Multi-Label Quantification
ACM TRANSACTIONS ON KNOWLEDGE DISCOVERY FROM DATA(2024)
摘要
Quantification, variously called supervised prevalence estimation or learning to quantify, is the supervised learning task of generating predictors of the relative frequencies (a.k.a. prevalence values) of the classes of interest in unlabelled data samples. While many quantification methods have been proposed in the past for binary problems and, to a lesser extent, single-label multiclass problems, the multi-label setting (i.e., the scenario in which the classes of interest are not mutually exclusive) remains by and large unexplored. A straightforward solution to the multi-label quantification problem could simply consist of recasting the problem as a set of independent binary quantification problems. Such a solution is simple but naive, since the independence assumption upon which it rests is, in most cases, not satisfied. In these cases, knowing the relative frequency of one class could be of help in determining the prevalence of other related classes. We propose the first truly multi-label quantification methods, i.e., methods for inferring estimators of class prevalence values that strive to leverage the stochastic dependencies among the classes of interest in order to predict their relative frequencies more accurately. We show empirical evidence that natively multi-label solutions outperform the naive approaches by a large margin. The code to reproduce all our experiments is available online.
更多查看译文
关键词
Quantification,learning to quantify,supervised prevalence estimation,class prior estimation,multi-label quantification,multi-label learning
AI 理解论文
溯源树
样例
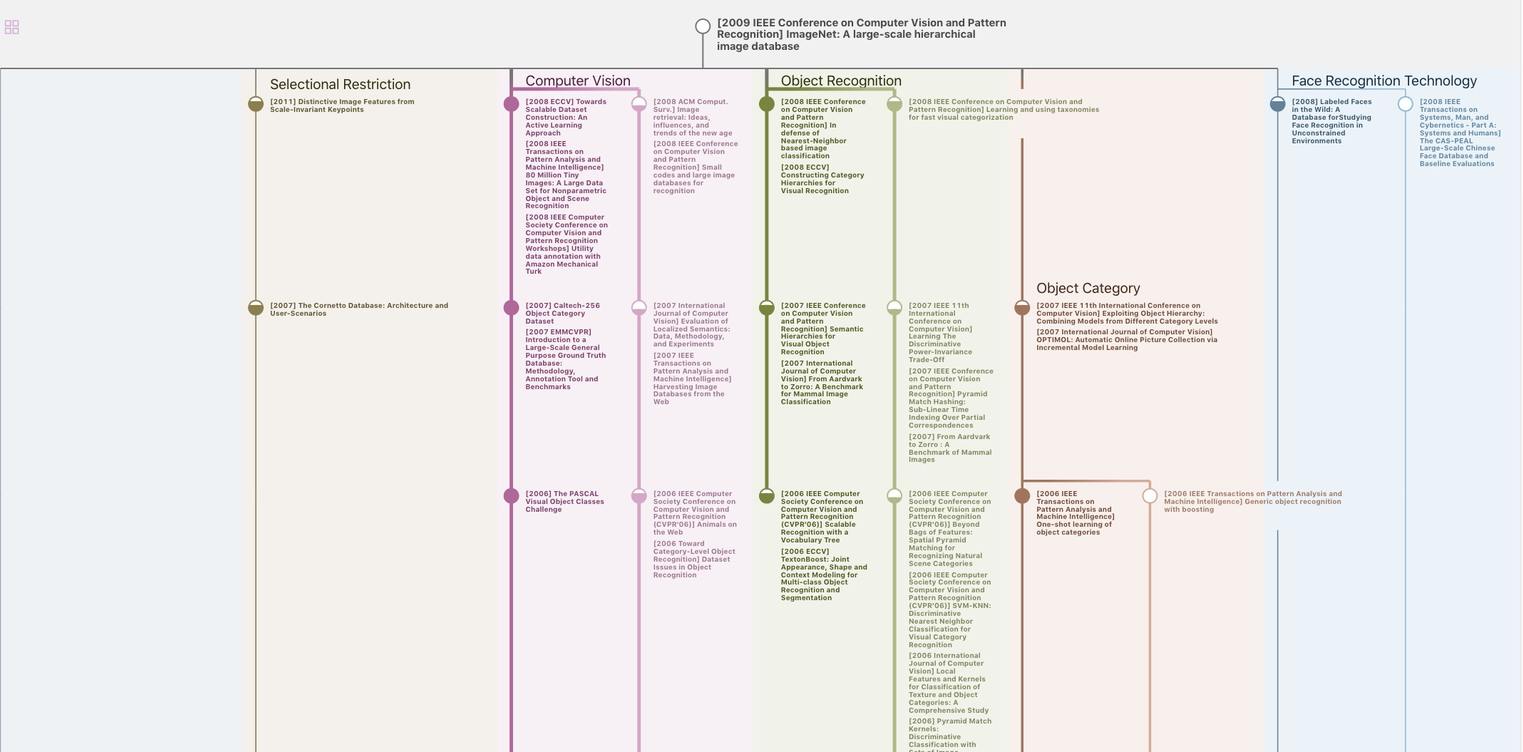
生成溯源树,研究论文发展脉络
Chat Paper
正在生成论文摘要