Leela Zero Score: a Study of a Score-based AlphaGo Zero
arXiv (Cornell University)(2022)
摘要
AlphaGo, AlphaGo Zero, and all of their derivatives can play with superhuman strength because they are able to predict the win-lose outcome with great accuracy. However, Go as a game is decided by a final score difference, and in final positions AlphaGo plays suboptimal moves: this is not surprising, since AlphaGo is completely unaware of the final score difference, all winning final positions being equivalent from the winrate perspective. This can be an issue, for instance when trying to learn the "best" move or to play with an initial handicap. Moreover, there is the theoretical quest of the "perfect game", that is, the minimax solution. Thus, a natural question arises: is it possible to train a successful Reinforcement Learning agent to predict score differences instead of winrates? No empirical or theoretical evidence can be found in the literature to support the folklore statement that "this does not work". In this paper we present Leela Zero Score, a software designed to support or disprove the "does not work" statement. Leela Zero Score is designed on the open-source solution known as Leela Zero, and is trained on a 9x9 board to predict score differences instead of winrates. We find that the training produces a rational player, and we analyze its style against a strong amateur human player, to find that it is prone to some mistakes when the outcome is close. We compare its strength against SAI, an AlphaGo Zero-like software working on the 9x9 board, and find that the training of Leela Zero Score has reached a premature convergence to a player weaker than SAI.
更多查看译文
关键词
score-based
AI 理解论文
溯源树
样例
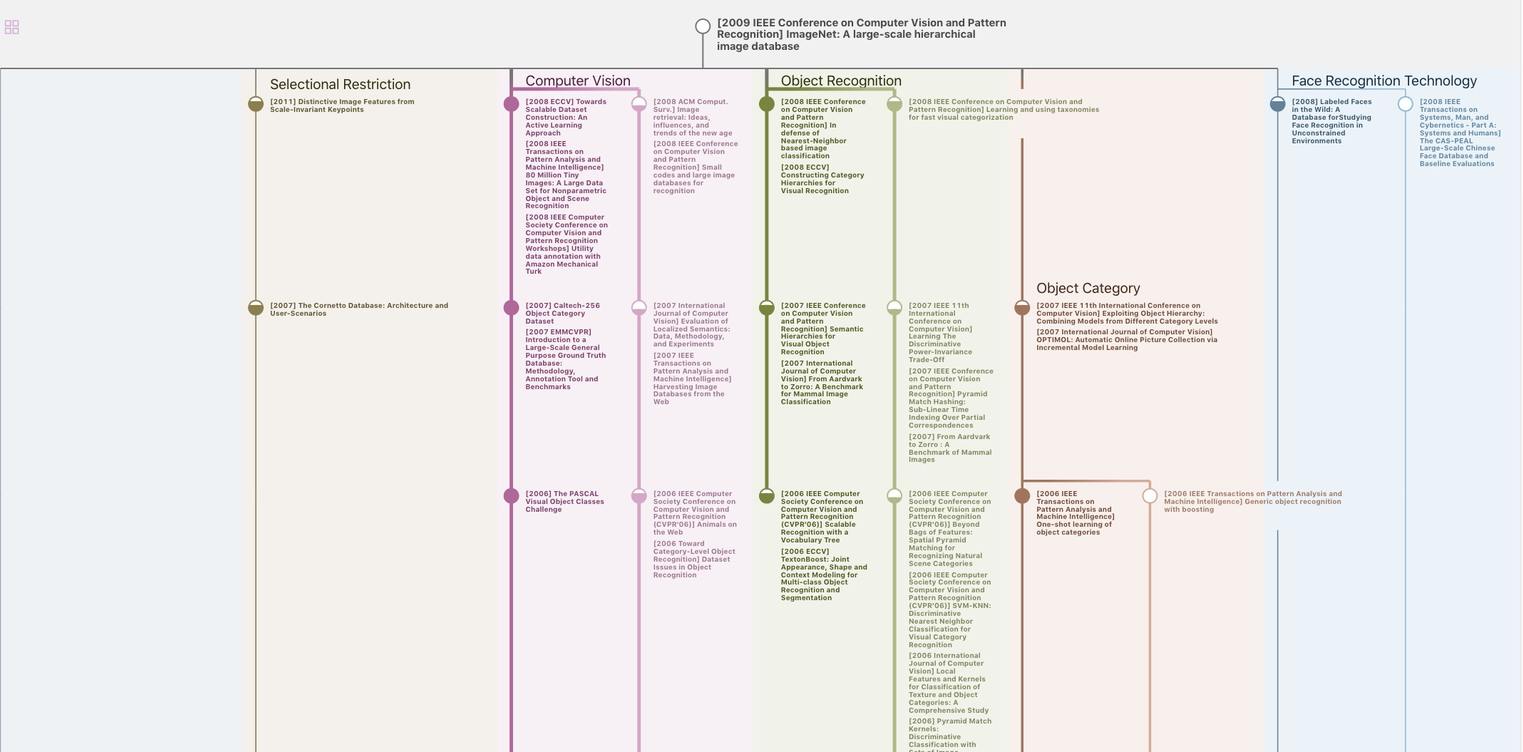
生成溯源树,研究论文发展脉络
Chat Paper
正在生成论文摘要