Outliers Robust Unsupervised Feature Selection for Structured Sparse Subspace
IEEE TRANSACTIONS ON KNOWLEDGE AND DATA ENGINEERING(2024)
摘要
Feature selection is one of the important topics of machine learning, and it has a wide range of applications in data preprocessing. At present, feature selection based on l(2,1)-norm regularization is a relatively mature method, but it is not enough to maximize the sparsity and parameter-tuning leads to increased costs. Later scholars found that the l(2,0)-norm constraint is more conductive to feature selection, but it is difficult to solve and lacks convergence guarantees. To address these problems, we creatively propose a novel Outliers Robust Unsupervised Feature Selection for structured sparse subspace (ORUFS), which utilizes l(2,0)-norm constraint to learn a structured sparse subspace and avoid tuning the regularization parameter. Moreover, by adding binary weights, outliers are directly eliminated and the robustness of model is improved. More importantly, a Re-Weighted (RW) algorithm is exploited to solve our l(p)-norm problem. For the NP-hard problem of l(2,0)-norm constraint, we develop an effective iterative optimization algorithm with strict convergence guarantees and closed-form solution. Subsequently, we provide theoretical analysis about convergence and computational complexity. Experimental results on real-world datasets illustrate that our method is superior to the state-of-the-art methods in clustering and anomaly detection tasks.
更多查看译文
关键词
l(20)-norm constraint,anomaly detection,outlier robust,unsupervised feature selection,clustering
AI 理解论文
溯源树
样例
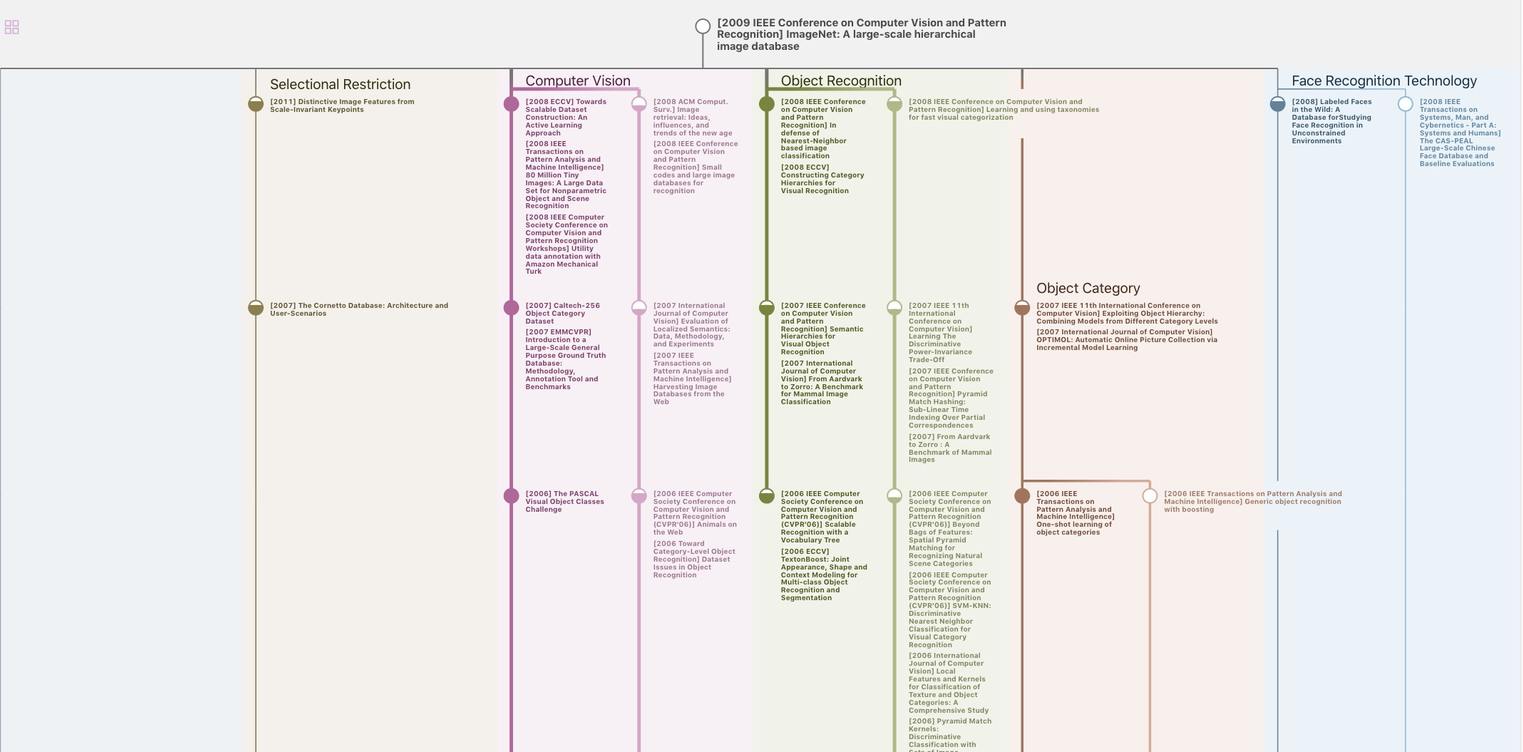
生成溯源树,研究论文发展脉络
Chat Paper
正在生成论文摘要