Rethinking and Simplifying Bootstrapped Graph Latents
PROCEEDINGS OF THE 17TH ACM INTERNATIONAL CONFERENCE ON WEB SEARCH AND DATA MINING, WSDM 2024(2024)
摘要
Graph contrastive learning (GCL) has emerged as a representative paradigm in graph self-supervised learning, where negative samples are commonly regarded as the key to preventing model collapse and producing distinguishable representations. Recent studies have shown that GCL without negative samples can achieve stateof-the-art performance as well as scalability improvement, with bootstrapped graph latent (BGRL) as a prominent step forward. However, BGRL relies on a complex architecture to maintain the ability to scatter representations, and the underlying mechanisms enabling the success remain largely unexplored. In this paper, we introduce an instance-level decorrelation perspective to tackle the aforementioned issue and leverage it as a springboard to reveal the potential unnecessary model complexity within BGRL. Based on our findings, we present SGCL, a simple yet effective GCL framework that utilizes the outputs from two consecutive iterations as positive pairs, eliminating the negative samples. SGCL only requires a single graph augmentation and a single graph encoder without additional parameters. Extensive experiments conducted on various graph benchmarks demonstrate that SGCL can achieve competitive performance with fewer parameters, lower time and space costs, and significant convergence speedup.
更多查看译文
关键词
Graph Neural Networks,Graph Representation Learning,Graph Self-supervised Learning
AI 理解论文
溯源树
样例
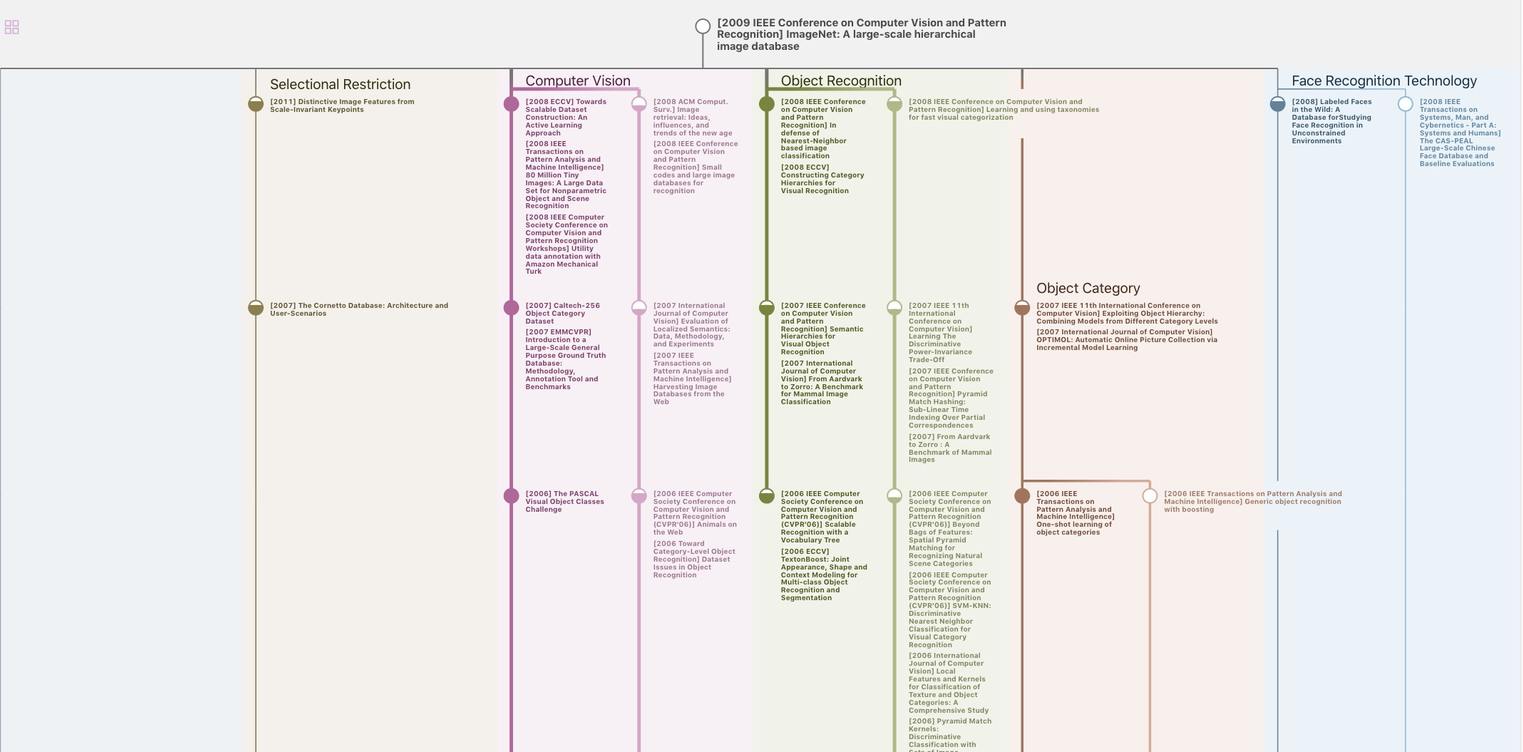
生成溯源树,研究论文发展脉络
Chat Paper
正在生成论文摘要