I2D2: Inductive Knowledge Distillation with NeuroLogic and Self-Imitation
PROCEEDINGS OF THE 61ST ANNUAL MEETING OF THE ASSOCIATION FOR COMPUTATIONAL LINGUISTICS (ACL 2023): LONG PAPERS, VOL 1(2023)
摘要
Commonsense capabilities of pre-trained language models dramatically improve with scale, leading many to believe that scale is the only winning recipe. But is it? Here, we investigate an alternative that a priori seems impossible: can smaller language models (e.g., GPT-2) win over models that are orders of magnitude larger and better (e.g., GPT-3), if powered with novel commonsense distillation algorithms? The key intellectual challenge is to design a learning algorithm that achieves a competitive level of commonsense acquisition, without relying on the benefits of scale. In particular, we study generative models of commonsense knowledge, focusing on the task of generating generics, statements of commonsense facts about everyday concepts, e.g., birds can fly. We introduce I2D2, a novel commonsense distillation framework that loosely follows West et al. (2022)'s Symbolic Knowledge Distillation but breaks the dependence on the extreme-scale teacher model with two innovations: (1) the novel adaptation of NeuroLogic Decoding (Lu et al., 2021) to enhance the generation quality of the weak, off-the-shelf language models, and (2) self-imitation learning to iteratively learn from the model's own enhanced commonsense acquisition capabilities. Empirical results suggest that scale is not the only way, as novel algorithms can be a promising alternative. Moreover, our study leads to a new corpus of generics, Gen-A-tomic, that is the largest and highest-quality available to date.
更多查看译文
关键词
inductive knowledge distillation,neurologic,self-imitation
AI 理解论文
溯源树
样例
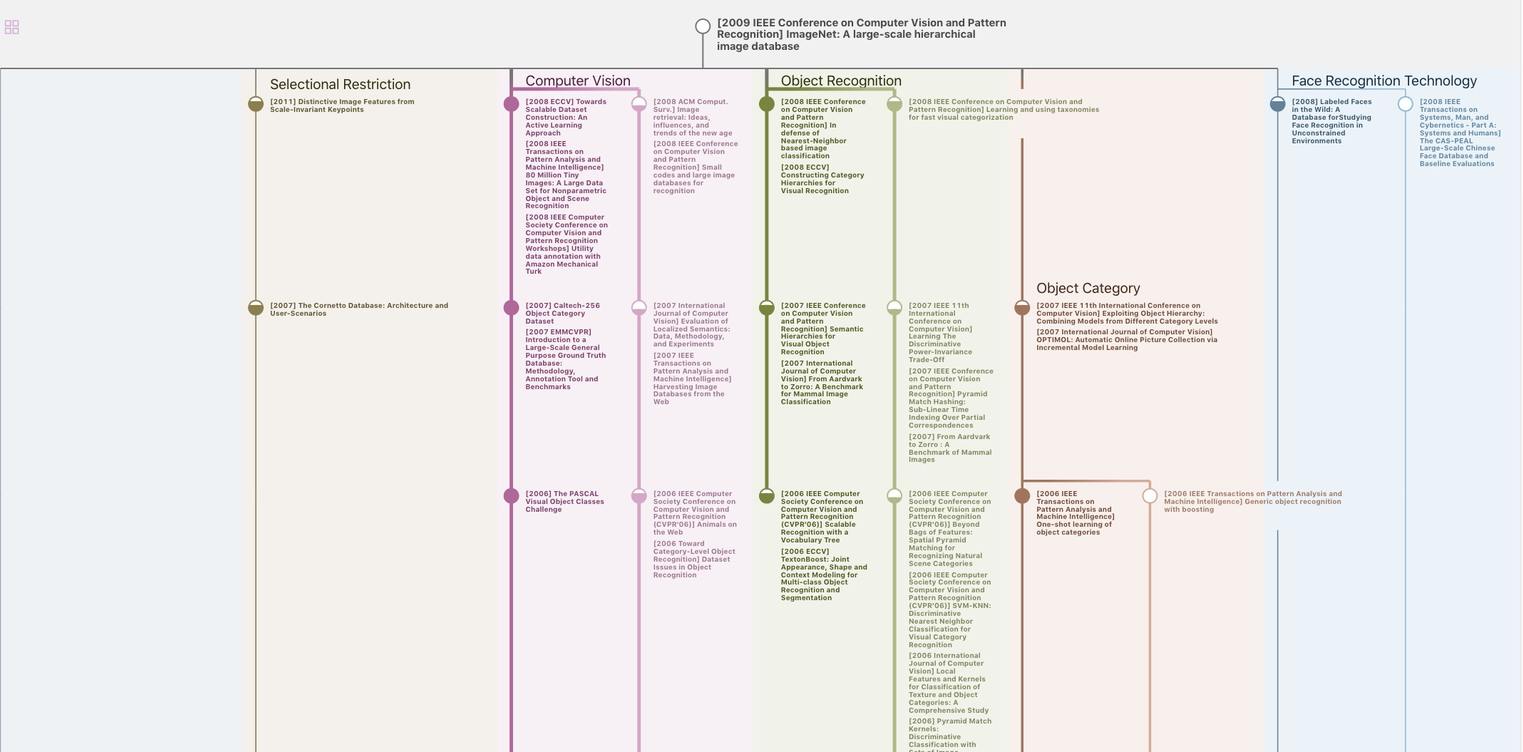
生成溯源树,研究论文发展脉络
Chat Paper
正在生成论文摘要