Correlation and Unintended Biases on Univariate and Multivariate Decision Trees
CoRR(2023)
摘要
Decision Trees are accessible, interpretable, and well-performing classification models. A plethora of variants with increasing expressiveness has been proposed in the last forty years. We contrast the two families of univariate DTs, whose split functions partition data through axis-parallel hyperplanes, and multivariate DTs, whose splits instead partition data through oblique hyperplanes. The latter include the former, hence multivariate DTs are in principle more powerful. Surprisingly enough, however, univariate DTs consistently show comparable performances in the literature. We analyze the reasons behind this, both with synthetic and real-world benchmark datasets. Our research questions test whether the pre-processing phase of removing correlation among features in datasets has an impact on the relative performances of univariate vs multivariate DTs. We find that existing benchmark datasets are likely biased towards favoring univariate DTs.
更多查看译文
关键词
Decision Tree,Benchmark Datasets,Hyperplane,Dataset Characteristics,Real-world Datasets,Performance Literature,Split Function,Learning Algorithms,Support Vector Machine,Optimization Algorithm,Standard Practice,Performance Metrics,Class Labels,Information Gain,Root Node,Average Precision,Correlated Features,Decision Boundary,Standard Datasets,Linear Support Vector Machine,Label Noise,Slope Angle,Feature Pairs,Standard Benchmark Datasets,Absence Of Noise,Mixed Integer Linear Programming,Child Nodes,Performance Gap
AI 理解论文
溯源树
样例
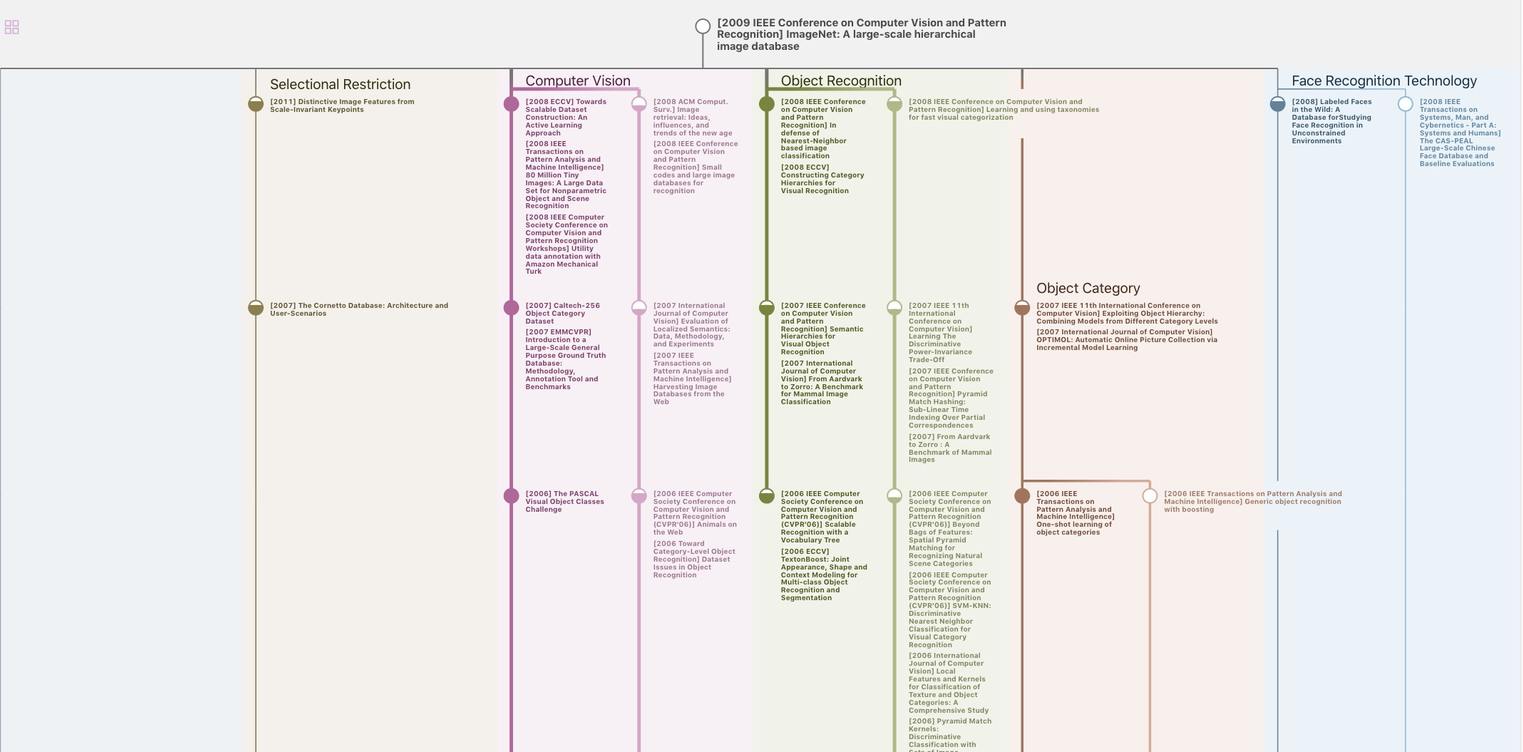
生成溯源树,研究论文发展脉络
Chat Paper
正在生成论文摘要