Penetrative AI: Making LLMs Comprehend the Physical World
arXiv (Cornell University)(2023)
摘要
Recent developments in Large Language Models (LLMs) have demonstrated their remarkable capabilities across a range of tasks. Questions, however, persist about the nature of LLMs and their potential to integrate common-sense human knowledge when performing tasks involving information about the real physical world. This paper delves into these questions by exploring how LLMs can be extended to interact with and reason about the physical world through IoT sensors and actuators, a concept that we term "\textit{Penetrative AI}". The paper explores such an extension at two levels of LLMs' ability to penetrate into the physical world via the processing of sensory signals. Our preliminary findings indicate that LLMs, with ChatGPT being the representative example in our exploration, have considerable and unique proficiency in employing the knowledge they learned during training for interpreting IoT sensor data and reasoning over them about tasks in the physical realm. Not only this opens up new applications for LLMs beyond traditional text-based tasks, but also enables new ways of incorporating human knowledge in cyber-physical systems.
更多查看译文
关键词
penetrative ai,llms,physical world
AI 理解论文
溯源树
样例
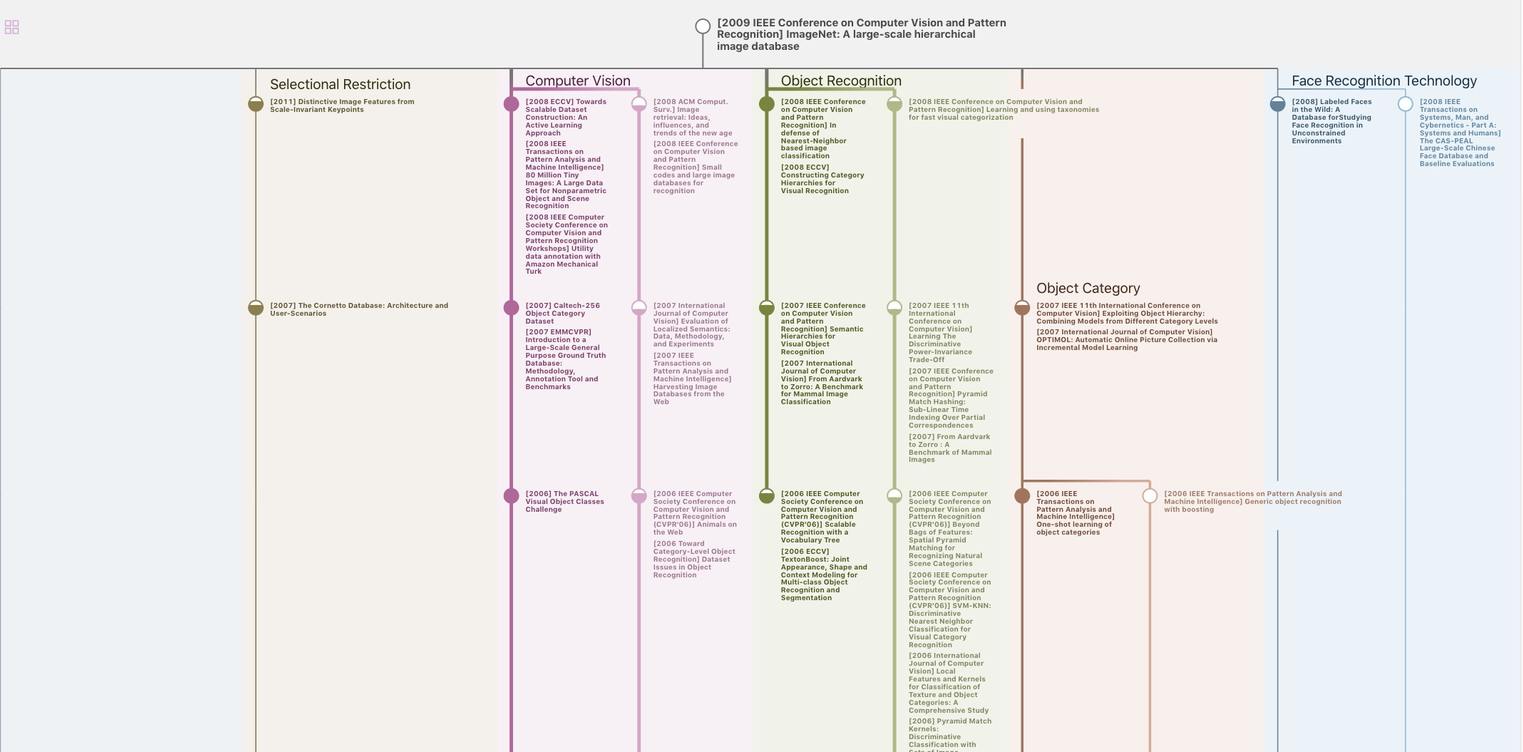
生成溯源树,研究论文发展脉络
Chat Paper
正在生成论文摘要