An artificial neural network-based machine learning approach to correct coarse-mesh-induced error in computational fluid dynamics modeling of cell culture bioreactor
FOOD AND BIOPRODUCTS PROCESSING(2024)
摘要
Computational Fluid Dynamics (CFD) is a valuable tool for studying fluid environments within cell culture bioreactors and optimizing processing parameters, but it can be computationally expensive. This study developed an artificial neural network (ANN)-based machine learning model to predict and correct the coarse-meshinduced errors in CFD modeling of a spinner flask bioreactor. A baseline ANN model was trained to predict the velocity error function between the coarse and optimized reference mesh results at one rotational speed (90 rpm), demonstrating that the ANN-based approach could correct the coarse-mesh velocity with RMSE values of nodal velocities improved by an average of similar to 20% at different rotational speeds. The effect of ANN structure, input data normalization, and training dataset combinations on prediction performance was evaluated. More neurons and hidden layers generated better results but required more computational time for training. The model's generalization capabilities were further evaluated in case studies of correcting velocity and Kolmogorov length at different fluid viscosity and bioreactor impeller geometry conditions. Results suggested that the ANN model had better generalization in correcting Kolmogorov length than velocity. This research provides insights into using a machine learning approach to enhance CFD modeling in bioreactor applications, contributing to advancing tissue engineering processes.
更多查看译文
关键词
Machine learning,ANN,CFD,Coarse mesh error,Spinner flask,Cell culture
AI 理解论文
溯源树
样例
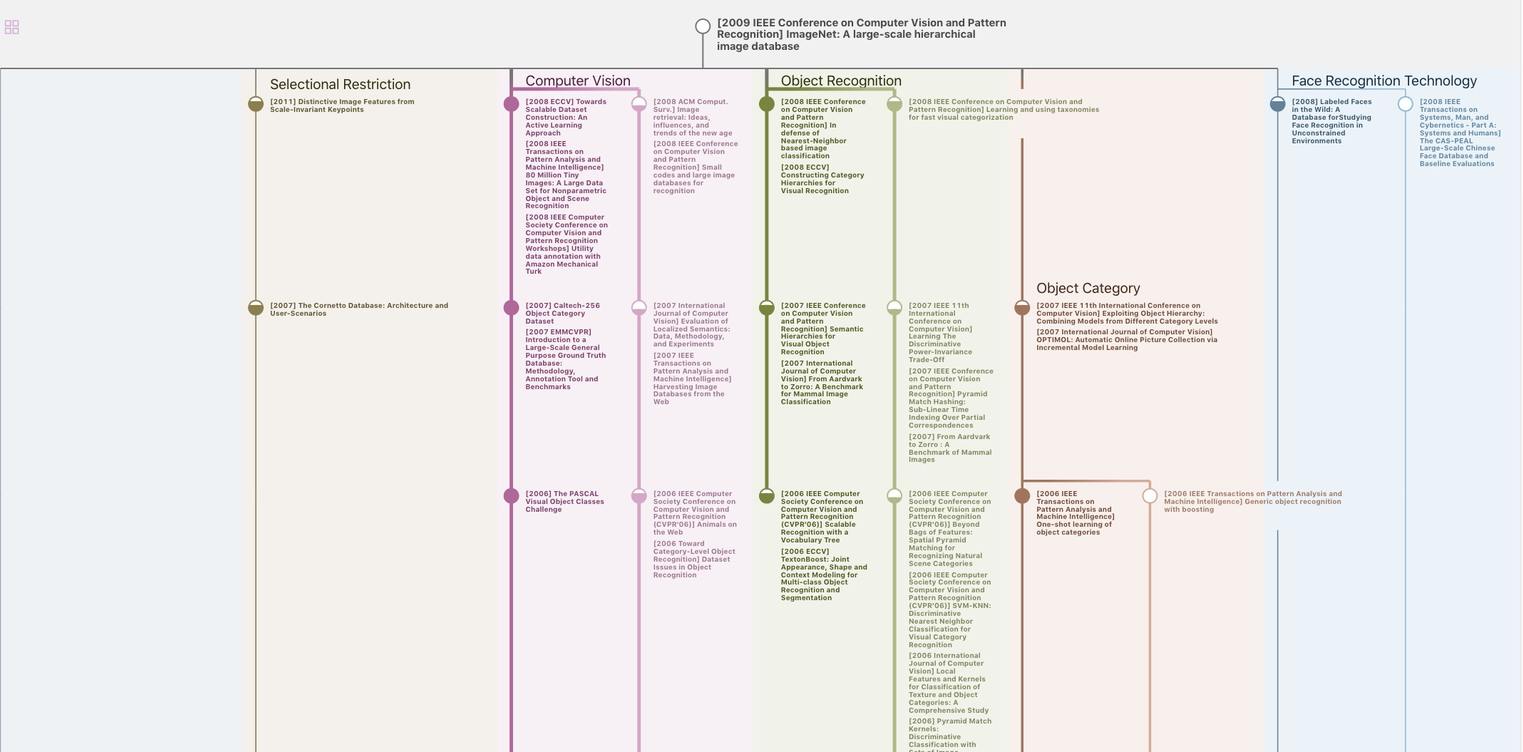
生成溯源树,研究论文发展脉络
Chat Paper
正在生成论文摘要