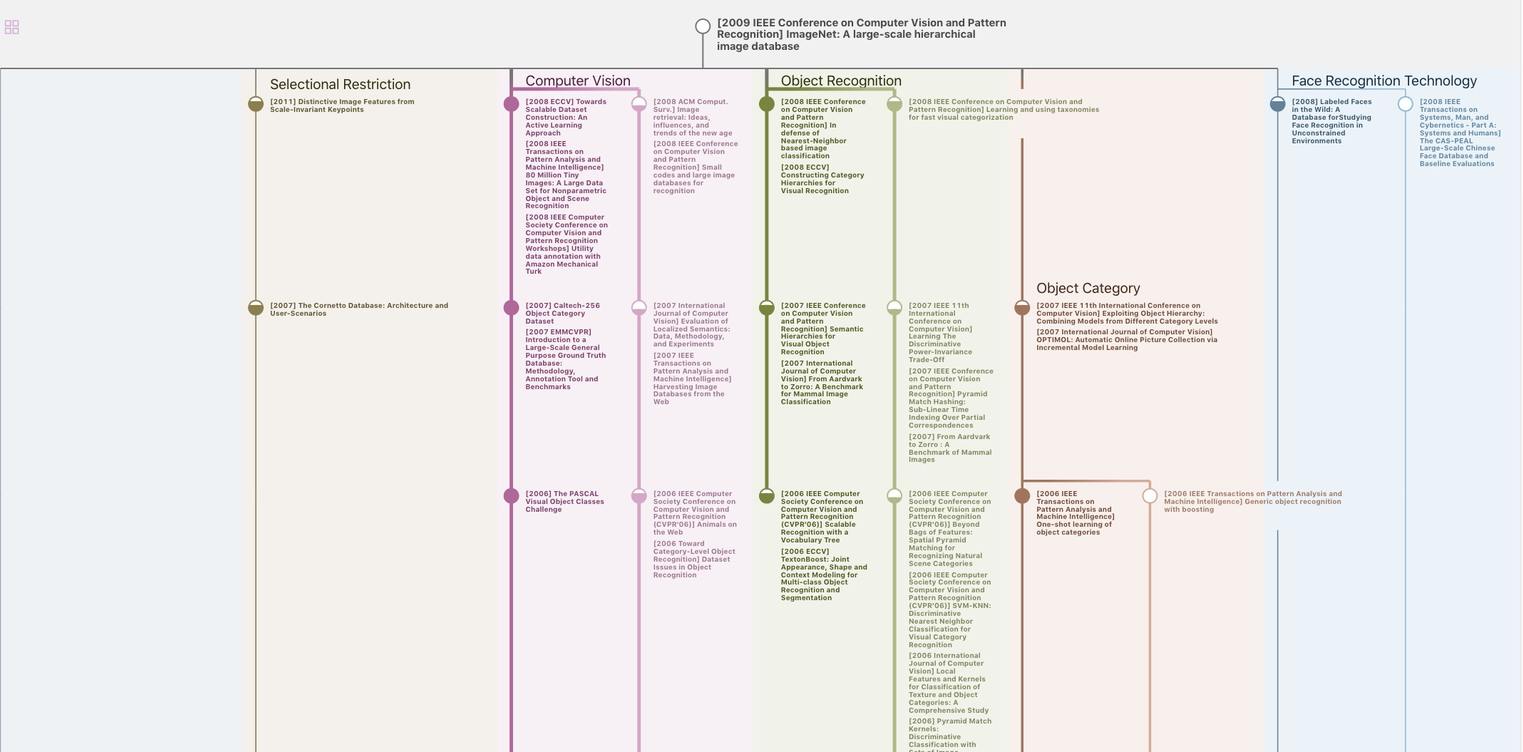
生成溯源树,研究论文发展脉络
Chat Paper
正在生成论文摘要