Problem-Driven Scenario Generation for Stochastic Programming Problems: A Survey
ALGORITHMS(2023)
摘要
Stochastic Programming is a powerful framework that addresses decision-making under uncertainties, which is a frequent occurrence in real-world problems. To effectively solve Stochastic Programming problems, scenario generation is one of the common practices that organizes realizations of stochastic processes with finite discrete distributions, which enables the use of mathematical programming models of the original problem. The quality of solutions is significantly influenced by the scenarios employed, necessitating a delicate balance between incorporating informative scenarios and preventing overfitting. Distributions-based scenario generation methodologies have been extensively studied over time, while a relatively recent concept of problem-driven scenario generation has emerged, aiming to incorporate the underlying problem's structure during the scenario generation process. This survey explores recent literature on problem-driven scenario generation algorithms and methodologies. The investigation aims to identify circumstances under which this approach is effective and efficient. The work provides a comprehensive categorization of existing literature, supplemented by illustrative examples. Additionally, the survey examines potential applications and discusses avenues for its integration with machine learning technologies. By shedding light on the effectiveness of problem-driven scenario generation and its potential for synergistic integration with machine learning, this survey contributes to enhanced decision-making strategies in the context of uncertainties.
更多查看译文
关键词
scenario generation,stochastic programming problems,problem-driven
AI 理解论文
溯源树
样例
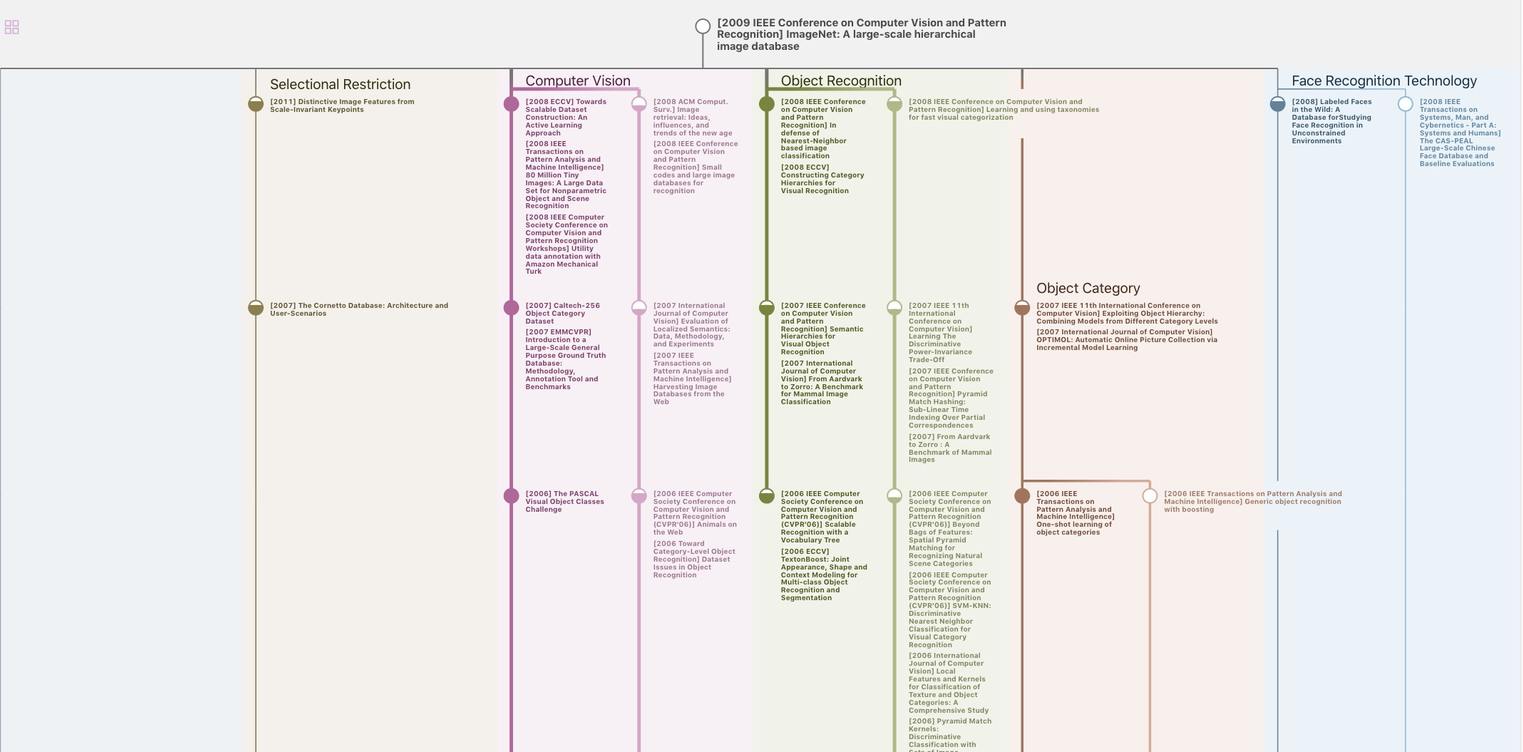
生成溯源树,研究论文发展脉络
Chat Paper
正在生成论文摘要