SPaRKLE : Symbolic caPtuRing of knowledge for Knowledge graph enrichment with LEarning
K-CAP(2023)
摘要
Knowledge graphs (KGs) naturally capture the convergence of data and knowledge, making them expressive frameworks for describing and integrating heterogeneous data in a coherent and interconnected manner. However, based on the Open World Assumption (OWA), the absence of information within KGs does not indicate falsity or non-existence; it merely reflects incompleteness. Inductive learning over KGs involves predicting new relationships based on existing statements in the KG, using either numerical or symbolic learning models. The Partial Completeness Assumption (PCA) heuristic efficiently guides inductive learning methods for Link Prediction (LP) by refining predictions about absent KG relationships. Nevertheless, numeric techniques– like KG embedding models– alone may fall short in accurately predicting missing information, particularly when it comes to capturing implicit knowledge and complex relationships. We propose a hybrid method named SPaRKLE that seamlessly integrates symbolic and numerical techniques, leveraging the PCA heuristic to capture implicit knowledge and enrich KGs. We empirically compare SPaRKLE with state-of-the-art KG embedding and symbolic models, using established benchmarks. Our experimental outcomes underscore the efficacy of this hybrid approach, as it harnesses the strengths of both paradigms. SPaRKLE is publicly available on GitHub1.
更多查看译文
AI 理解论文
溯源树
样例
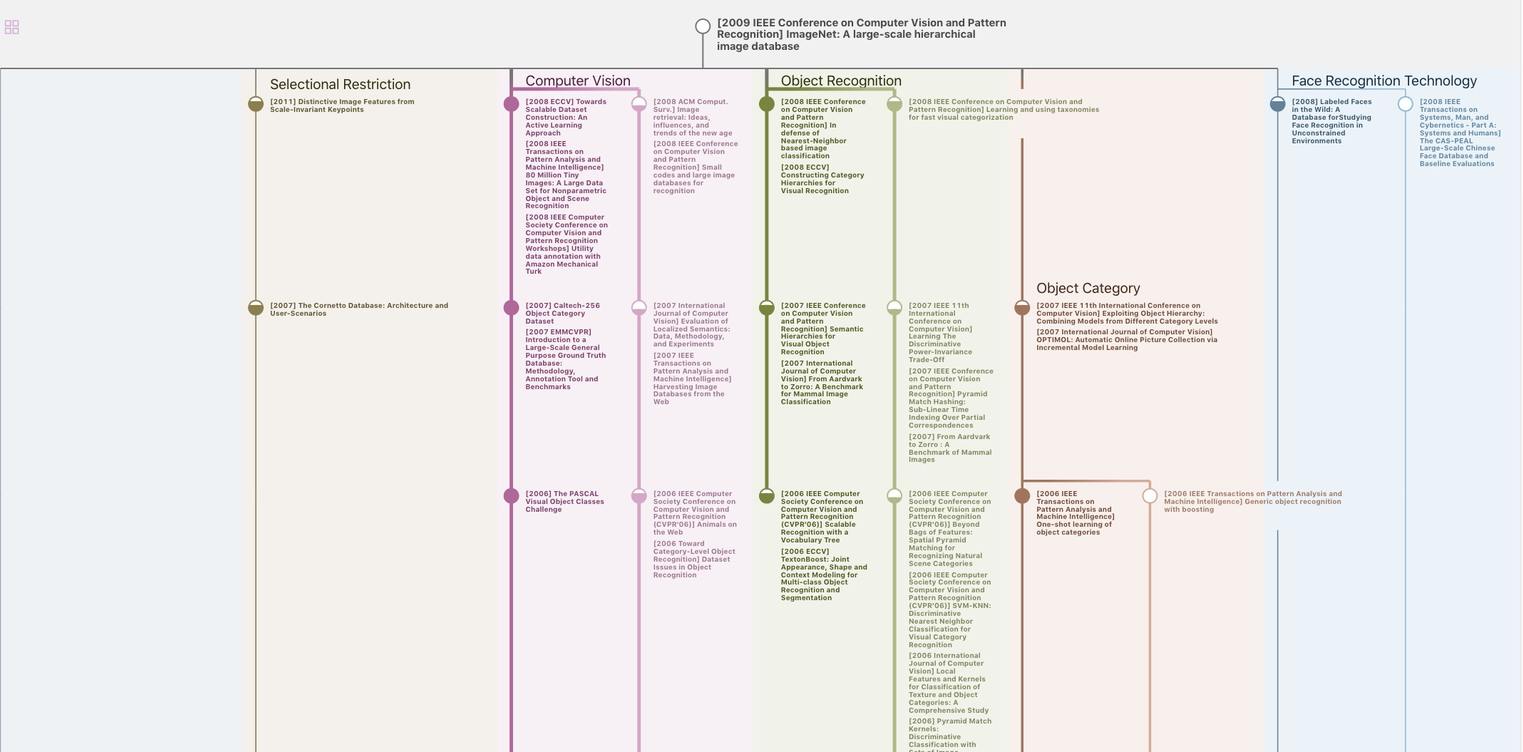
生成溯源树,研究论文发展脉络
Chat Paper
正在生成论文摘要