Resting state electroencephalographic brain activity in neonates can predict age and is indicative of neurodevelopmental outcome
Clinical Neurophysiology(2024)
摘要
Objective
Electroencephalography (EEG) can be used to estimate neonates’ biological brain age. Discrepancies between postmenstrual age and brain age, termed the brain age gap, can potentially quantify maturational deviation. Existing brain age EEG models are not well suited to clinical cot-side use for estimating neonates’ brain age gap due to their dependency on relatively large data and pre-processing requirements.
Methods
We trained a deep learning model on resting state EEG data from preterm neonates with normal neurodevelopmental Bayley Scale of Infant and Toddler Development (BSID) outcomes, using substantially reduced data requirements. We subsequently tested this model in two independent datasets from two clinical sites.
Results
In both test datasets, using only 20 min of resting-state EEG activity from a single channel, the model generated accurate age predictions: mean absolute error=1.03 weeks (p-value=0.0001) and 0.98 weeks (p-value=0.0001). In one test dataset, where 9-month follow-up BSID outcomes were available, the average neonatal brain age gap in the severe abnormal outcome group was significantly larger than that of the normal outcome group: difference in mean brain age gap=0.50 weeks (p-value=0.04).
Conclusions
These findings demonstrate that the deep learning model generalises to independent datasets from two clinical sites, and that the model’s brain age gap magnitudes differ between neonates with normal and severe abnormal follow-up neurodevelopmental outcomes.
Significance
The magnitude of neonates’ brain age gap, estimated using only 20 min of resting state EEG data from a single channel, can encode information of clinical neurodevelopmental value.
更多查看译文
关键词
Infant,deep learning,convolutional neural network,electroencephalography,Bayley Scale,brain age gap
AI 理解论文
溯源树
样例
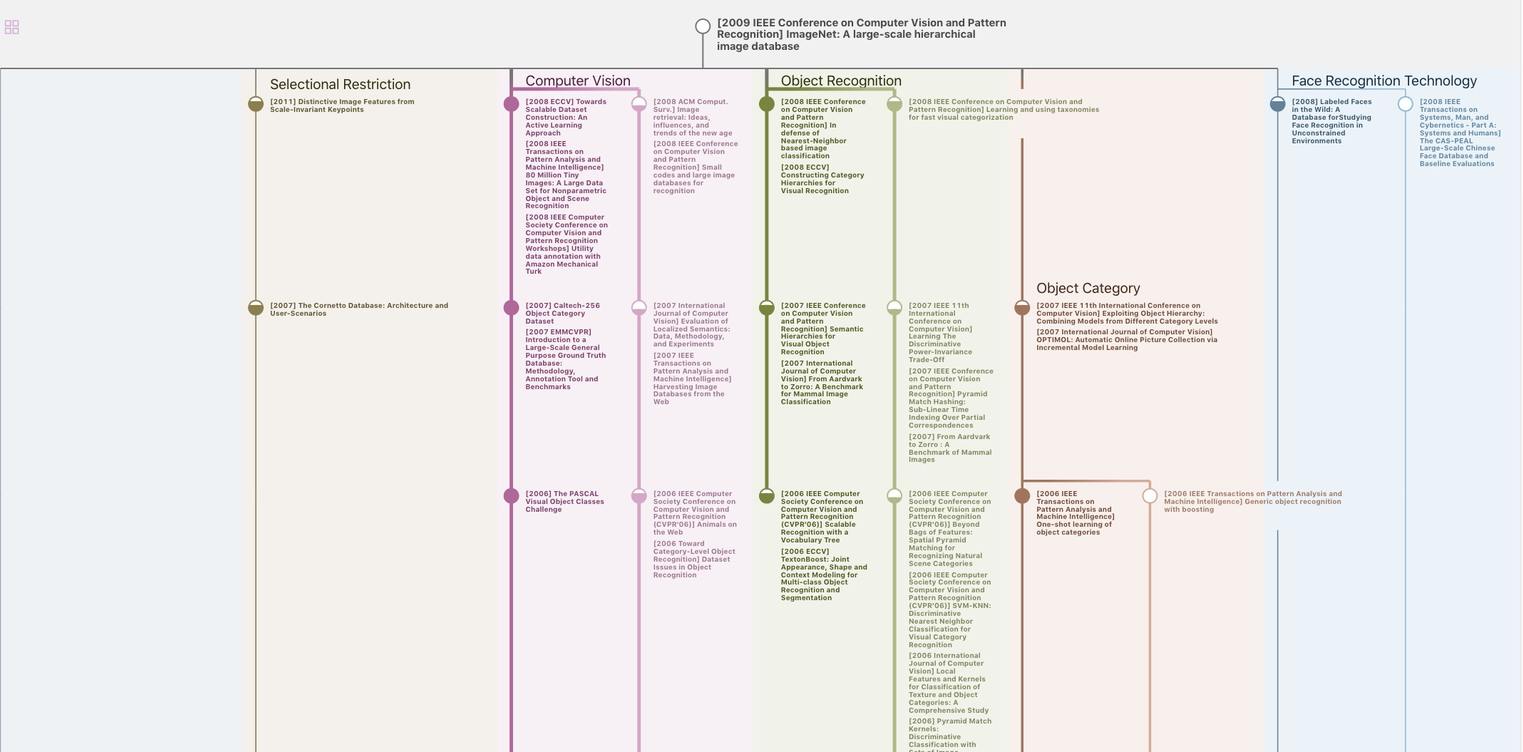
生成溯源树,研究论文发展脉络
Chat Paper
正在生成论文摘要