Multi-Hop Knowledge Graph Reasoning in Few-Shot Scenarios
IEEE TRANSACTIONS ON KNOWLEDGE AND DATA ENGINEERING(2024)
摘要
Reinforcement learning (RL)-based multi-hop reasoning has become an interpretable way for knowledge graph reasoning owing to its persuasive explanations for the predicted results, but the reasoning performance of these methods drops significantly over few-shot relations (only contain few triplets). To address this problem, recent studies introduce meta-learning into RL-based reasoning methods. However, the performance of these studies is still limited due to the following points: (1) the overall reasoning accuracy is impaired due to the low reasoning accuracies over some hard relations; (2) the reasoning process becomes laborious and ineffective owing to the existence of noisy data; (3) the generalizability is negatively affected due to the lack of knowledge-sharing. To tackle these challenges, we propose a novel model HMLS consisting of two modules HHML (H ierarchical H ardness-aware M eta-reinforcement L earning) and HHS (H ierarchical H ardness-aware S ampling). Specifically, HHML contains the following two components: (1) a hardness-aware RL conducts multi-hop reasoning by training hardness-aware batches and reducing noise; (2) a knowledge-sharing meta-learning adapts to few-shot relations by exploiting common features in the hierarchical relation structure. The other module HHS generates hardness-aware batches from relation and relation-cluster levels. The experimental results demonstrate that this work notably outperforms the state-of-the-art approaches in few-shot scenarios.
更多查看译文
关键词
Few-shot relations,hard sample mining,meta-reinforcement learning,multi-hop knowledge graph reasoning
AI 理解论文
溯源树
样例
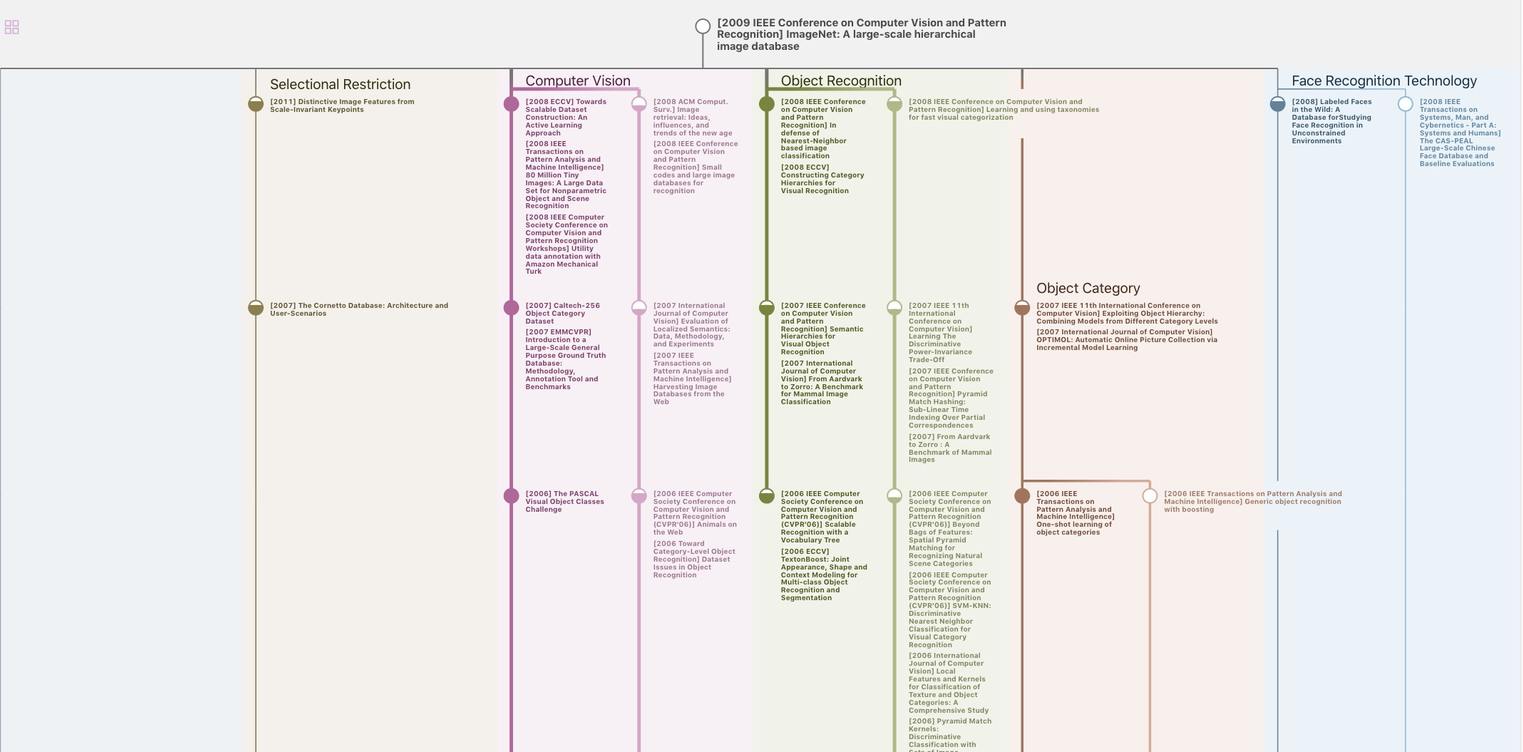
生成溯源树,研究论文发展脉络
Chat Paper
正在生成论文摘要