Metadata and Image Features Co-Aware Semi-Supervised Vertical Federated Learning With Attention Mechanism
IEEE TRANSACTIONS ON VEHICULAR TECHNOLOGY(2024)
摘要
Recently, vertical federated learning (VFL) has gained more attention due to its ability to combine various valuable features for better model performance without violating privacy. However, the development of VFL still faces three challenges: 1) insufficient aligned samples, 2) monotone data modality, and 3) simple concatenation-based collaborative training strategy. In this article, we construct a metadata and image features co-aware VFL model for smart healthcare, from which users can obtain convenient and timely online auxiliary diagnostic services. Furthermore, we propose a metadata and image features co-aware semi-supervised VFL scheme with attention mechanism, which aims to jointly train the VFL models by utilizing both metadata and image data from the entire sample space. Specifically, a generative-adversarial method is designed to train the generator to estimate the missing features, so as to expand the training set and improve the accuracy of model. Moreover, using semi-supervised clustering to forecast pseudo labels for the missing part of the active party, we further boost the performance of the VFL model by increasing the proportion of labeled samples. Meanwhile, inspired by the attention mechanism, we establish an entirely novel VFL training mode that emphasizes the underlying effect of metadata features on image features, which makes metadata features highlight crucial areas in the image features to improve the training effect. Finally, the experimental results confirm that our proposed scheme achieves higher accuracy compared with other related works in the VFL scenario.
更多查看译文
关键词
Vertical federated learning,semi-supervised learning,attention mechanism,bimodality fusion
AI 理解论文
溯源树
样例
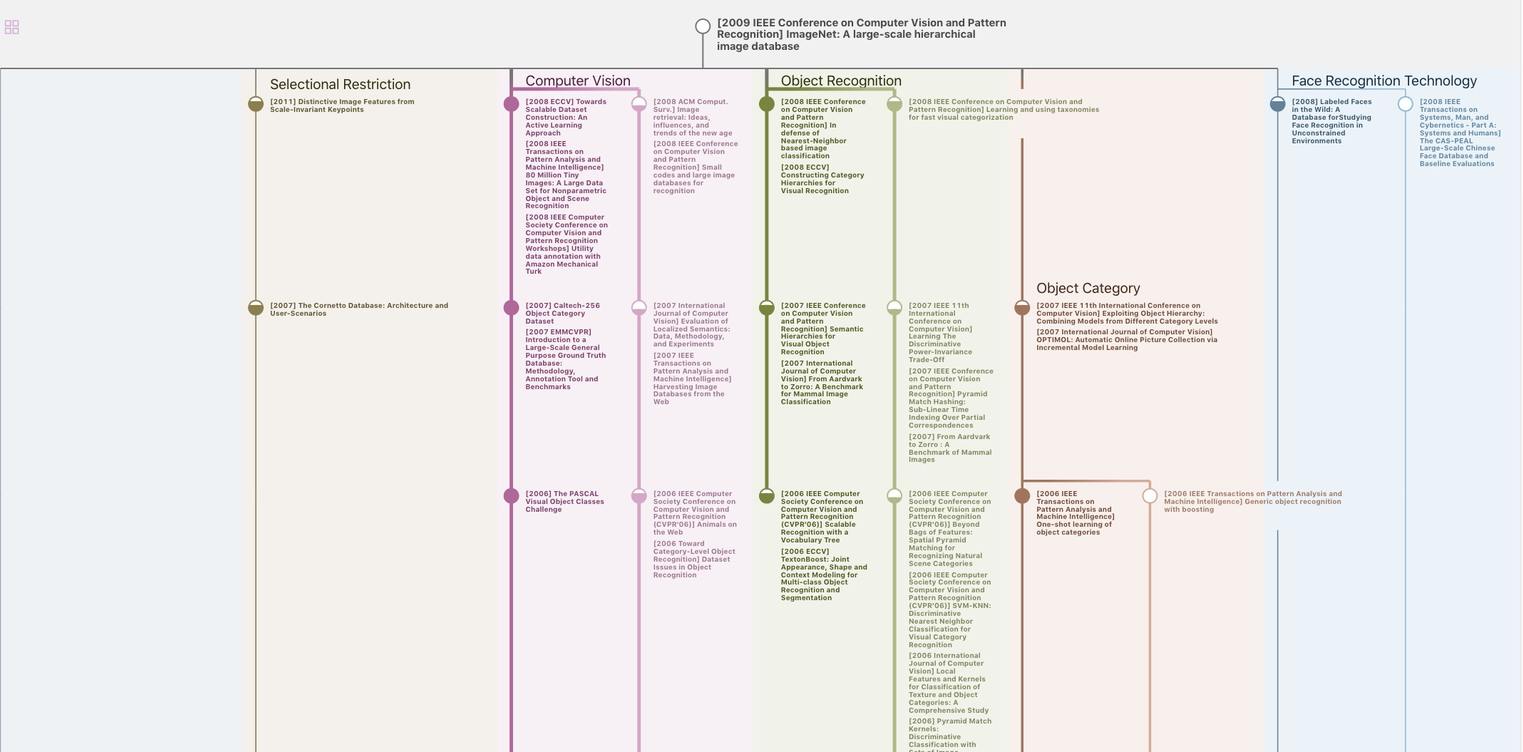
生成溯源树,研究论文发展脉络
Chat Paper
正在生成论文摘要