Likelihood Annealing: Fast Calibrated Uncertainty for Regression
arXiv (Cornell University)(2023)
摘要
Recent advances in deep learning have shown that uncertainty estimation is becoming increasingly important in applications such as medical imaging, natural language processing, and autonomous systems. However, accurately quantifying uncertainty remains a challenging problem, especially in regression tasks where the output space is continuous. Deep learning approaches that allow uncertainty estimation for regression problems often converge slowly and yield poorly calibrated uncertainty estimates that can not be effectively used for quantification. Recently proposed post hoc calibration techniques are seldom applicable to regression problems and often add overhead to an already slow model training phase. This work presents a fast calibrated uncertainty estimation method for regression tasks called Likelihood Annealing, that consistently improves the convergence of deep regression models and yields calibrated uncertainty without any post hoc calibration phase. Unlike previous methods for calibrated uncertainty in regression that focus only on low-dimensional regression problems, our method works well on a broad spectrum of regression problems, including high-dimensional regression.Our empirical analysis shows that our approach is generalizable to various network architectures, including multilayer perceptrons, 1D/2D convolutional networks, and graph neural networks, on five vastly diverse tasks, i.e., chaotic particle trajectory denoising, physical property prediction of molecules using 3D atomistic representation, natural image super-resolution, and medical image translation using MRI.
更多查看译文
关键词
likelihood annealing,calibrated uncertainty,regression
AI 理解论文
溯源树
样例
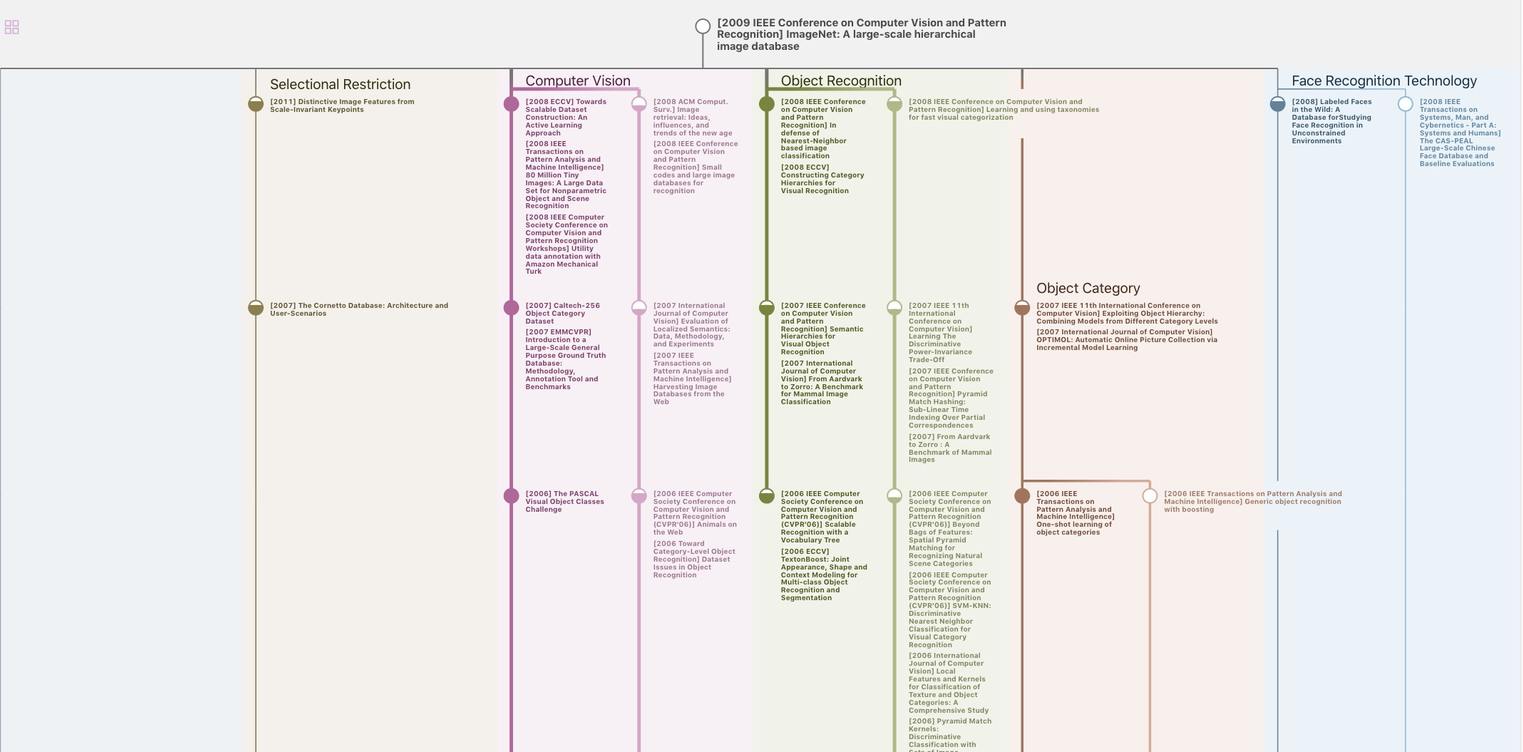
生成溯源树,研究论文发展脉络
Chat Paper
正在生成论文摘要