Multi-patch integrated learning dehazing network for small-scale dataset
JOURNAL OF ELECTRONIC IMAGING(2023)
摘要
To further improve the feature representation ability of haze removal model on small-scale datasets, we propose a three-hierarchical encoder-decoder integrating learning architecture. Unlike increasing the depth of network to improve the performance, we use three sets of transfer learning encoders to aggregate features on multiple patches. This strategy makes full use of deep and shallow features from different spatial parts of original hazy images. These different spatial parts are used to jointly optimize non-homogeneous hazy images with different scene depths and degradation degrees. We propose an integrated learning decoder, in which the internal integrated learning module decodes by gradually infusing the deep and shallow features of the identical patch. We fuse all encoded features at each stage for original resolution decoder. We report the quantitative and visual results of eight advanced haze removal methods (2019 to 2021). We compare these methods on five real-world and synthetic hazy datasets, including homogeneous and non-homogeneous degraded datasets. We present the results of the ablation study for key components. The generalization of our method is significantly better than other methods on real hazy scenes. We report improvements in semantic segmentation performance. This result could be used for autonomous driving, automated guided vehicle scheduling in closed factory area, etc. (c) 2023SPIE and IS&T
更多查看译文
关键词
multi-patch,hierarchical architecture,transfer learning,integrated learning
AI 理解论文
溯源树
样例
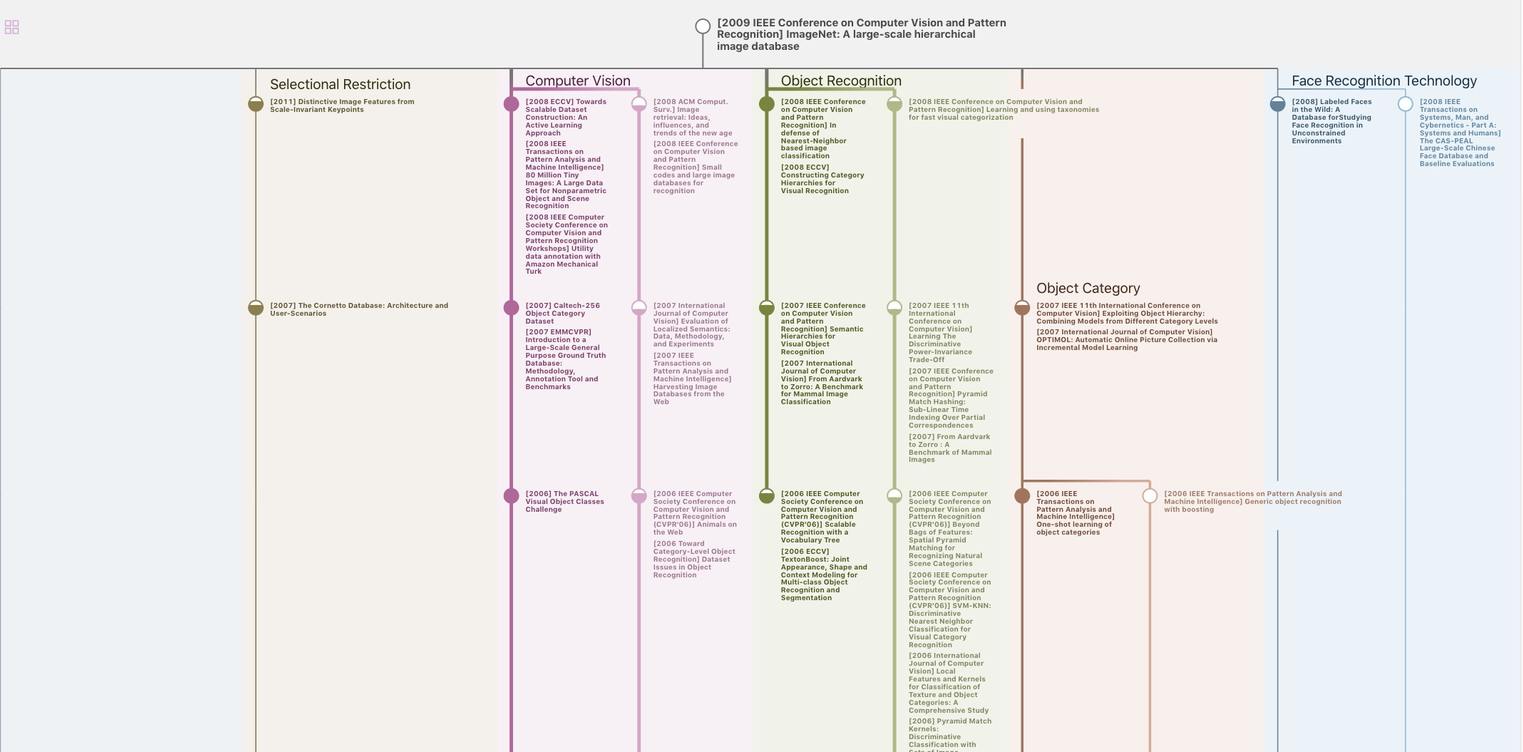
生成溯源树,研究论文发展脉络
Chat Paper
正在生成论文摘要