Joint extraction of biomedical overlapping triples through feature partition encoding
EXPERT SYSTEMS WITH APPLICATIONS(2024)
摘要
Entities and relations extraction are the key tasks in the construction of biomedical knowledge graph, which play an important role in the biomedical artificial intelligence. However, extraction of entities and relations from biomedical texts is challenging because of the overlapping triples problem. The previous approaches typically divided the task into two separate sub-tasks. However, these methods failed to address the error propagation problem. Recent methods have been proposed to perform both sub-tasks simultaneously. Nonetheless, most current methods still encounter issues related to imbalanced interactions and independent features. In this paper, we propose a novel method based on feature partition encoding and relative positional embedding to joint extract biomedical entity and relation triples simultaneously. Compared to previous work, our method shows exceptional accurate in extracting entities and relations, while efficiently tackling the challenge of overlapping triples in biomedical texts. Our work has two contributions. Firstly, our method divides the features into task-specific and shared parts through entity, relation and sharing partitions at the encoding stage. And the encoded features will be aggregated according to the subsequent tasks. Secondly, we introduce a relative positional embedding method to capture the relative distance information between token pairs. In this way, our method can effectively deal with the sub-tasks interactions problem and improve entities and relations extraction. The experimental results show that our method improves the F1 scores of relations extraction by 3.2%, 2.1%, 3.4%, and 2.8% on four biomedical datasets, respectively.
更多查看译文
关键词
Entities and relations extraction,The overlapping triple problem,Feature partition encoding,Relative positional embedding
AI 理解论文
溯源树
样例
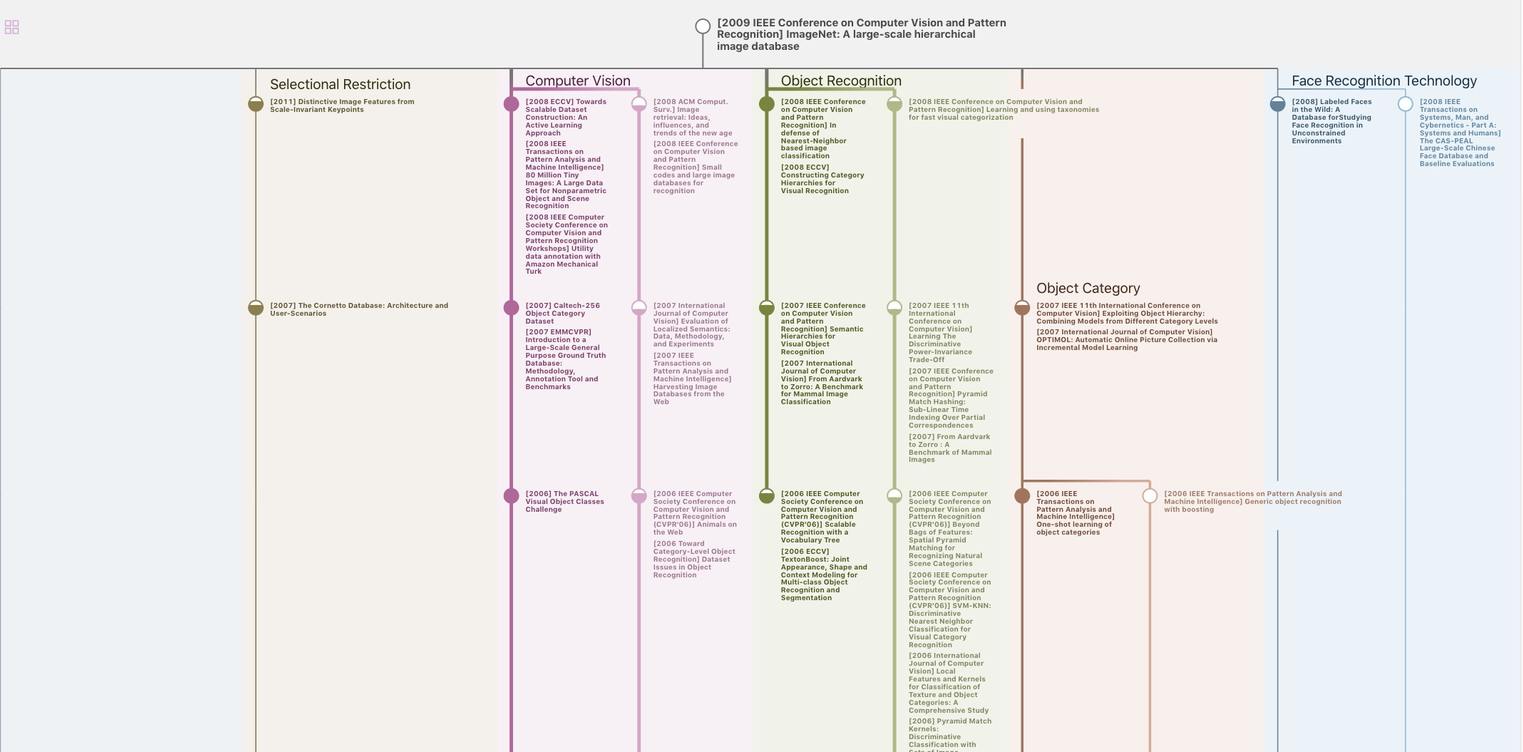
生成溯源树,研究论文发展脉络
Chat Paper
正在生成论文摘要