Completed Part Transformer for Person Re-Identification
IEEE TRANSACTIONS ON MULTIMEDIA(2024)
摘要
Recently, part information of pedestrian images has been demonstrated to be effective for person re-identification (ReID), but the part interaction is ignored when using Transformer to learn long-range dependencies. In this article, we propose a novel transformer network named Completed Part Transformer (CPT) for person ReID, where we design the part transformer layer to learn the completed part interaction. The part transformer layer includes the intra-part layer and the part-global layer, where they consider long-range dependencies from the aspects of the intra-part interaction and the part-global interaction, simultaneously. Furthermore, in order to overcome the limitation of fixed number of the patch tokens in the transformer layer, we propose the Adaptive Refined Tokens (ART) module to focus on learning the interaction between the informative patch tokens in the pedestrian image, which improves the discrimination of the pedestrian representation. Extensive experimental results on four person ReID datasets, i.e., MSMT17, Market1501, DukeMTMC-reID, and CUHK03, demonstrate that the proposed method achieves a new state-of-the-art performance, e.g., it achieves 68.0% mAP and 84.6% Rank-1 accuracy on MSMT17.
更多查看译文
关键词
Person ReID,transformer,adaptive refined tokens
AI 理解论文
溯源树
样例
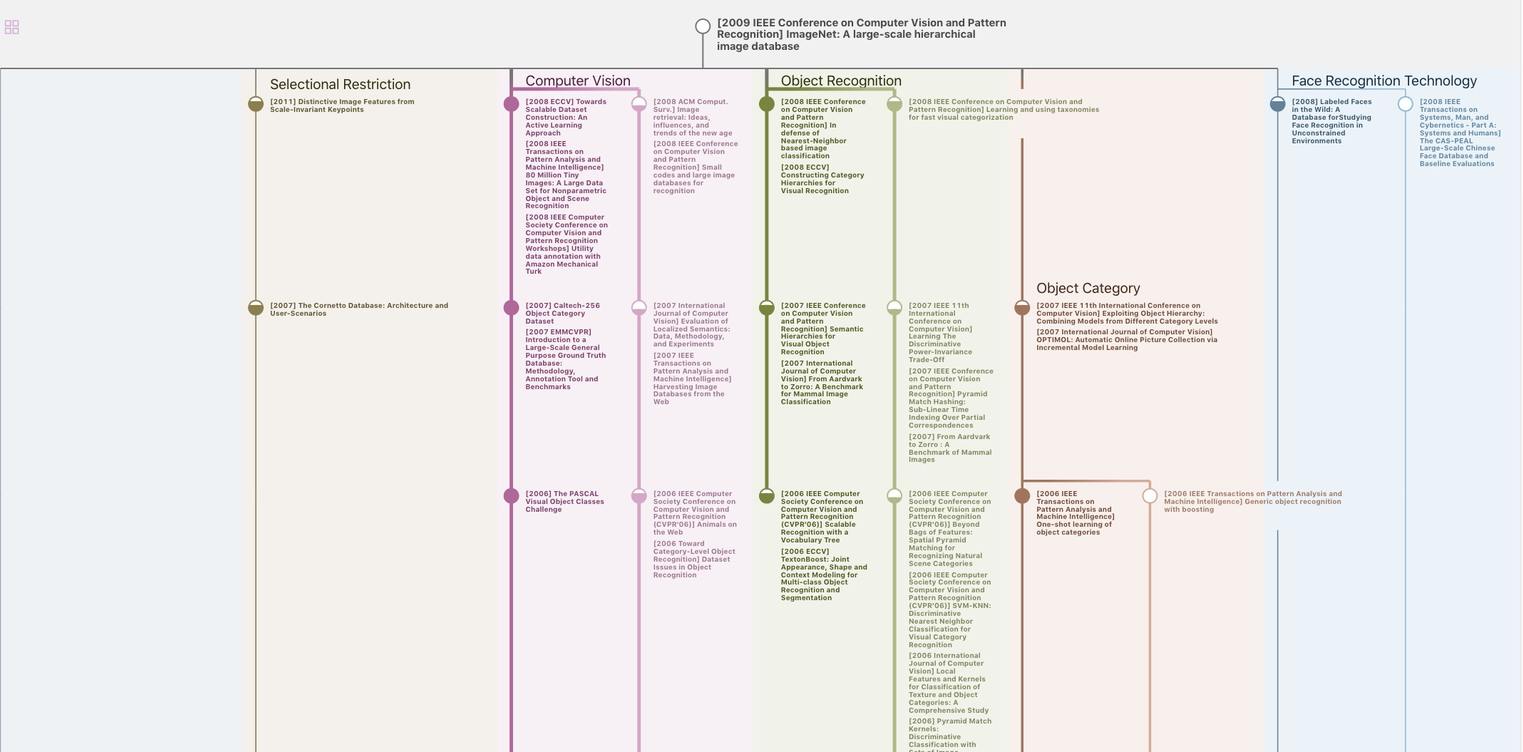
生成溯源树,研究论文发展脉络
Chat Paper
正在生成论文摘要