Progressive reinforcement learning for video summarization
INFORMATION SCIENCES(2024)
摘要
Video summarization addresses generating video summaries to help watchers grasp the content of a video without watching it entirely. Many methods have engaged in automatic video summarization. Although these methods have performed well, they still suffer from limited training data and sparse reward problems. We propose a Progressive Reinforcement Learning Video Summarization structure (PRLVS) with an unsupervised reward. The reward measures the information and quality the selected frames convey without annotations. Striving to earn higher rewards, our PRLVS adopts a "T"-type human thinking paradigm: choosing some key frames and checking if their adjacent frames are better than them. To simulate this paradigm, we decompose the flat strategy into a hierarchical strategy consisting of a horizontal policy and a vertical policy. These two policies are optimized alternatively, which densifies the reward while reducing the exploration space. Their cooperation also makes the agent capture the context information of the whole video at every step. Extensive experimental results on two benchmark databases (i.e., SumMe, TVSum) show that our PRLVS outperforms the comparisons and approaches the supervised methods, which indicates that it is significant to integrate our unsupervised reward into the progressive reinforcement learning structure to address limited annotation and sparse reward problems.
更多查看译文
关键词
Video summarization,Progressive reinforcement learning,Hierarchical strategy
AI 理解论文
溯源树
样例
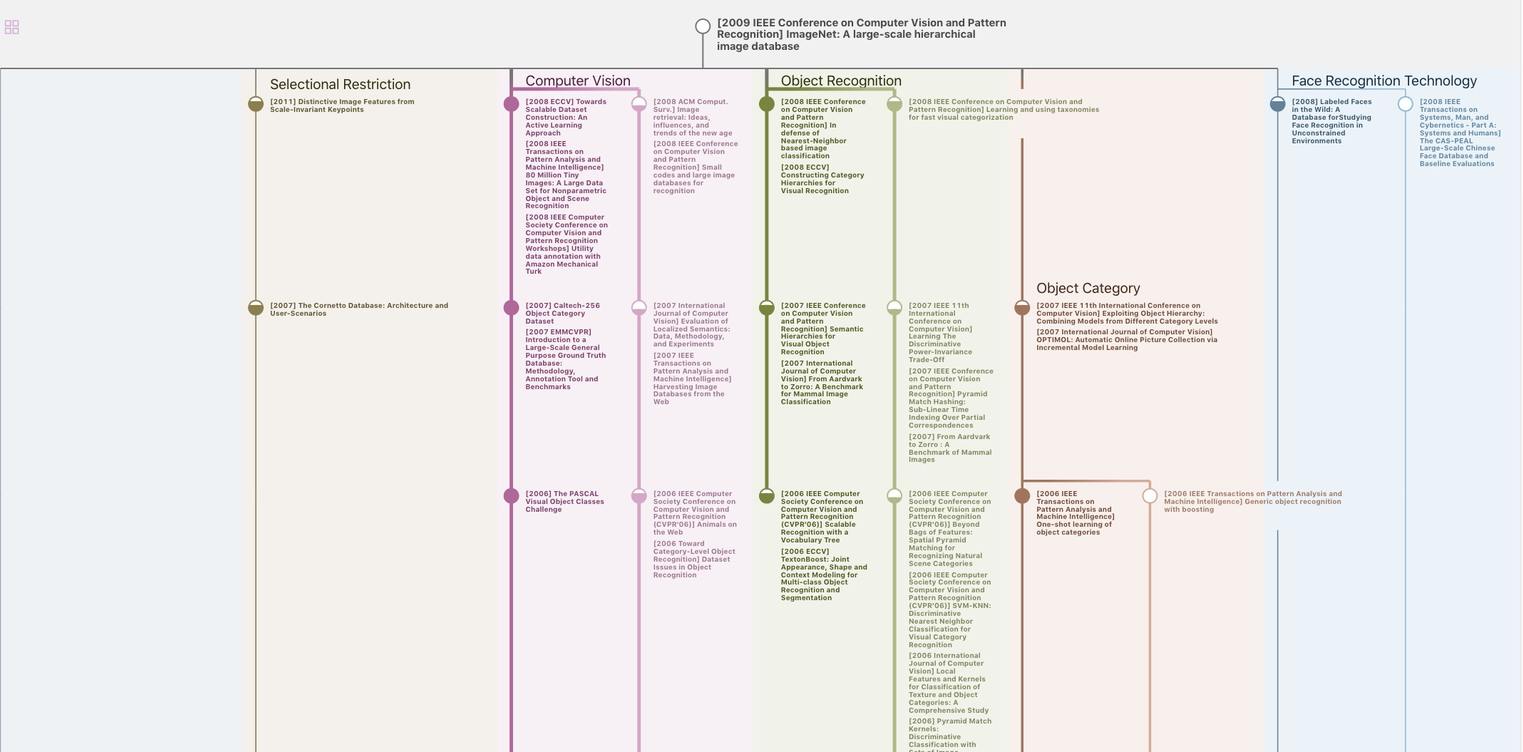
生成溯源树,研究论文发展脉络
Chat Paper
正在生成论文摘要