Privacy-preserving correlation of cross-organizational cyber threat intelligence with private graph intersections
COMPUTERS & SECURITY(2023)
摘要
Sharing cyber threat intelligence is important because it allows organizations to stay ahead of new and emerging threats, prevent downtime and improve their overall security posture. Information about known vulnerabilities and post-mortem analyses of successful attacks is instrumental to make tactical decisions and implement adequate countermeasures. However, organizations are hesitant or cautious to share their locally collected cyber threat intelligence with third parties because of possible damage to the organization's reputation, legal or liability concerns, or the risk that the information is used against them.In order to promote a collaborative cybersecurity environment that accommodates the varying confidentiality requirements of both threat intelligence producers and consumers, we introduce and assess a viable solution for preserving privacy while sharing and analyzing sensitive or confidential data. This solution is designed to work seamlessly with modern cyber threat intelligence platforms. Furthermore, we examine the security implications and computational impact associated with these techniques, enabling the analysis of correlations between threat events in a manner that respects confidentiality and extends across multiple organizations involved in information sharing.
更多查看译文
关键词
Threat intelligence sharing,Security,Privacy,Polyglot persistence and analysis,Private graph intersection
AI 理解论文
溯源树
样例
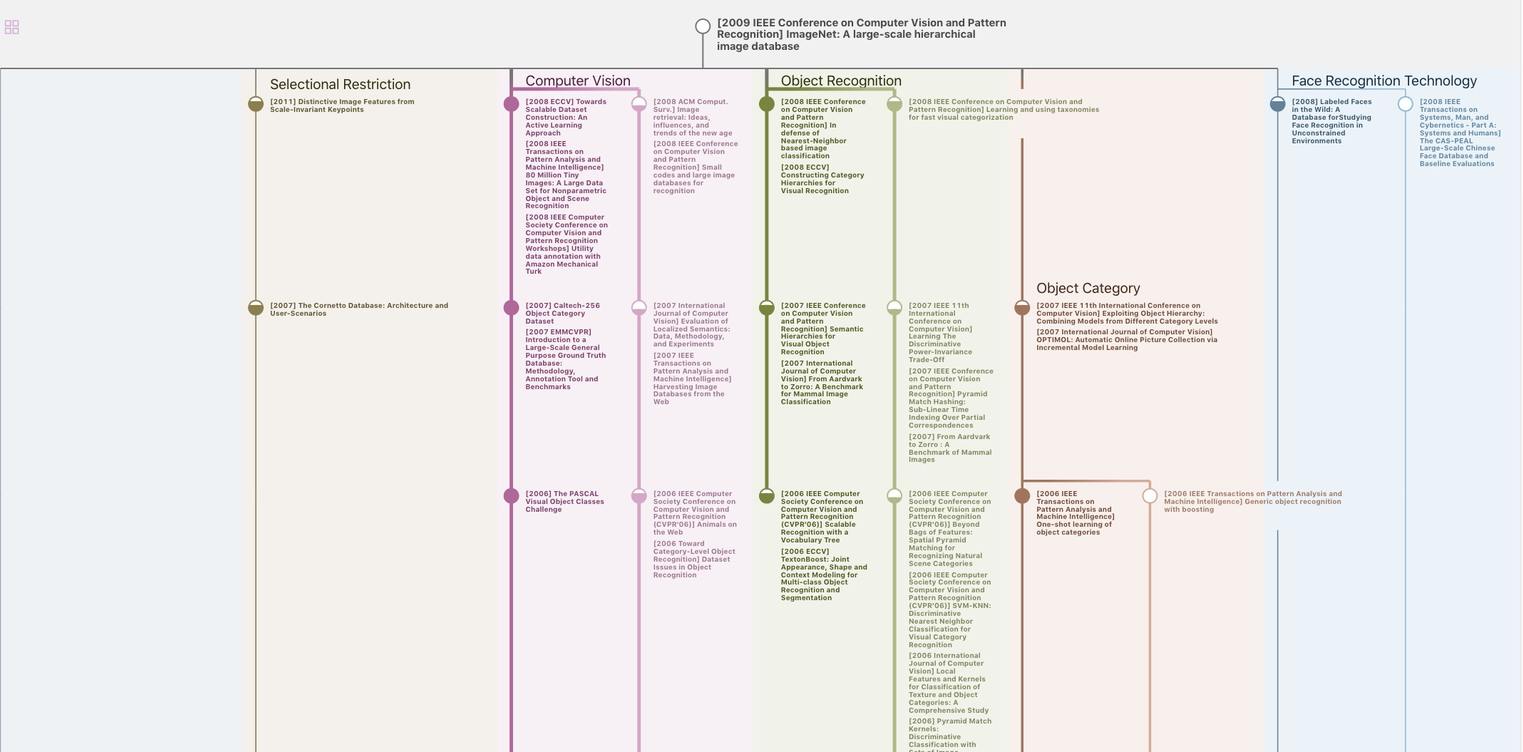
生成溯源树,研究论文发展脉络
Chat Paper
正在生成论文摘要