Safety assessment of tunnel construction based on counterintuitivity detection using multi-profile multi-model ensemble learning
EXPERT SYSTEMS WITH APPLICATIONS(2024)
摘要
Motivated to accurately predict the building tilt rate (BTR) in tunnel construction, a new multi-profile multimodel ensemble learning approach is proposed in this study by distinguishing between counterintuitive data and intuitive data, and handling each type appropriately. The proposed approach consists of three phases, namely the definition, identification, and handling of counterintuitive data, which are the major theoretical contributions of this study. Firstly, counterintuitive data is defined based on the input-output causal relation of safety-related data, introducing a novel concept in this research. Secondly, counterintuitive data is initially identified using a multi-profile ensemble learning approach and subsequently validated through multi-model ensemble learning. Finally, the identified and confirmed counterintuitive data is handled by assigning reduced weights. To validate the practicality of the approach, a case study on predicting the building tilt rate (BTR) in tunnel construction is conducted. The results of the case study demonstrate that the proposed multi-profile multi-model approach yields more accurate predictions of BTR compared to direct prediction and several other machine learning approaches. Furthermore, the proposed approach aids in identifying counterintuitive data in the testing dataset. Additionally, the effectiveness and superiority of the proposed approach are verified by comparing the prediction results when traditional abnormal data or random data is identified and treated as counterintuitive data.
更多查看译文
关键词
Tunnel construction,Safety assessment,Counterintuitive data,Multi-profile ensemble learning,Multi -model ensemble learning
AI 理解论文
溯源树
样例
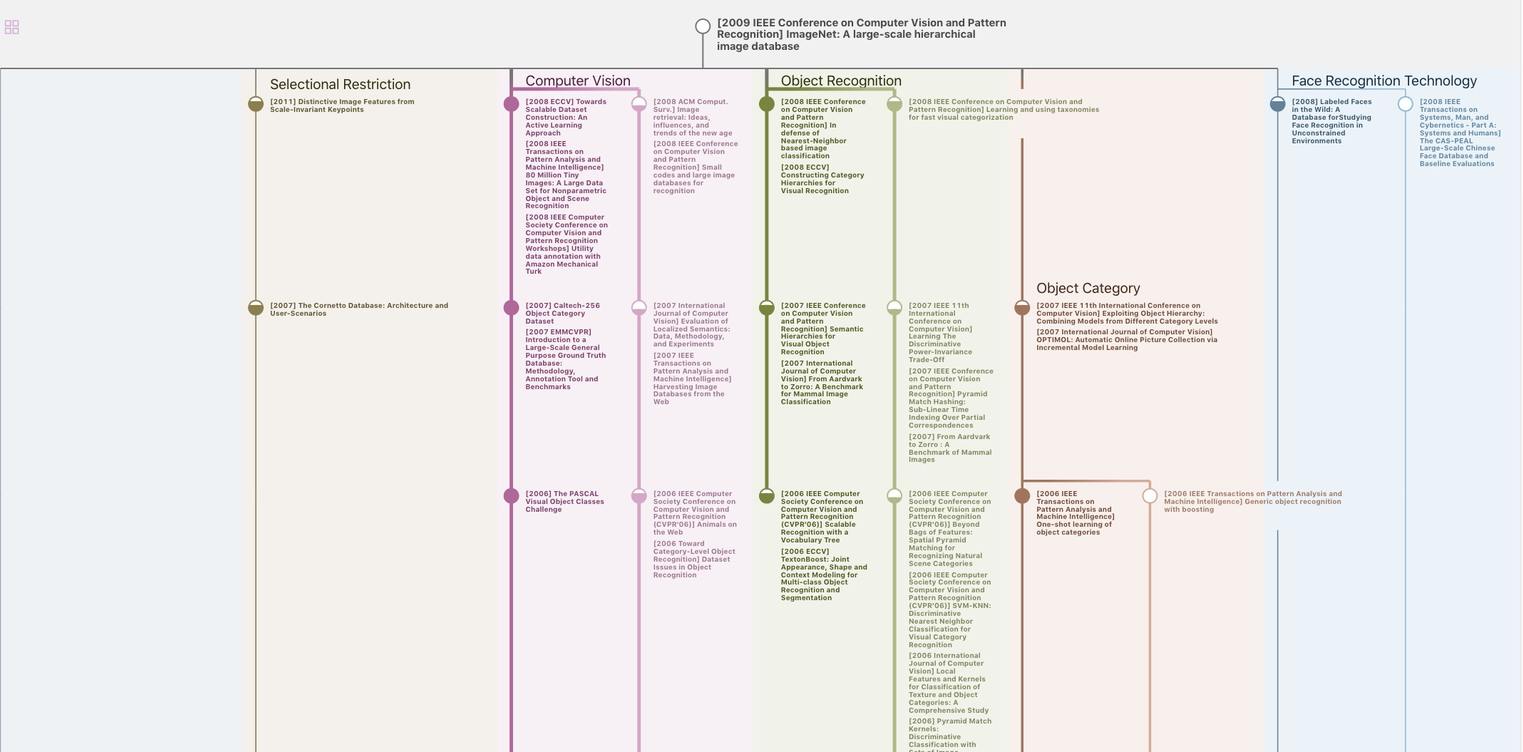
生成溯源树,研究论文发展脉络
Chat Paper
正在生成论文摘要